Editorial
Computer Methods in Biomechanics and Biomedical Engineering: Imaging & Visualization(2019)
摘要
Deep learning methods have experienced an immense growth in interest from the medical image analysis community, particularly in the last years. The main reasons behind this interest lie in ability of deep learning algorithms to process very large training sets, to transfer learned features between different databases, and to analyse multimodal data. These advantages are providing important opportunities for the development of medical image analysis methodologies, such as computer-aided diagnosis, image segmentation, image annotation and retrieval, image registration and multimodal image analysis. Deep Learning in Medical Image Analysis (DLMIA) is a workshop dedicated to the presentation of works focused on the design and use of deep learningmethods in medical image analysis applications. This workshop is setting the trends and identifying the challenges of the use of deep learning methods in medical image analysis. Another important objective of the workshop is to continue and increase the connection between software developers, specialist researchers and applied end-users from diverse fields related to Medical Image and Signal Processing, which are the main scopes of the International Conference On Medical Image Computing & Computer Assisted Intervention (MICCAI). The 2 MICCAI workshop on Deep Learning in Medical Image Analysis (DLMIA 2016) was organized under the auspicious of the 19th International Conference on Medical Image Computing and Computer Assisted Intervention (MICCAI 2016), which was held from October 17th to 21st, 2016, in Athens, Greece, and the 3 MICCAI workshop on Deep Learning in Medical Image Analysis (DLMIA 2017) was organized under the auspicious of the 20th International Conference on Medical Image Computing and Computer Assisted Intervention (MICCAI 2017), which was held from September 10th to 14th, in Quebec City, Quebec, Canada. This special issue of the journal Computer Methods in Biomechanics and Biomedical Engineering: Imaging & Visualization contains the extended and revised manuscripts of 2 contributions presented at DLMIA 2016 and of 2 contributions presented at DLMIA 2017, which were reviewed according to the journal policy. The included manuscripts present and discuss methods, techniques, challenges and new trends related to Deep Learning in Medical Imaging Analysis: 1) Yoo et al. studied whether deep learning can extract latent multiple sclerosis (MS) lesion features from magnetic resonance imaging (MRI) scans that, when combined with user-defined radiological and clinical measurements, can predict conversion toMS, in patients with early MS symptoms, a prodromal stage of MS, more accurately than imaging biomarkers that have been used in clinical studies to evaluate overall disease state, such as lesion volume andbrain volume; 2) AkselrodBallin et al. proposedanovel system for detection andclassification of masses in breast mammography, which integrates a breast segmentation module together with a modified region-based convolutional network to obtain detection and classification of masses according to Breast Imaging Reporting and Data System (BI-RADS) score; 3) Akbar et al. suggested a modified ‘transition’ module, which includes filters of varying sizes are used to encourage class-specific filters at multiple spatial resolutions followed by global average pooling, in order to encourage generalisation in a deep learning frameworkwith few training samples, and tested it in classifying breast tumours in two data-sets of scanned histology sections; and 4) Yuexiang and Chen proposed a deep-learning network, namely HEp-Net, to automatically classify Human Epithelial-2 (HEp-2) cell images. The Guest-Editors wish to thank all the DLMIA 2016 & 2017 Authors, particularly the authors of the four articles included in this special issue, and themembers of the Program Committee for sharing their expertise, The MICCAI Society for having hosted and supported theworkshopwithinMICCAI 2016&2017 and also to the CMBBE: Imaging & Visualization Editors and Reviewers for helping improving the manuscripts accepted.
更多查看译文
AI 理解论文
溯源树
样例
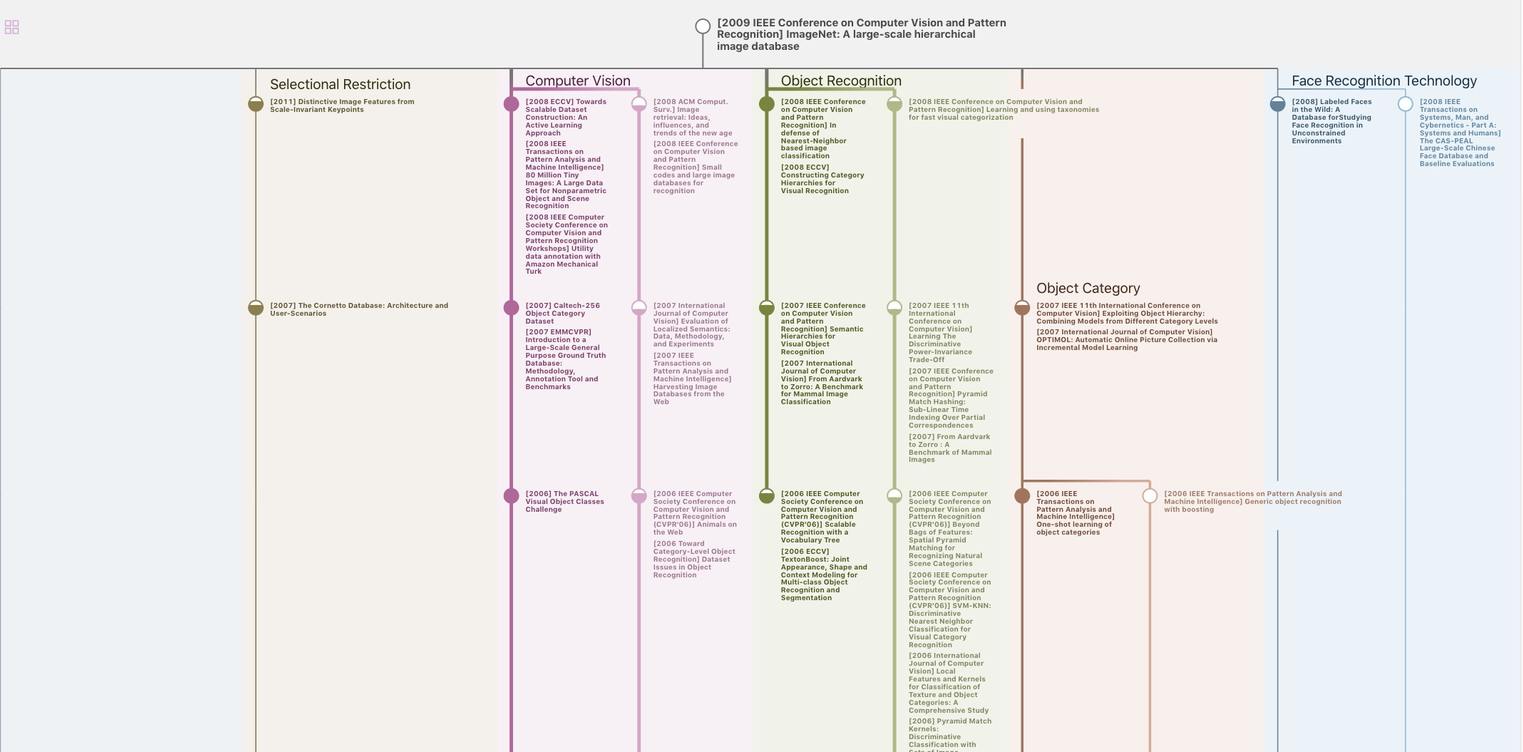
生成溯源树,研究论文发展脉络
Chat Paper
正在生成论文摘要