Experimentation in a Model-Based Game
semanticscholar(2019)
摘要
One challenge for building software organisms is to support more autonomous, self-directed learning, rather than learning from annotated data or blindly exploring state spaces. We present a mechanism for learning a simple game given a qualitative model that provides partial information about how actions and quantities influence each other and about which goals trade off with each other. That information lets the learner progressively rule out unproductive actions based on qualitative state descriptions of the current situation and to experimentally adjust the relative importance of competing goals. We show that this amounts to operationalizing a qualitative model into a quantitative prescriptive model, which can lead to rapid improvement in performance on a game. In our experiments with a simple Human Resources Management game, it learned to win after one trial and continued to improve its score and reduced the number of actions needed to win throughout the next nine trials.
更多查看译文
AI 理解论文
溯源树
样例
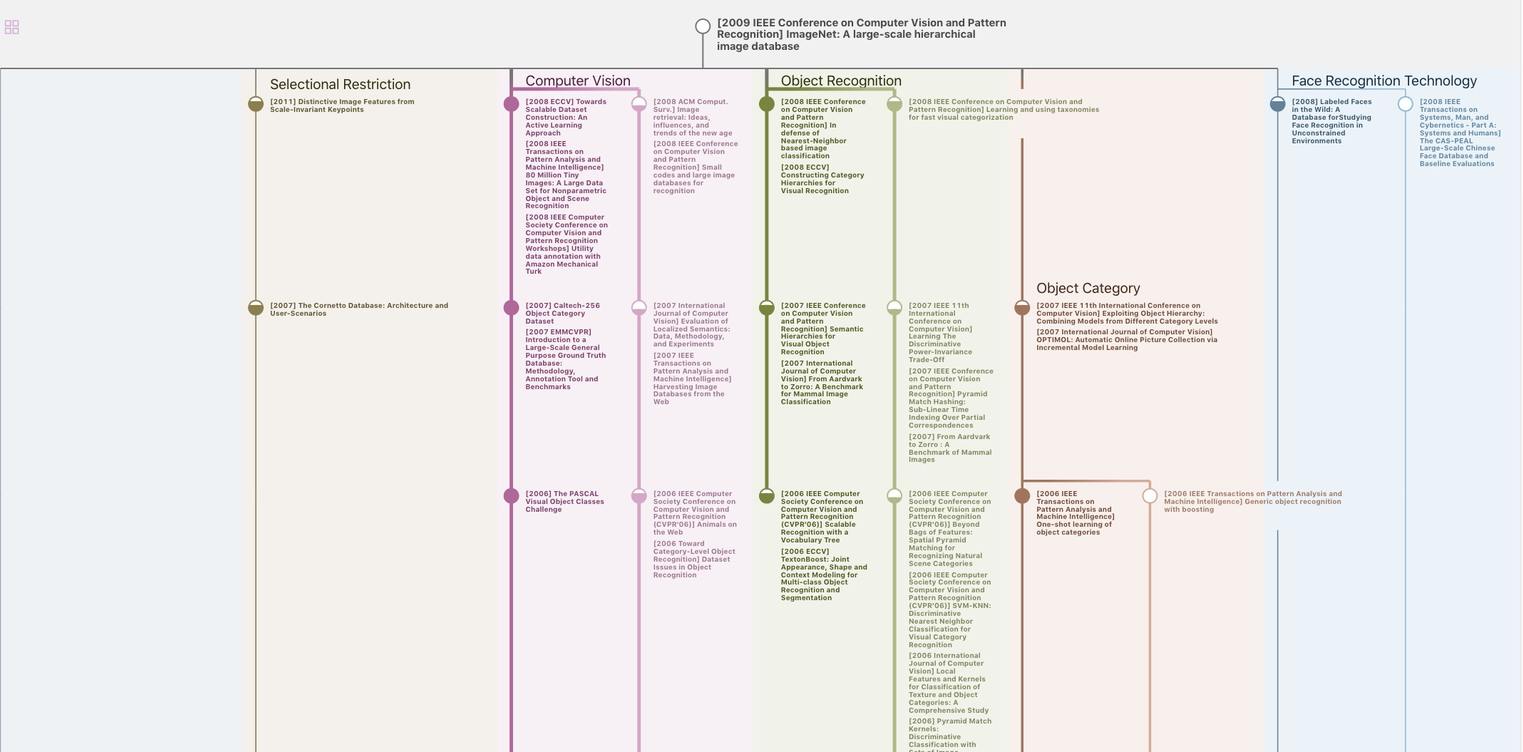
生成溯源树,研究论文发展脉络
Chat Paper
正在生成论文摘要