A Topic Modeling Framework for Humpback Whale Song
semanticscholar(2018)
摘要
Humpback whales produce intricate hierarchical patterns of repeated vocal elements called songs. Studying these songs requires consistent classification of song components and measurement of variation over time and geographic location. Performing such classification and measurements manually is time consuming and can produce subjective results that differ from study to study, which makes it difficult to perform large scale analyses and impedes comparability between results of different analyses. For these reasons, efforts have been made to develop automated methods for performing such analyses. These analyses have operated at the level of song units, using a manual or automated detection pass as a precursor to modeling, and using the units isolated by the detection pass as the model’s input. This paper explores the application of a probabilistic topic modeling framework to modeling humpback whale song, which does not require a detection pass as a precursor, and is a mixed membership model, allowing the model to adapt the evolving nature of humpback whale song. The findings presented in this paper indicate that the framework is capable of differentiating song units and background noise, and to a certain extent, between song units themselves.
更多查看译文
AI 理解论文
溯源树
样例
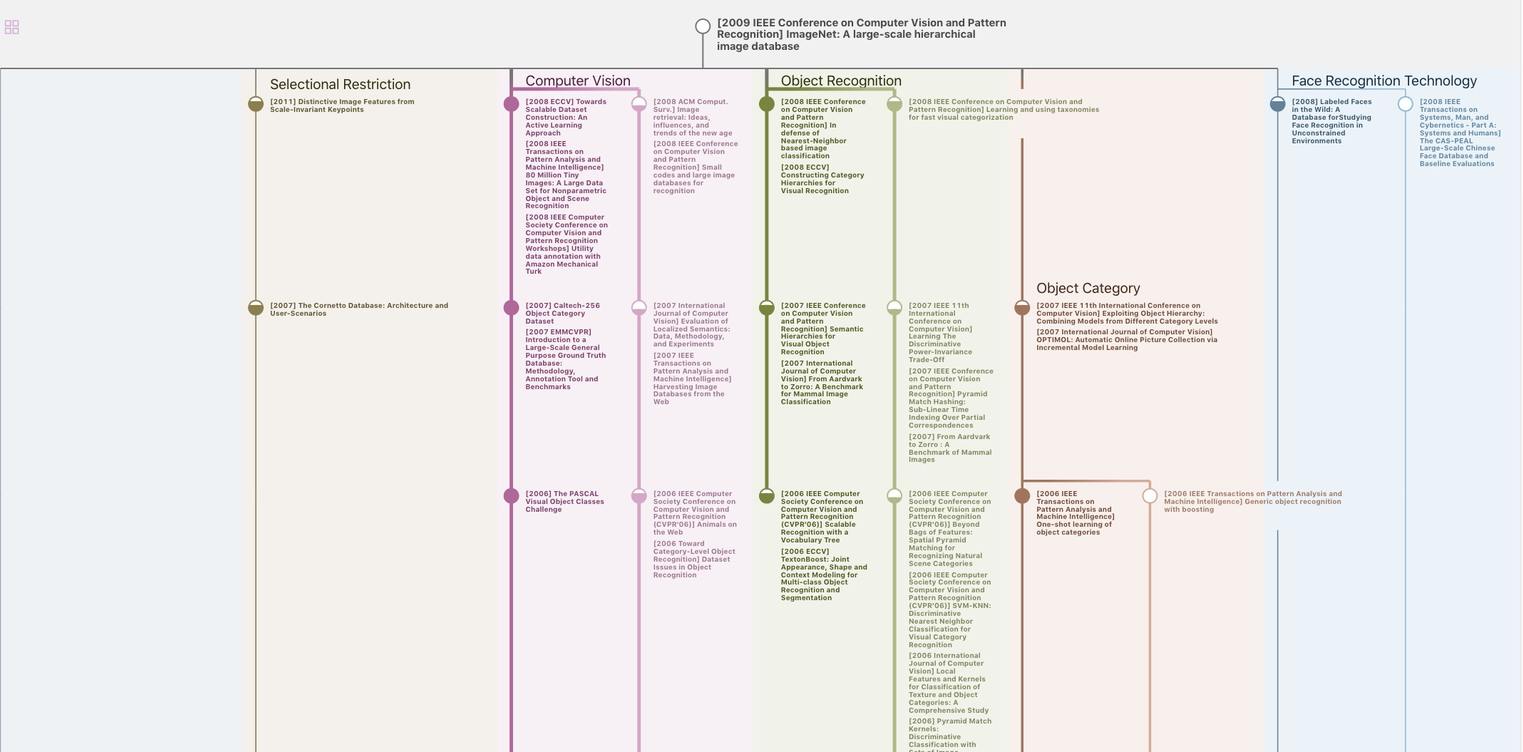
生成溯源树,研究论文发展脉络
Chat Paper
正在生成论文摘要