Counterfactual learning in networks : an empirical study of model dependence
semanticscholar(2019)
摘要
Within the potential outcomes framework for causal inference, the choice of unit features and matching algorithms can impact the estimated causal effects, a problem known as model dependence. Here, we look at this problem in the context of observational network data and recently developed network representations within machine learning. By varying node representations, matching models, and methods for causal effect estimation on synthetic and real-world graph datasets, we show experimentally that estimated causal effects can vary significantly, both in sign and magnitude. With this paper, we aim to highlight some of the challenges of estimating causal effects from observational network data and hope to inspire further studies on model dependence in causal inference.
更多查看译文
AI 理解论文
溯源树
样例
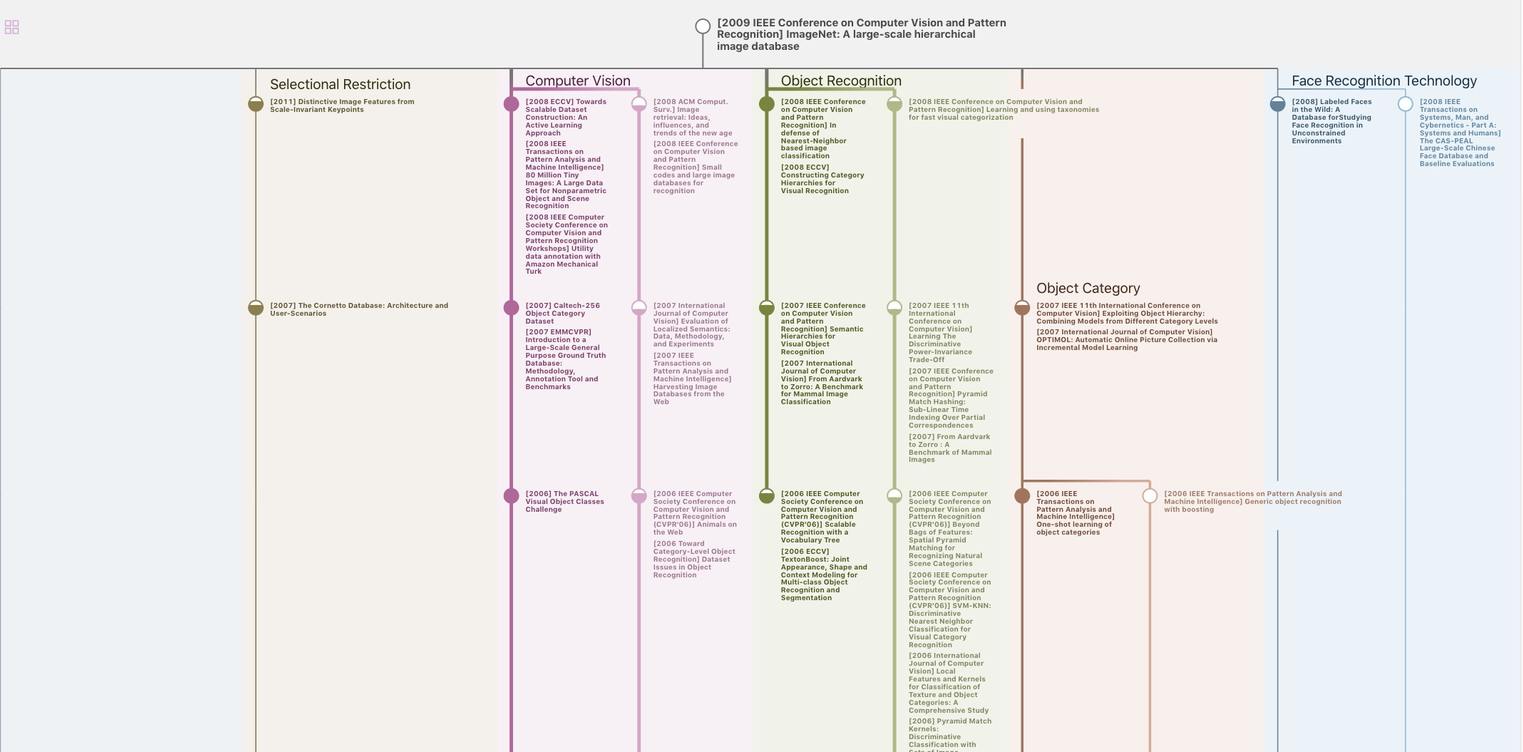
生成溯源树,研究论文发展脉络
Chat Paper
正在生成论文摘要