Borderline Oversampling in Feature Space for Learning Algorithms in Imbalanced Data Environments
semanticscholar(2016)
摘要
In this paper, we propose a new approach to over-sample new minority-class instances along the borderline using the Euclidean distance in the feature space to improve support vector machine (SVM) performance in imbalanced data environments. SVM has been an outstandingly successful classifier in a wide variety of applications where balanced class data distribution is assumed. However, SVM is ineffective when coping with imbalanced datasets whereby the majorityclass instances far outnumber the minority-class instances. Our new approach, called Borderline Over-sampling in the Feature Space, can deal with imbalanced data to effectively recognize new minority-class instances for better classification with SVM. The results of our class prediction experiments using the proposed approach demonstrate better performance than the existing SMOTE, Borderline-SMOTE and borderline over-sampling methods in terms of the g-mean and F-measure.
更多查看译文
AI 理解论文
溯源树
样例
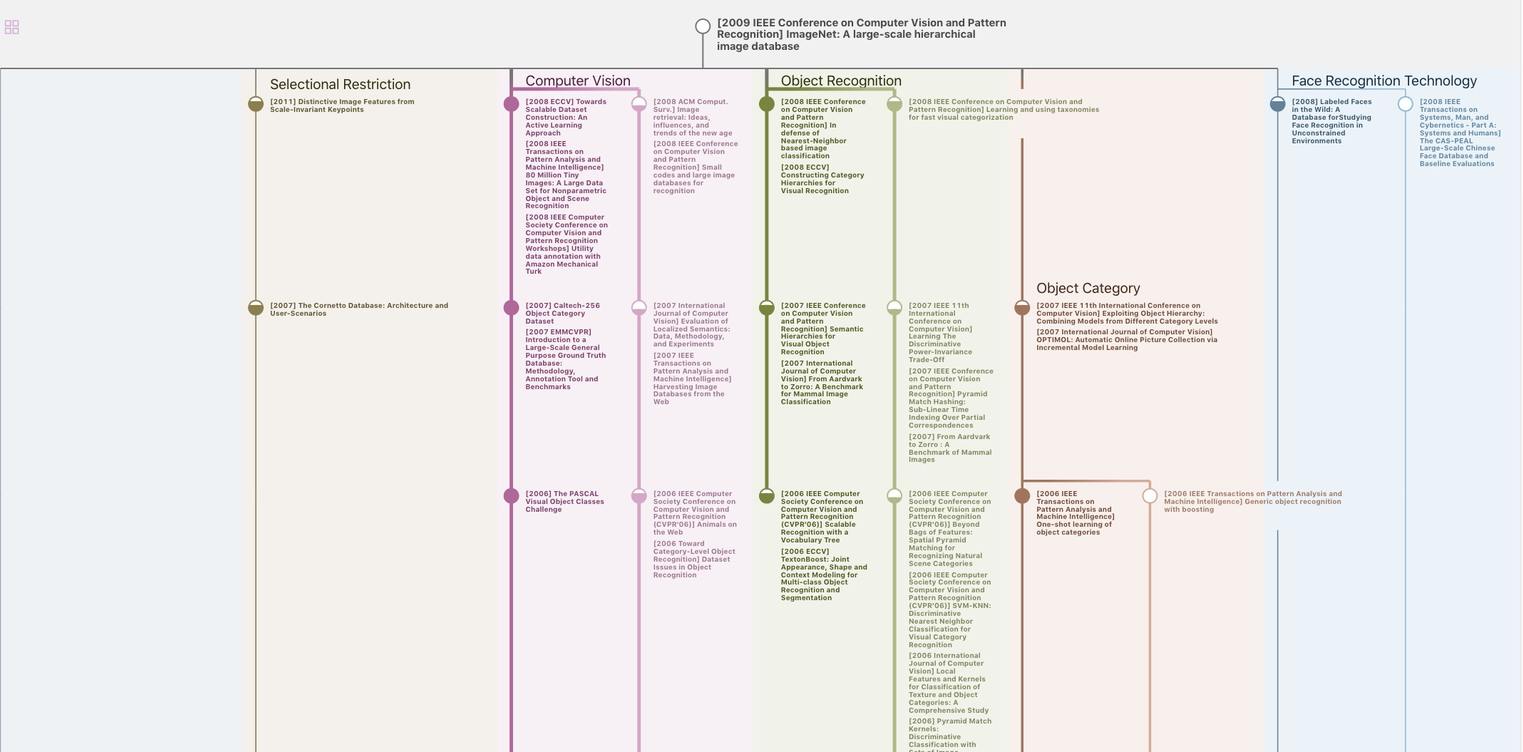
生成溯源树,研究论文发展脉络
Chat Paper
正在生成论文摘要