Interpreting Models of Student Interaction in Immersive Simulation Settings
semanticscholar(2020)
摘要
Immersive simulations are increasingly used for teaching and training in many societally important arenas including healthcare, disaster response and science education. The interactions of students in such settings leads to a complex array of emergent outcomes that present challenges for analysis. This paper studies a central element of such an analysis, namely the interpretability of models for inferring structure in time series data that are generated by the immersive simulations. This problem is explored in the context of modeling student interactions in an ecological-system simulation. Unsupervised machine learning is applied to data on system dynamics with the aim of helping teachers determine the effects of students’ actions on these dynamics. We address the question of choosing the optimal machine learning model, considering both statistical information criteria and interpretabilty quality. Our approach adapts two interpretability tests from the literature that measure the agreement between the model output and human judgment. The results of a user study show that the models that are the best understood by people are not those that optimize information theoretic criteria. This is a challenge for education settings as we cannot guarantee optimally interpretable models by choosing to optimise a statistical metric. We conclude that it is important to consider the interpretability of machine learning models as a separate optimization objective to statistical likelihood metrics when deploying models that hope to provide explanations of the complex dynamics occurring in rich embodied simulations.
更多查看译文
AI 理解论文
溯源树
样例
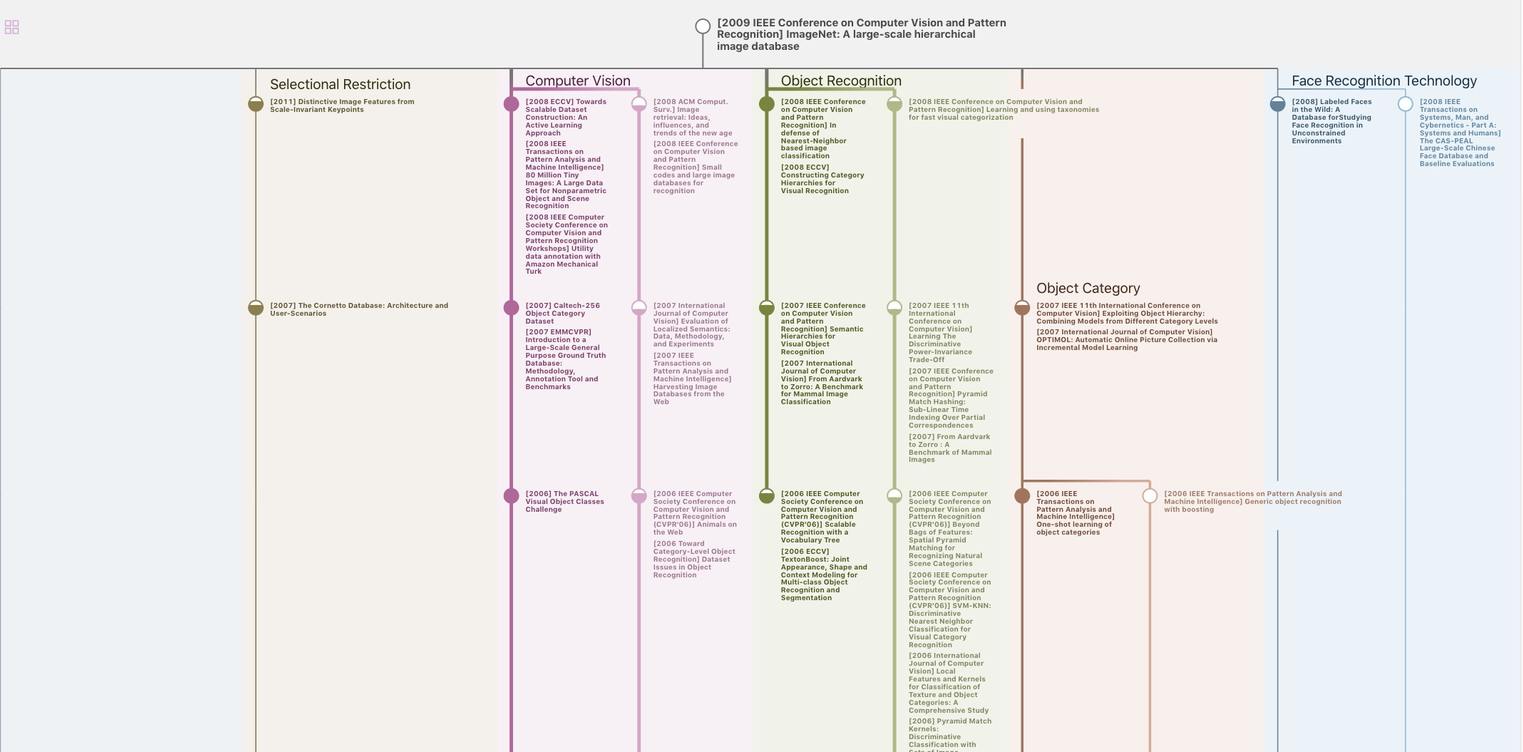
生成溯源树,研究论文发展脉络
Chat Paper
正在生成论文摘要