Presented at the Task-Agnostic Reinforcement Learning Workshop at ICLR 2019 U NSUPERVISED D ISCOVERY OF D ECISION S TATES THROUGH I NTRINSIC C ONTROL
semanticscholar(2019)
摘要
Learning diverse and reusable skills in the absence of rewards in an environment is a key challenge in reinforcement learning. One solution to this problem, as has been explored in prior work (Gregor et al., 2016; Eysenbach et al., 2018; Achiam et al., 2018), is to learn a set of intrinsic macro-actions or options that reliably correspond to trajectories when executed in an environment. In this options framework, we identify and distinguish between decision-states (e.g. crossroads) where one needs to make a decision, as being distinct from corridors (where one can follow default behavior) in the modeling of options. Our intuition is that identifying decision states would lead to more interpretable behavior from an RL agent, exposing clearly what the underlying options correspond to. We formulate this as an information regularized intrinsic control problem using techniques similar to (Goyal et al., 2019) who applied the information bottleneck to goal-driven tasks. Our qualitative results demonstrate that we learn interpretable decision states in an unsupervised manner by merely interacting with the environment.
更多查看译文
AI 理解论文
溯源树
样例
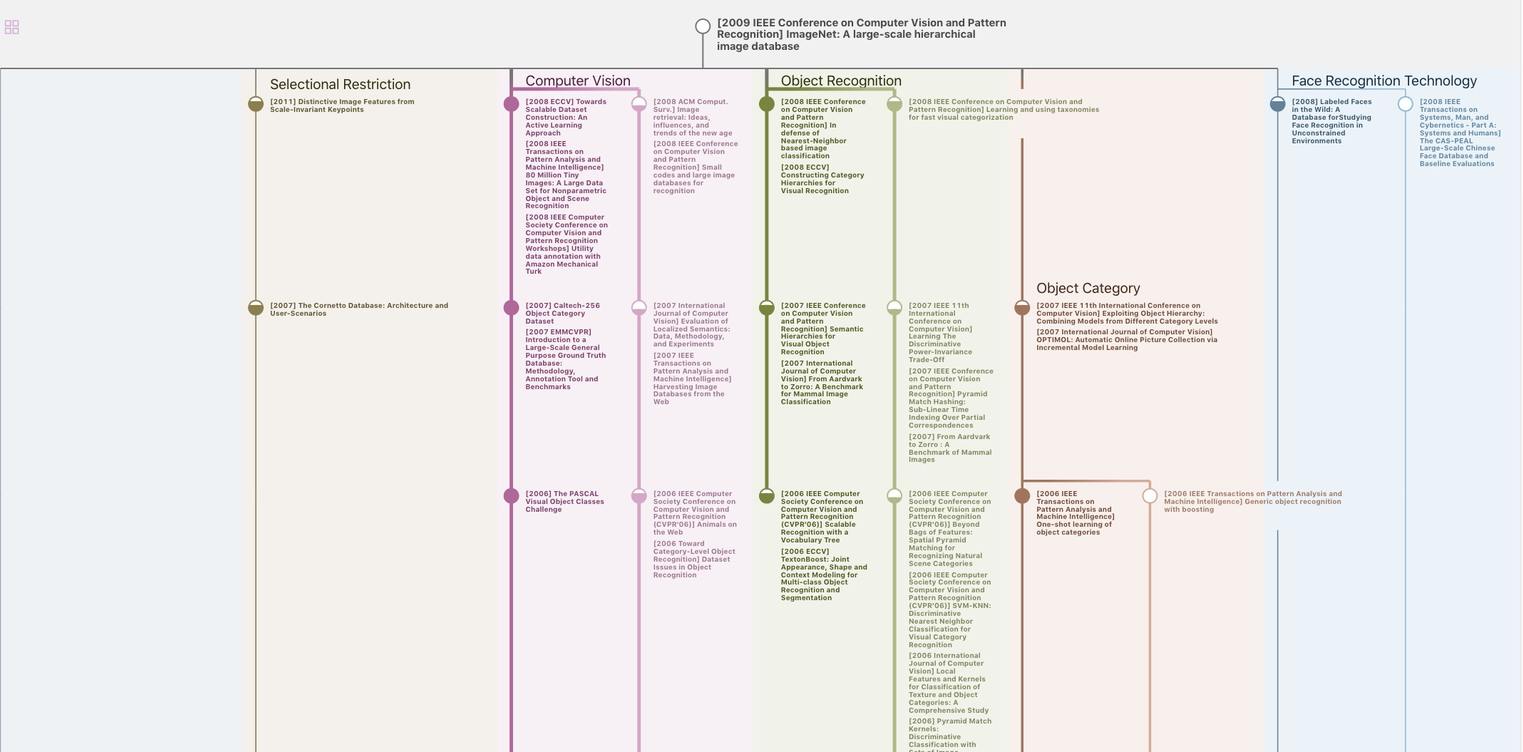
生成溯源树,研究论文发展脉络
Chat Paper
正在生成论文摘要