Using a Hierarchical Model to Get the Best of Both Worlds: Good Prediction and Good Explanation
semanticscholar(2019)
摘要
Understanding how learning transfers from one task to another is a critical topic in learning science. In this paper, we investigate the impact of the scope and granularity of learning transfer by comparing three models across multiple data sets. Prior work demonstrated the value of component models of learning transfer that group items into knowledge components. Within the component models, that work left open whether difficulty (variation in performance over tasks) is better modeled by knowledge components (the strong model) or by items (the “weak” model). The strong component model is theoretically desirable because it provides a single explanation for both difficulty and transfer. However, we find that the weak component model better predicts student performance across six data sets. While this weak model predicts better, it is hard to interpret because an explanatory parameter that represents latent knowledge difficulty of student performance is absent. To maintain explanatory power without sacrificing prediction, we propose a new alternative that uses a hierarchical mixed effect regression model where item difficulty is pooled within component difficulty. Experimental results, across six data sets, show that the predictions of the hierarchical model are better than the strong model and as good as the weak model, while also producing theoretical useful explanatory parameter values for knowledge components.
更多查看译文
AI 理解论文
溯源树
样例
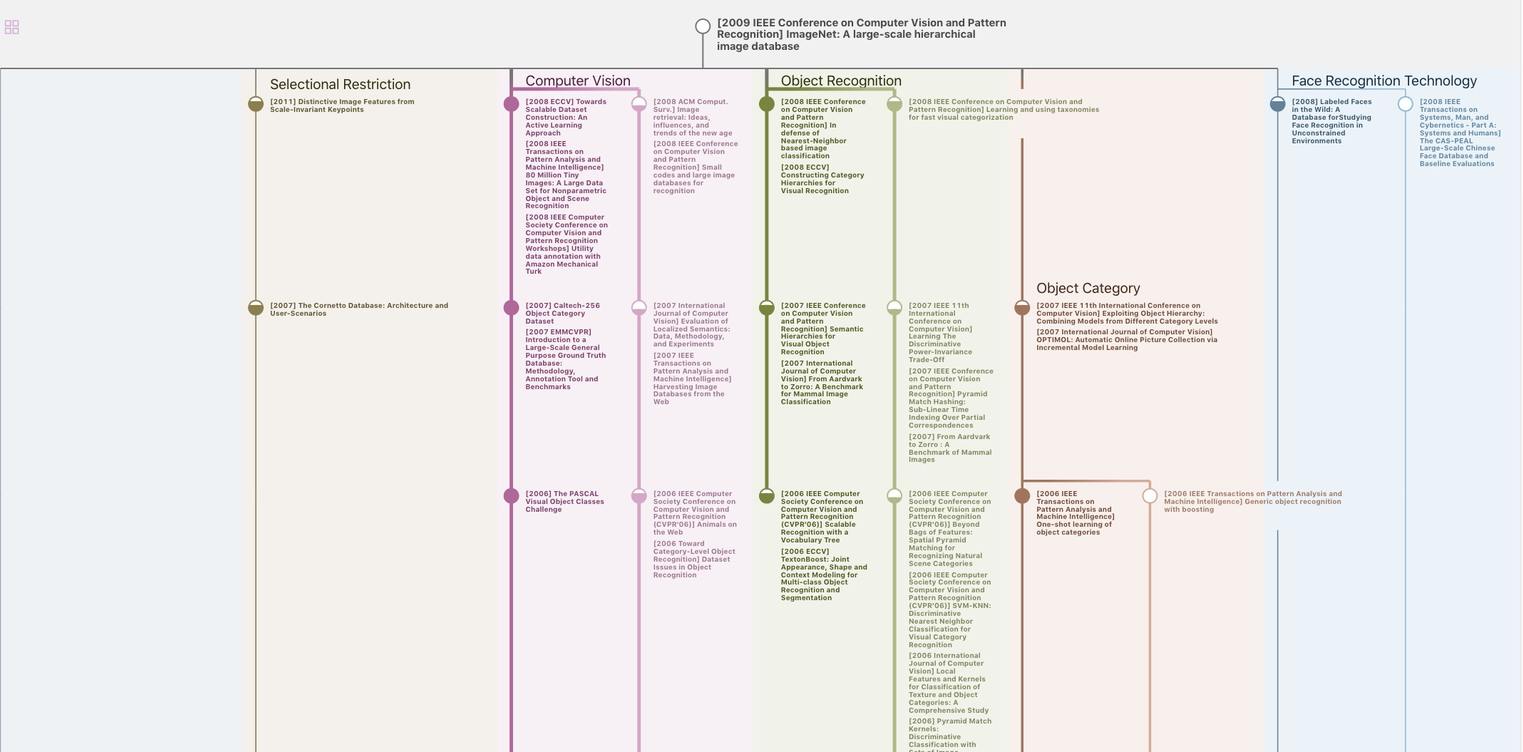
生成溯源树,研究论文发展脉络
Chat Paper
正在生成论文摘要