References 1] X.d. Huang and M.a. Jack. Uniied Techniques for Vector Quantization and Hidden Markov Modelling Using Semi-continuous Models. In
semanticscholar(2007)
摘要
comparison between diierent initialization methods produced quite similar results (g. 4). When random initial values were used, signiicantly more iterations were required both in the case of the Baum-Welch and of the segmental K-means algorithms to achieve good recognition rates. The SOMs were trained in this experiment as follows: The size of the SOM for each state was 5 times 5. Training data contains 5211 phonemes. Each phoneme sample was divided into 4 groups of feature vectors; one for each state in the HMM (see g. 1). The feature vectors were then used in random order to update the corresponding originally random valued SOMs. The training data was used 5 times during which the teaching gain was decreased monotonically from 0.2 to 0 and neighborhood radius from 3 to 0. Using LVQ algorithms to get more discriminative initialization produced low recognition error rates for the Baum-Welch training ((gure 3) and especially for the segmental K-means training ((gure 4). The codebook vectors of LVQ were initialized by nding a group of vectors which satisfy the K-nearest neighbor (KNN) criterion as suggested in 6]. The KNN criterion states that from the K-nearest neighbors in the training data the majority must belong to the same class as the tested vector. In this application of LVQ the vector to be updated corresponding to a training feature vector was selected by nding the closest Gaussian mean vector in the group of all mixture components in all state output density functions. For adjustments the learning laws LVQ1, LVQ2, LVQ3 5] and the optimized learning rate OLVQ1 6] were tried with almost equal recognition rates. In gures 3 and 4 the recognition error rates are illustrated for LVQ1 where the teaching gain was decreased monotonically from 0.05 to 0 and the whole training data set was used 2 times. 6 CONCLUSIONS It is shown by experiments that a careful initialization of the parameters determining the observation density functions of the states in CDHMMs speeds up the convergence and leads to better models (in average). The criterion by which the models are compared is the performance in speech recognition. The improvements due to better initialization occur both in the iterative Baum-Welch and in the segmental K-means algorithms. The increased speed of convergence allows the use of more accurate and complex models which require more training data and iterations in estimation. The new methods introduced in this paper for …
更多查看译文
AI 理解论文
溯源树
样例
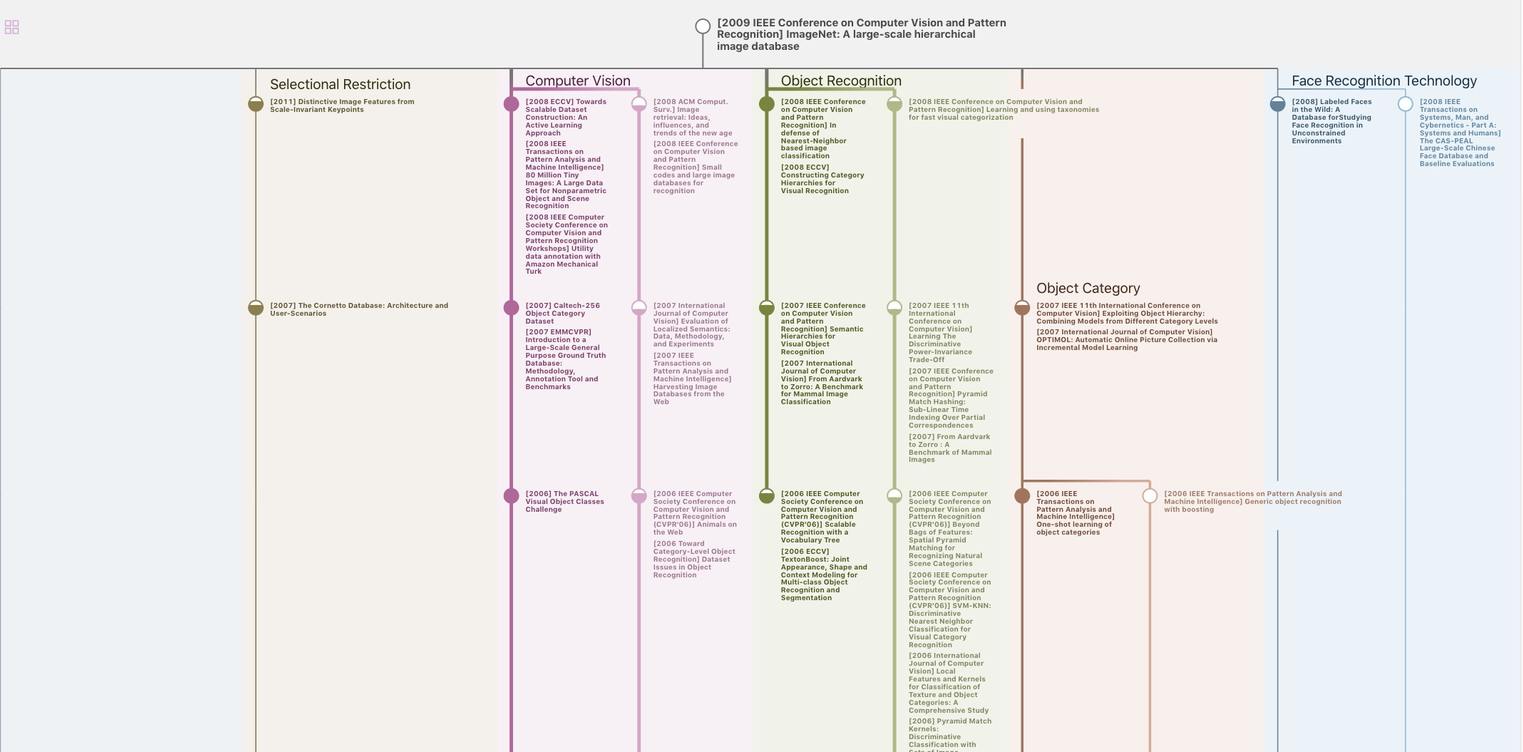
生成溯源树,研究论文发展脉络
Chat Paper
正在生成论文摘要