Scalable optimization methods for Distributed Machine Learning
semanticscholar(2015)
摘要
As data grows, there is a need to develop scalable, efficient and most importantly distributed machine learning algorithms. Distributed algorithms fall into two main categories (a) horizontal partitioning, where the data is distributed across multiple slaves. The main drawback of this strategy is that the model parameters need to be replicated on every machine. This is problematic when the number of classes, and consequently the number of parameters is very large, and hence cannot fit in a single machine. The other strategy is (b) vertical partitioning, where the model parameters are partitioned. However, here the data needs to be replicated on each machine, thus failing to scale to massive datasets.
更多查看译文
AI 理解论文
溯源树
样例
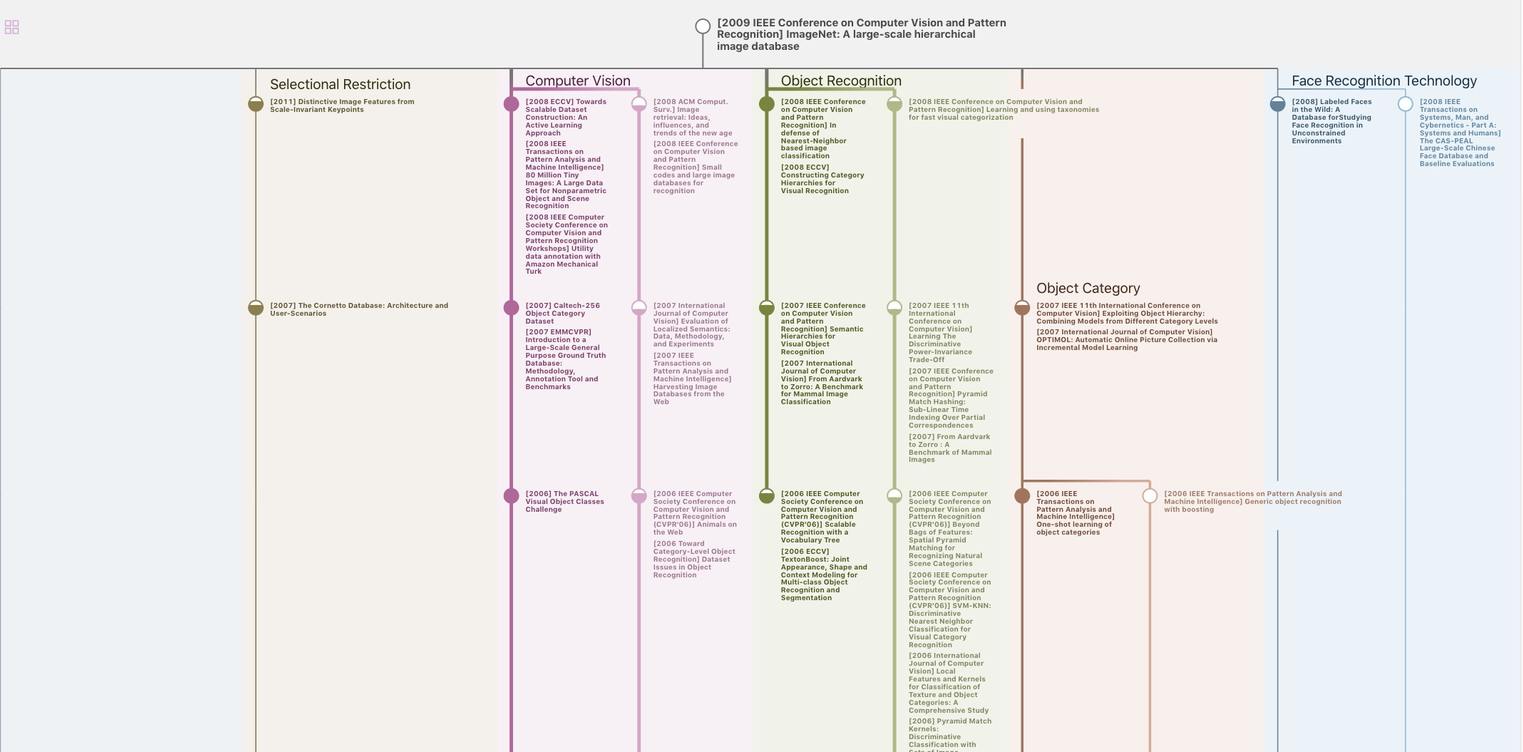
生成溯源树,研究论文发展脉络
Chat Paper
正在生成论文摘要