A robust gap-filling method for Net Ecosystem CO 2 Exchange based on image inpainting ( IIP )
semanticscholar(2016)
摘要
Traditional gap-filling approaches adopt a temporally linear perspective on data; whether synthesizing data statistically within a moving window, or using complex functions based on a “best-guess” understanding of the processes driving exchange. The former approach is limited in its ability to capture non-linear trends, and the latter is limited in situations where the flux response to driving variables is poorly understood or unknown (e.g. the response of gas exchange to water table depth in wetlands). Rearranging time-averaged half-hourly net ecosystem exchange (NEE) into a 48*N matrix 10 has been used to visualize NEE as a “flux fingerprint” and suggests a different way of filling data gaps. In this paper, we introduce an image processing technique known as image inpainting to fill gaps in this two-dimensional representation of a one-dimensional data. This has the advantage that any short-term structure can be accommodated without expressly implying any particular functional response to driving environmental variables, and medium-term temporal structure (i.e. day-to-day covariance) can be incorporated into gaps in the flux signal. In this way, data gaps are filled solely using information 15 contained in robust, primary data. This new method compares favourably with the marginal distribution sampling (MDS), when tested on twelve European-Flux datasets with four types of artificial gaps. Furthermore, we show that how random structures or noise embedded in the signal affect the gap-filling performance, which can simply be improved through a denoising procedure by using a Fourier transform algorithm. The inpainting-based gap-filling approach is more effective than MDS on the de-noised data. 20
更多查看译文
AI 理解论文
溯源树
样例
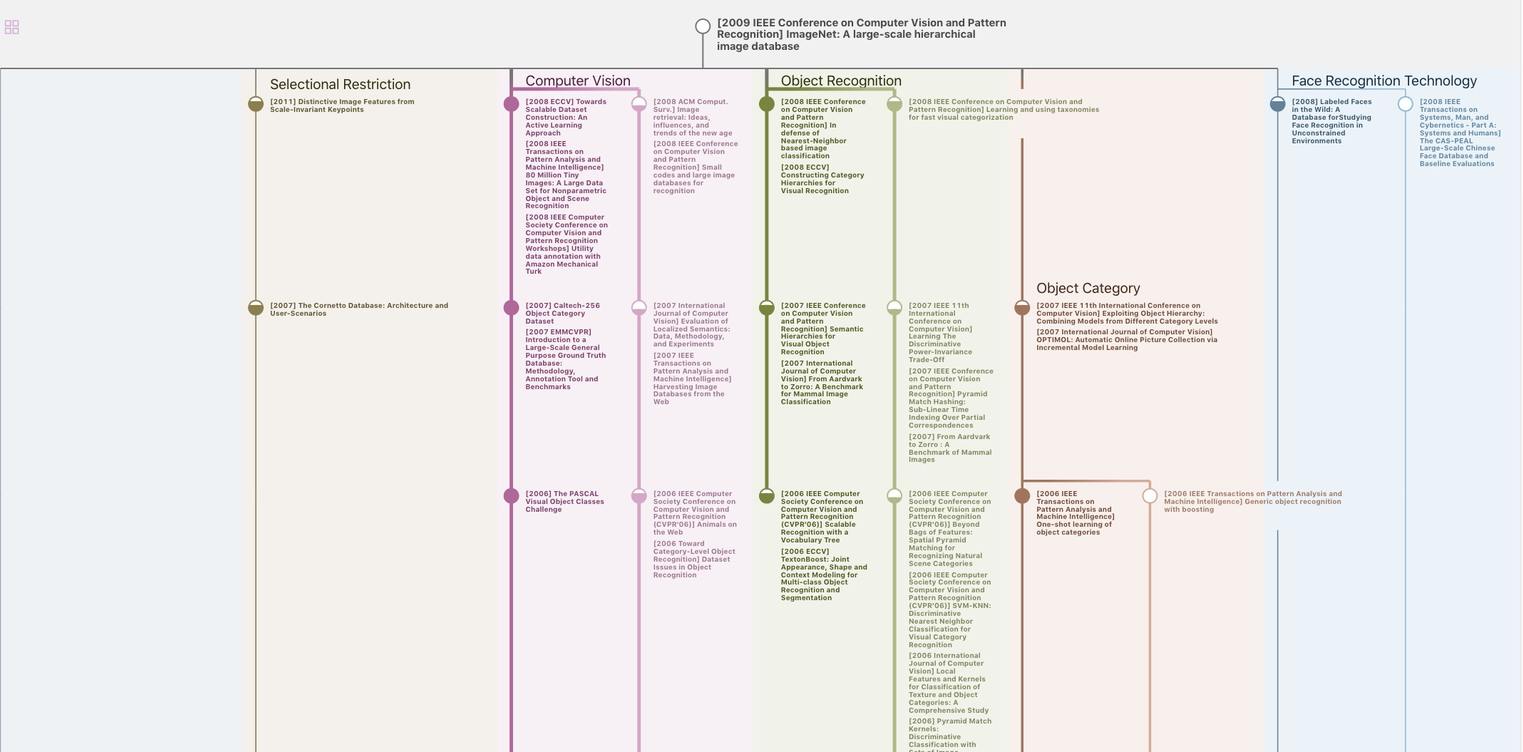
生成溯源树,研究论文发展脉络
Chat Paper
正在生成论文摘要