Detection of Prostate Cancer using Multi-parametric Magnetic Resonance
semanticscholar(2014)
摘要
A multi-channel statistical classifier to detect prostate cancer was developed by combining information from 3 different MR methodologies: T2-weighted, T2-mapping, and Line Scan Diffusion lmaging(LSDI). From these MR sequences, 4 sets of image intensities were obtained: T2-weighted(T2W) from T2-weighted imaging, Apparent Diffusion Coefficient(ADC) from LSDI, and Proton Density (PD) and T2 (T2Map) from T2-mapping imaging. Manuallysegmented tumor labels from a radiologist were validated by biopsy results to serve as tumor "ground truth." Textural features were derived from the images using co-occurrence matrix and discrete cosine transform. Anatomical location of voxels was described by a cylindrical coordinate system. Statistical jack-knife approach was used to evaluate our classifiers. Single-channel maximum likelihood(ML) classifiers were based on 1 of the 4 basic image intensities. Our multi-channel classifiers: support vector machine (SVM) and fisher linear discriminant(FLD), utilized 5 different sets of derived features. Each classifer generated a summary statistical map that indicated tumor likelihood in the peripheral zone(PZ) of the gland. To assess classifier accuracy, the average areas under the receiver operator characteristic (ROC) curves were compared. Our best FLD classifier achieved an average ROC area of 0.839 (±0.064) and our best SVM classifier achieved an average ROC area of 0.761 (±0.043). The T2W intensity maximum likelihood classifier, our best single-channel classifier, only achieved an average ROC area of 0.599 (± 0.146). Compared to the best single-channel ML classifier, our best multi-channel FLD and SVM classifiers have statistically superior ROC performance with P-values of 0.0003 and 0.0017 respectively from pairwise 2-sided t-test. By integrating information from the multiple images and capturing the textural and anatomical features in tumor areas, the statistical summary maps can potentially improve the accuracy of image-guided prostate biopsy and enable the delivery of localized therapy under image guidance. Thesis Supervisors: William Wells 111, Eric Grimson PREFACE ................................................................................................................................. 2 PART 1: TH E EXPERIM ENT ................................................................................................ 2 INTRODUCTION ....................................................................................................................... 2 M ATERIALS AND M ETHODS ........................................................................................... 4 Patient selection and imaging protocols ........................................................................... 4 Tumor "ground-truth " labels ............................................................................................ 5 Texturalfeatures ................................................................................................................ 5 Anatomicalfeatures .......................................................................................................... 6 F e a tu re se ts ....................................................................................................................... 6 Statistical classifiers ......................................................................................................... 6 S of tw a re ............................................................................................................................ 6 Classifier accuracy ........................................................................................................... 6 RESULTS .............................................................................................................................. 7 Pathology summary .......................................................................................................... 7 Signal intensity statistics ................................................................................................... 7 Classifier accuracy ........................................................................................................... 8 CONCLUSION S ................................................................................................................. 