Adversarial Attacks on Image Recognition *
semanticscholar(2016)
摘要
This project extends the work done by Papernot et al. in [4] on adversarial attacks in image recognition. We investigated whether a reduction in feature dimensionality using principle component analysis (PCA) can maintain a comparable level of misclassification success while increasing computational efficiency. We attacked black-box image classifiers trained on the MNIST dataset by forcing the oracle to misclassify images that were modified with small perturbations. The method we used was two-fold: the target classifier was imitated with a substitute logistic regression model and then the adversarial samples were generated off of the substitute model [4]. The results show that reasonable misclassification rates with reduced computation time can be achieved for a PCA-reduced feature set utilizing the Papernot adversarial crafting algorithm.
更多查看译文
AI 理解论文
溯源树
样例
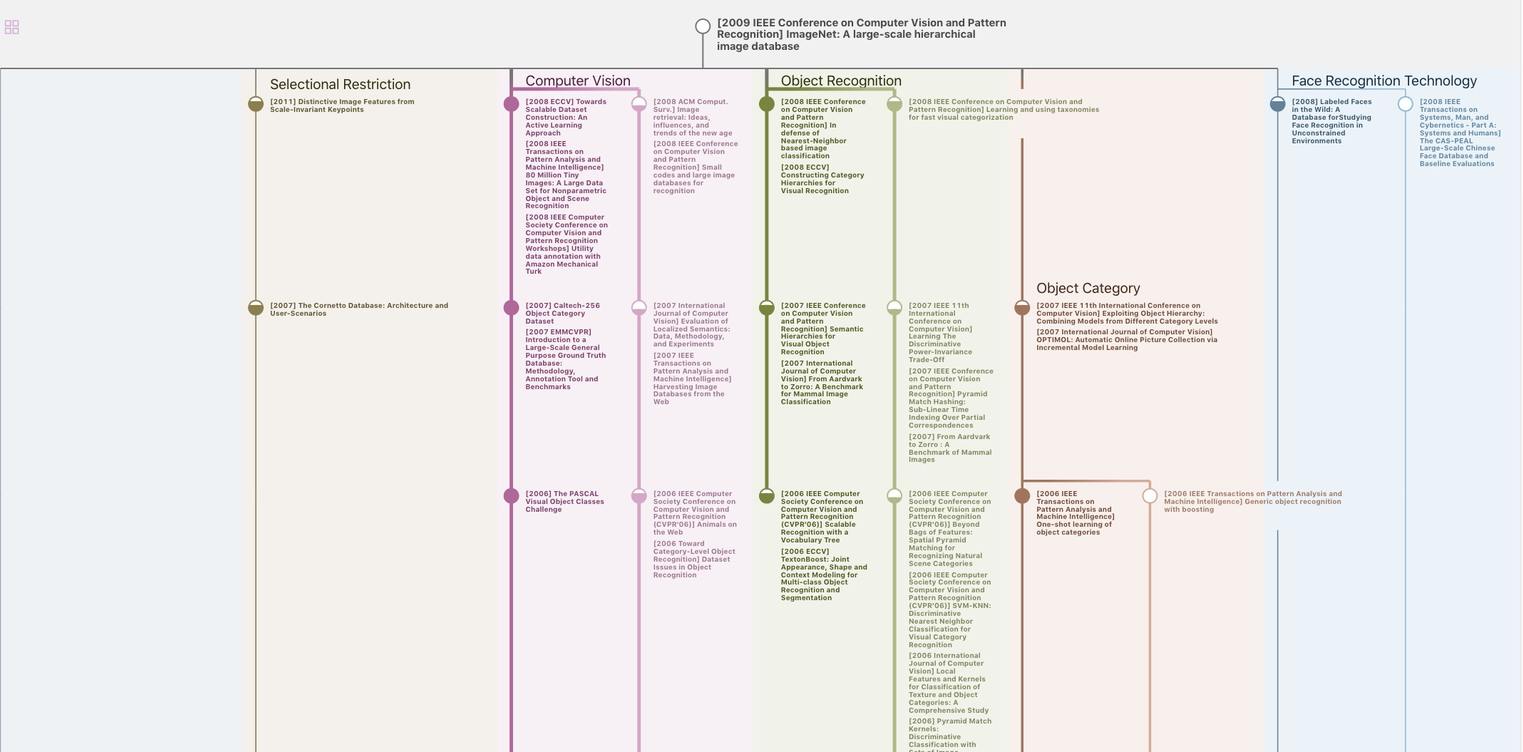
生成溯源树,研究论文发展脉络
Chat Paper
正在生成论文摘要