Machine Learning and Knowledge Discovery in Databases
Lecture Notes in Computer Science(2018)
摘要
How does one build a production ML system that can effectively incorporate corrections, while avoiding the typical risks and engineering costs of online learning methods? An effective solution requires a combination of very latest and some well-dated algorithms. Parametric machine learning methods such as neural networks, boosted trees, factorization methods and their ensembles yield state-of-the-art results on ML benchmarks and competitions. Real-world deployments of ML systems, however, differ dramatically from those static settings. We discuss issues that differentiate production and academic ML systems, leading to the need for combining parametric models with their non-parametric brethren, i.e, modern variants of Nearest Neighbor algorithms. The combined approach is particularly suitable for systems where incorporating corrections must be accomplished rapidly, as illustrated by some lively real-life examples from a large-scale conversational assistant. Bio: Misha Bilenko heads the Machine Intelligence and Research (MIR) division at Yandex, which integrates research and product development in core AI areas: machine learning, dialog systems, speech recognition and synthesis, machine translation and computer vision. Before Yandex, he led the Machine Learning Algorithms team at Microsoft, which shipped ML technologies in multiple products across all Microsoft divisions. He started his career in the Machine Learning Group in Microsoft Research after receiving his Ph.D. in Computer Science from the University of Texas at Austin and stints at Google and IBM Research. Combatting Misinformation and Building Trust in the Media
更多查看译文
AI 理解论文
溯源树
样例
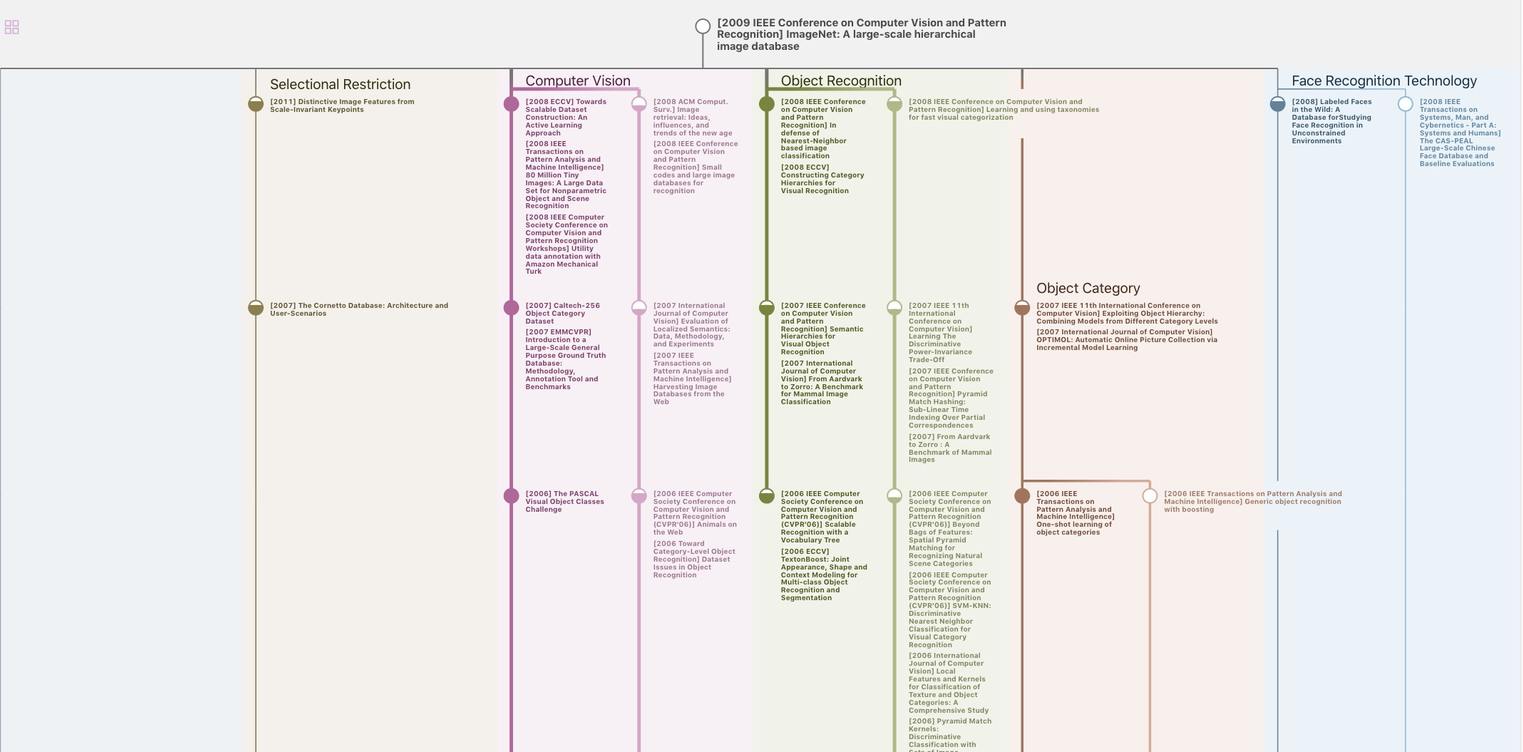
生成溯源树,研究论文发展脉络
Chat Paper
正在生成论文摘要