11 PART 2: SOFTW AR E TOOLS ............................................................................................. 17 GETTING STARTED: DOWNLOADING JAVA ............................................................................. 17 BASIC COMMANDS ............................................................................................................... 17 Loading images ............................................................................................................... 17 Adjusting Window/Level .................................................................................................. 17 Viewing to a Different Slice ............................................................................................. 18 Im a g e S ize ....................................................................................................................... 1 8 Scan Information ............................................................................................................. 18 Export to M ATLAB .......................................................................................................... 18 Export to Slicer ............................................................................................................... 18 Configuration .................................................................................................................. 19 Closing ImagelExiting .................................................................................................... 19 SEGMENTATION AND REGION OF INTEREST (ROI) ................................................................ 19 DrawinglErasinglHiding a ROI ...................................................................................... 19 LoadinglSaving ROI ....................................................................................................... 20 Export an ROI to Slicer ................................................................................................... 20 CopyinglSubtracting 2 ROIs ........................................................................................... 20 Biopsy Validated ROI ...................................................................................................... 20 IMAGE STATISTICS ................................................................................................................ 20 R O I Vo lu m e ..................................................................................................................... 2 1 Histogram: Image, ROI, Smap Histogram ..................................................................... 21 Correlation Coefficients and Scatter Plots ..................................................................... 21 FITTING ONE IMAGE VOLUME INTO ANOTHER: RESAMPLING ................................................ 21 CLASSIFIER TRAINING .......................................................................................................... 22 Standardizing Feature ..................................................................................................... 22 Build Co-occurrence matrices ........................................................................................ 22 Building Classifiers: FLD and SVM ............................................................................... 22 SUMMARY STATISTICAL M AP (SMAP) ................................................................................... 22 Generating a SmaplApplying a Classifier ....................................................................... 22 Loading a Smap .............................................................................................................. 23 Controlling Smap Appearance ........................................................................................ 23 Smap Error RatelROC ................................................................................................... 24 ACK NOW LEDGEM ENTS ................................................................................................... 25 REFERENCES ....................................................................................................................... 26
更多查看译文
AI 理解论文
溯源树
样例
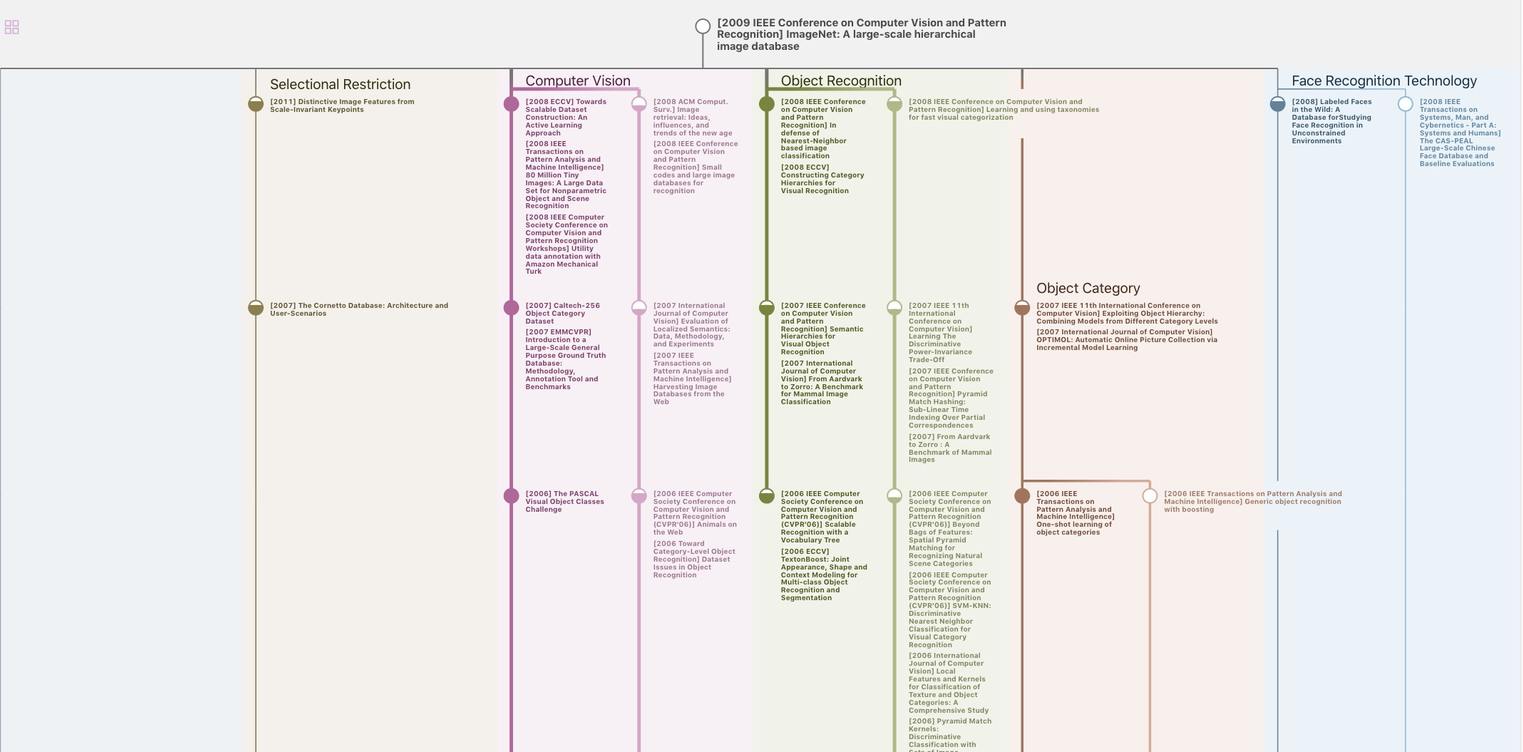
生成溯源树,研究论文发展脉络
Chat Paper
正在生成论文摘要