Unsupervised Lexical Semantic Frame Induction: A SemEval 2019 Task Proposal
semanticscholar(2019)
摘要
Frame Semantics (Fillmore, 1976) and other theories (Gamerschlag et al., 2014) that adopt typed feature structures for the representation of knowledge and linguistic structures have developed in parallel over several decades in formal linguistics studies related to the syntax– semantics interface, as well as in empirical corpus-driven applications in natural language processing (NLP). Building repositories of lexical semantic frames is a central topic in these efforts, regardless of their perspective. In formal studies, lexical semantic frame knowledge bases are built to instantiate foundational theories with tangible examples, e.g., to serve as supporting evidence for the theory. On a practical level, frame semantic repositories play a pivotal role in natural language understanding and semantic parsing (both as a source for inspiring a representation format and for training data-driven machine learning methods) to accomplish tasks such as information extraction, question answering, text summarization, machine translation, and so on. However, the manual development of lexical semantic frames databases (as well as corpus-derived annotations to support those frames) is a resource-intensive task. The most well-known publicly available Frame Semantics lexical resource is FrameNet (Ruppenhofer et al., 2016), which covers only a fraction of natural language events and concepts in a relatively small number of contextual semantic domains. While NLP research has integrated FrameNet into semantic parsing technologies (e.g. Das et al. (2014); Kshirsagar et al. (2015); Hartmann et al. (2017)), current parsing methods are not yet sufficiently effective for enriching frame repositories such as FrameNet with new frame templates, i.e., to port them to new semantic domains, and to extend them to languages other than English. In general, the same holds for supervised machine learning for the identification of frames. One way to rectify this situation is the use of unsupervised machine learning methods for identifying new semantic frame templates and populating them. Even if these unsupervised approaches are not ideal to create full-fledged frame semantic databases, employing them as an assistive lexicographical tool might well reduce the resource intensivity of the effort.1 Among the studies of unsupervised frame induction, most systems, e.g., Pennacchiotti et al. (2008) and Green et al. (2004), address the problem of domain coverage by employing other available lexical semantic resources such as WordNet (Miller, 1995), a technique that itself leads to other setbacks. Most importantly, these methods do not adapt easily across languages since for the most part these auxiliary resources are often not available in other languages.
更多查看译文
AI 理解论文
溯源树
样例
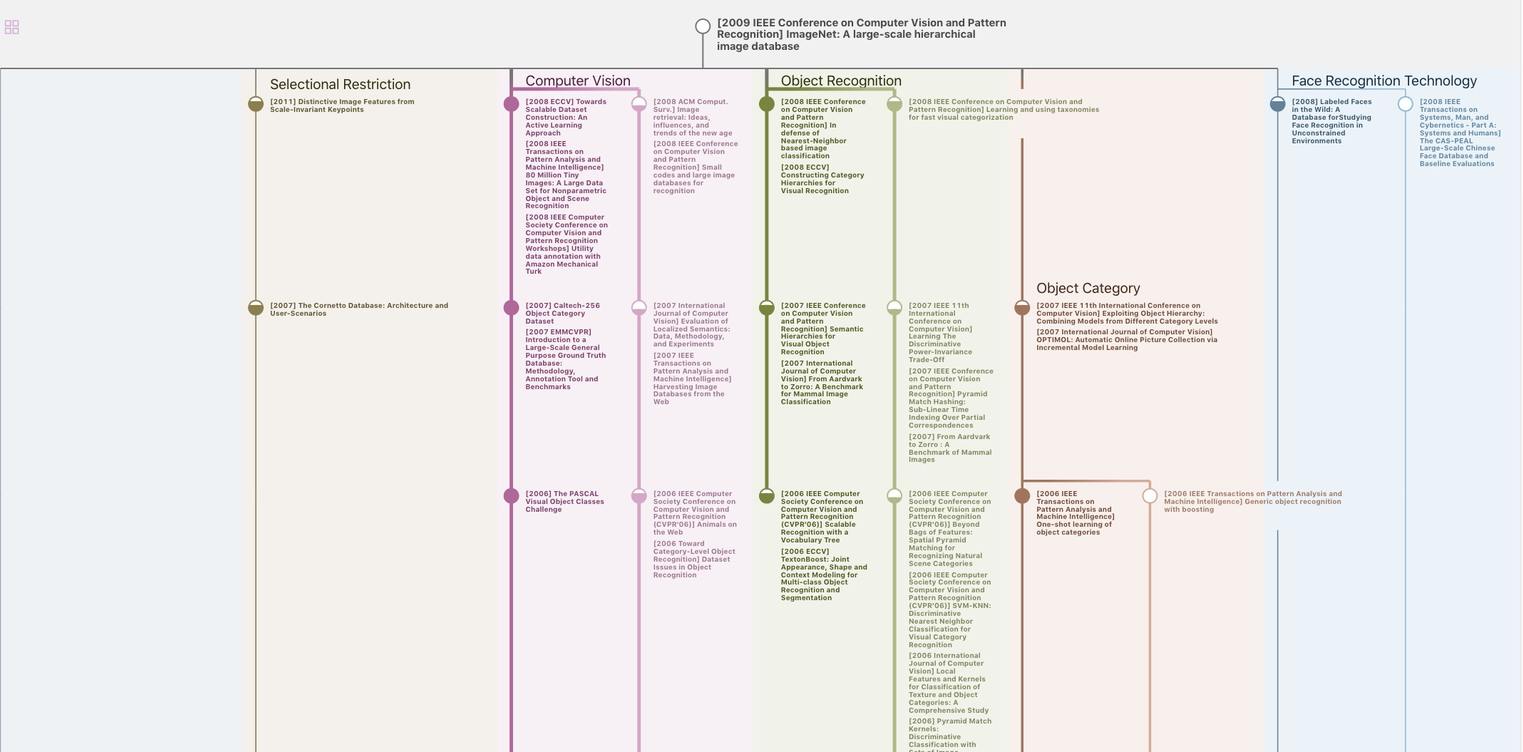
生成溯源树,研究论文发展脉络
Chat Paper
正在生成论文摘要