Automatically Improved Category Labels for Syntax-Based Statistical Machine Translation
semanticscholar(2011)
摘要
A common modeling choice in syntax-based statistical machine translation is the use of synchronous context-free grammars, or SCFGs. When training a translation model in a supervised setting, an SCFG is extracted from parallel text that has been statistically word-aligned and parsed by monolingual statistical parsers. However, the set of syntactic category labels used in a monolingual statistical parser is decided upon quite independently of the machine translation task, and there is no guarantee that it is optimal for a bilingual SCFG or for machine translation at all. In this thesis, we first demonstrate that the set of category labels used in a machine translation system’s grammar strongly affects three inter-related characteristics of the system: spurious ambiguity, rule sparsity, and reordering precision. We propose using these characteristics as the basis for evaluating the properties of an SCFG both outside of and within an actual translation task. Finally, as our main work, we propose three automatic relabeling methods that will create a better set of category labels for a given language pair and choice of automatic parsers. These methods involve clustering and collapsing unnecessary labels, splitting existing labels into multiple subtypes, and swapping specific instances of existing labels to correct for local errors. Improved properties of the grammar and improved translation results will be demonstrated for at least two language pairs.
更多查看译文
AI 理解论文
溯源树
样例
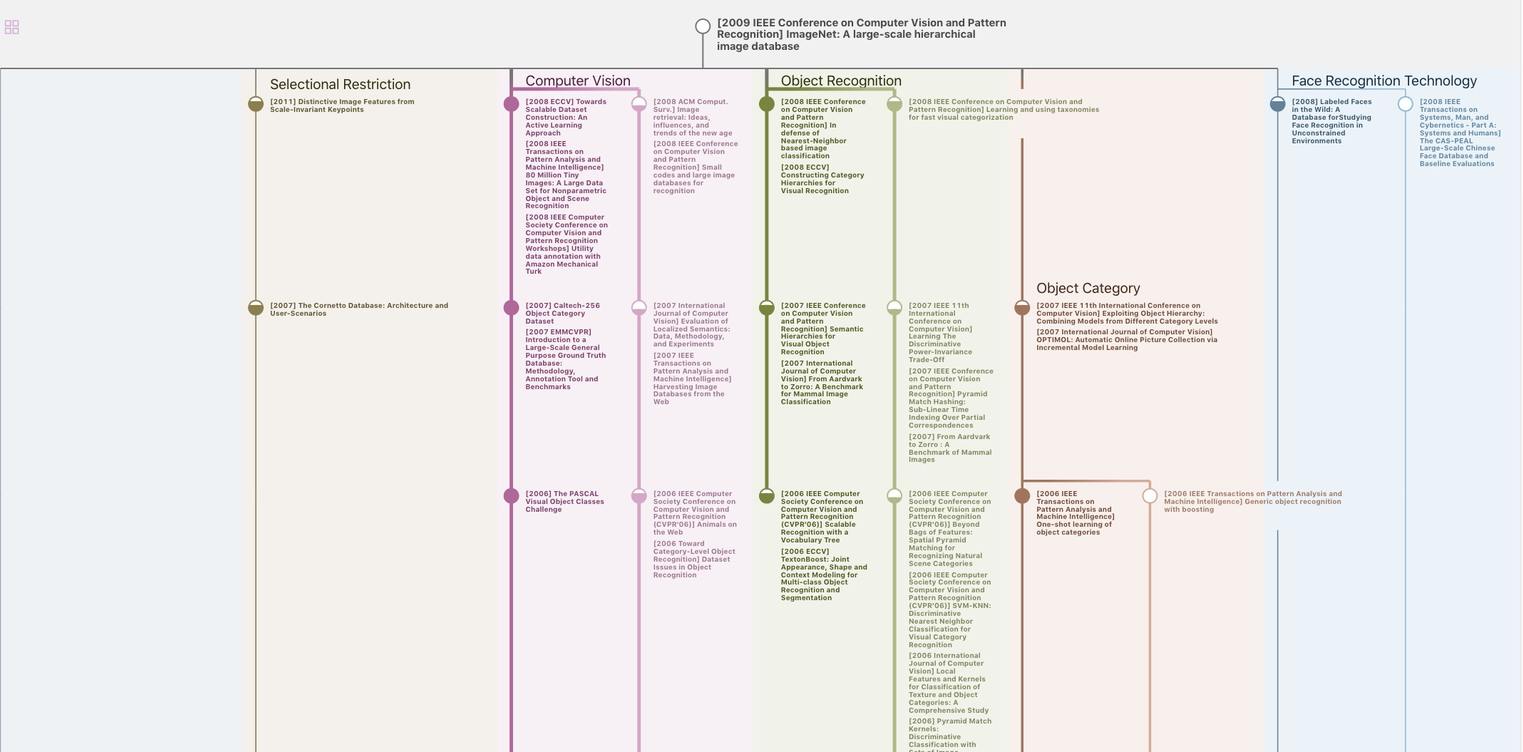
生成溯源树,研究论文发展脉络
Chat Paper
正在生成论文摘要