Overlap in high dimensional observational studies preliminary draft do not cite
semanticscholar(2017)
摘要
A key advantage of observational studies with high-dimensional covariates is that the unconfoundedness assumption is often more plausible than in low-dimensional settings. Less discussed is the fact that overlap in covariate distributions (a.k.a., positivity or common support) in the population becomes less plausible with high-dimensional covariates. We show that the overlap assumption in high dimensions is stronger than most investigators realize. In particular, overlap implies bounds on the information in the covariates that discriminates between the covariate distributions in the treated and control populations. These bounds more restrictive the higher the covariate dimension. Under some distributional assumptions, this implies an explicit constraint on the imbalance in covariate means, and in many cases, this bound converges to zero as the dimension grows large. These results are particularly relevant to regular semiparametric estimators of the average treatment effect (ATE), which have recently been adapted for high-dimensional settings, and which rely heavily on the overlap assumption. Given the strength of the overlap assumption in high dimensions, we suggest that (i) tests that can used to validate the overlap assumption and (ii) covariate reduction techniques that can weaken the overlap assumption should be developed alongside high-dimensional estimation methods.
更多查看译文
AI 理解论文
溯源树
样例
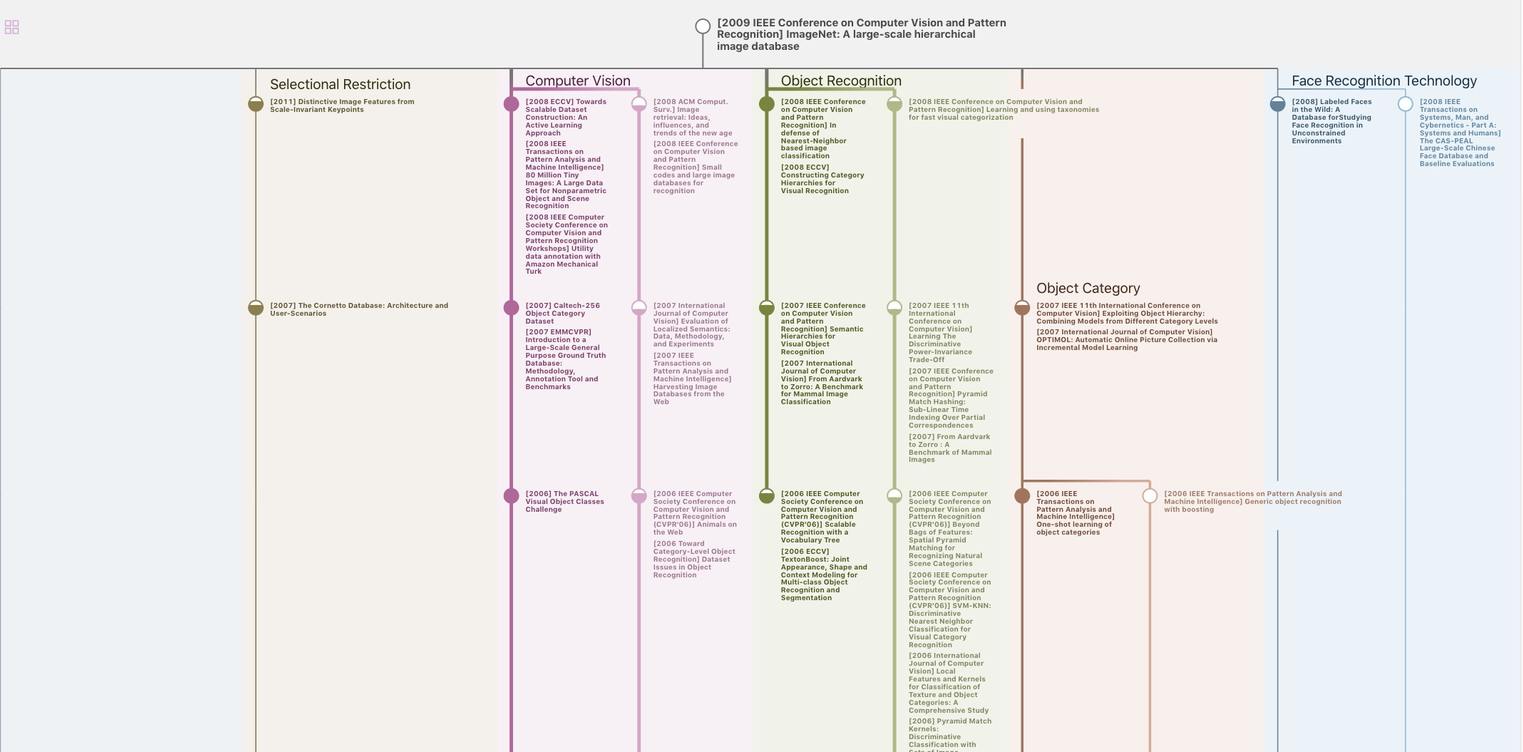
生成溯源树,研究论文发展脉络
Chat Paper
正在生成论文摘要