A machine learning ap- proach for optimisation in railway planning
semanticscholar(2018)
摘要
Planning and scheduling problem is a hard problem, especially in real life cases. The time and space complexity increase quickly along with the increase of problem size. In transportation systems, such problems exist a lot. The automation of transportation systems depends a lot on the improvements of developing planning and scheduling algorithm. Nowadays, machine learning, as modern technology, has been adopted in every field. The power of machine learning is its ability to obtain useful information from large datasets. Considering problem instance information and corresponding solution status as data and label respectively, there is the possibility that the solvable instances hold patterns in common. This is where machine learning comes into the stage. This research is focusing on how to combine machine learning with traditional planning and scheduling algorithm based on the Dutch Railway System. Specifically, making use of a large amount of stored instance and solution details to improve the service site planning process is our purpose. Combing machine learning and traditional scheduling system is a new and hard topic. In this work, we implement a machine learning system adapting to the scheduling algorithm content. We define and collect dataset matching our research goals. A framework is designed according to the imbalanced characteristics of our datasets. To explore the nature of algorithms, we choose to calculate features from the problem instances and scheduling process directly. Besides, we perform a high volume of experiments to select the based machine learning techniques to adopt. What’s more, we also design a test framework to evaluate our machine learning systems. Improvements are observed in our work. Additionally, we would like to explore the features and machine learning techniques to improve the performance in the future work.
更多查看译文
AI 理解论文
溯源树
样例
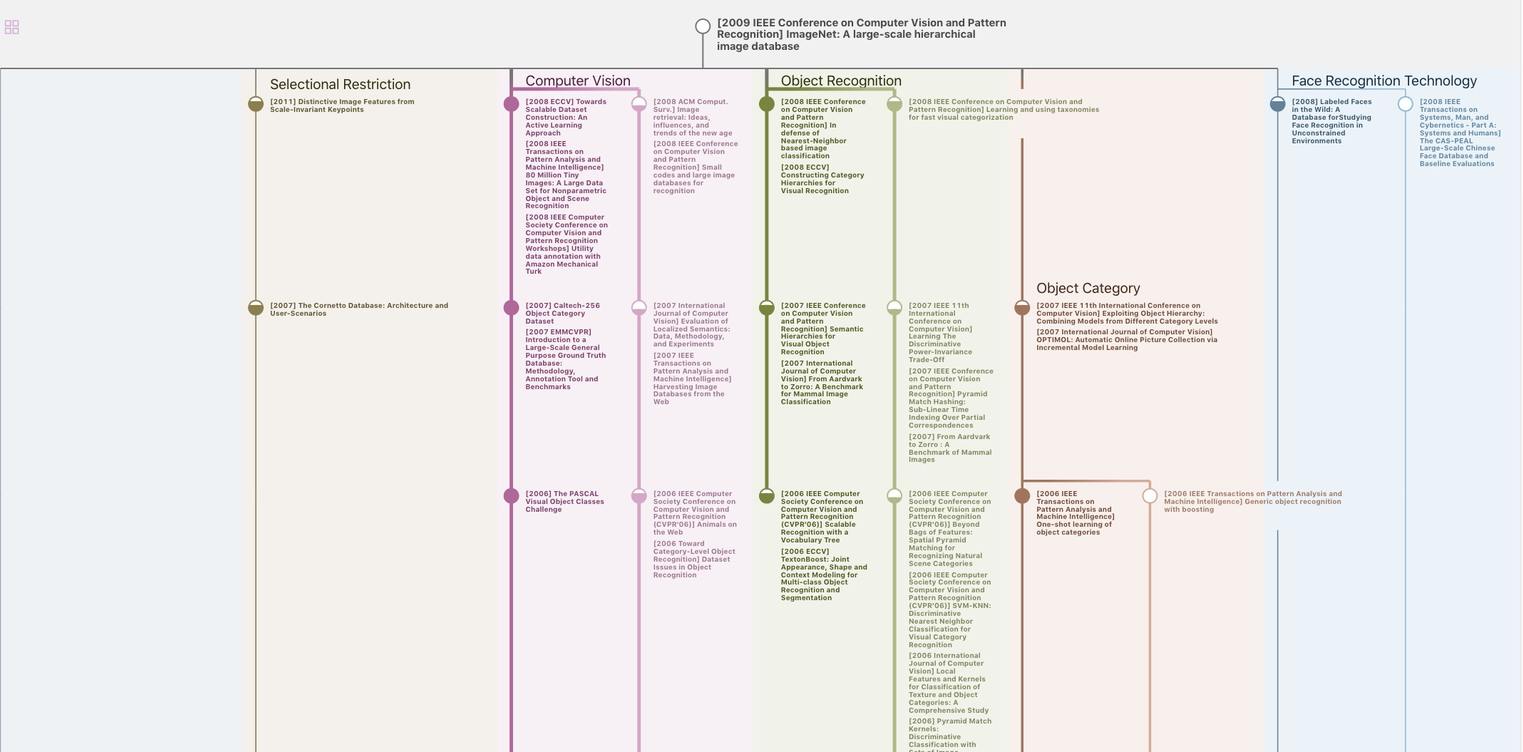
生成溯源树,研究论文发展脉络
Chat Paper
正在生成论文摘要