Unsupervised Named Entity Resolution
semanticscholar(2010)
摘要
Resolving the ambiguity of person, organisation and location names is a challenging problem in the Natural Language Processing (NLP) area. This problem is usually formulated as a clustering problem, in which the target is to group mentions of the same entity into the same cluster. In this paper, we present a different approach based on the Distributional Hypothesis and edit distance, which associates an ambiguous entity to its corresponding entry in the Wikipedia knowledge base. We experiment with two types of contextual features, i.e. bag-of-words and bigrams, as well as with another source of information, i.e. the edit distance between an entity mention and a Wikipedia article’s title. Our experiments show that the combination of these types of knowledge offers a superior performance than each one individually or any subset of them, in effect leading to the conclusion that they are able to capture non-overlapping information that is essential for this task.
更多查看译文
AI 理解论文
溯源树
样例
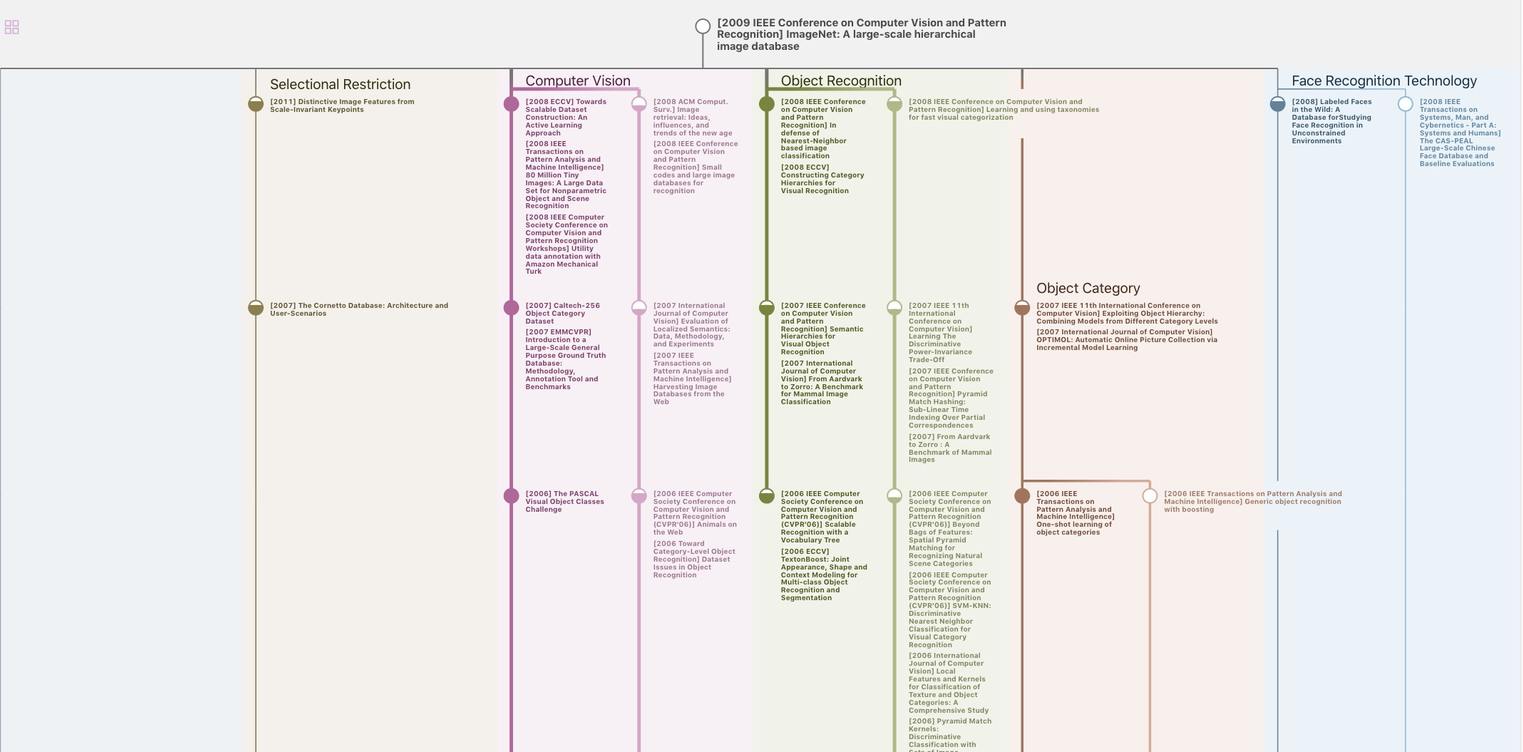
生成溯源树,研究论文发展脉络
Chat Paper
正在生成论文摘要