Nonlinear Model Predictive Control of an Aerial Manipulator using a Recurrent Neural Network Model
semanticscholar(2018)
摘要
The goal of this work is to control an aerial manipulator system which consists of an Unmanned Aerial Vehicle (UAV) platform equipped with an articulated robotic arm, through model-predictive control based on a data-driven dynamical model. The learned model captures both the internal closed loop dynamics of the UAV autopilot as well as the servo motor control logic. At the core of the model lies a Recurrent Neural Network (RNN) architecture combined with a feedforward model to produce the linear and angular accelerations applied on the robot. These accelerations are then integrated in a standard manner to obtain the next robot state. The learned RNN architecture is then leveraged in a Nonlinear Model Predictive Control (NMPC) framework that accounts for the sparsity inherent to the trajectory optimization problem. The NMPC optimization has been successfully implemented onboard a DJI Matrice UAV platform with a custom made two-link arm to track desired reference trajectories.
更多查看译文
AI 理解论文
溯源树
样例
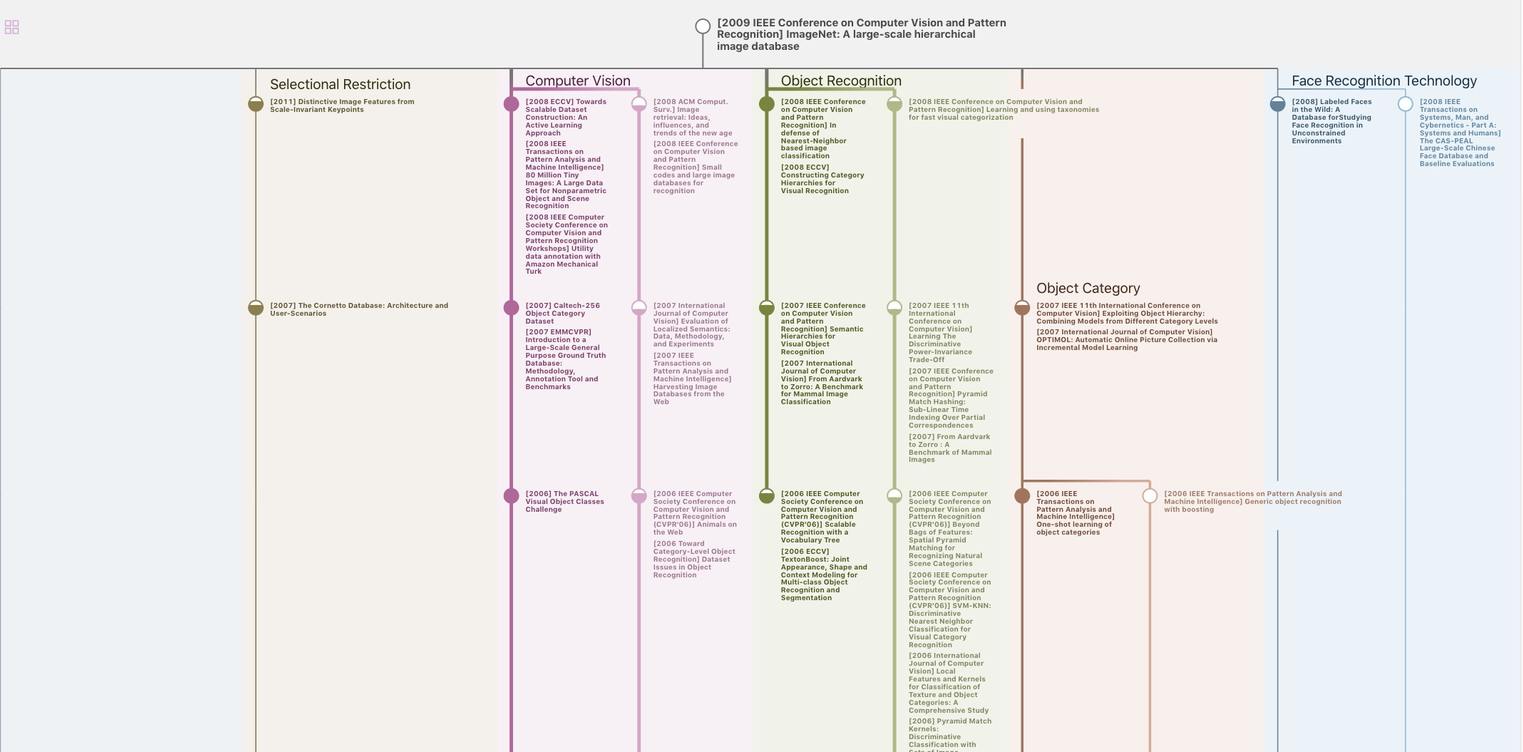
生成溯源树,研究论文发展脉络
Chat Paper
正在生成论文摘要