PROTEOMIC BIOMARKER STUDY USING NOVEL ROBUST PENALIZED ELASTIC NET ESTIMATORS By
semanticscholar(2018)
摘要
In large-scale quantitative proteomic studies, scientists measure the abundance of hundreds or thousands of proteins from the human proteome in search of novel biomarkers for a given disease. Despite current innovations in biomedical technologies, advanced statistical and computational methods are still required to harness the rich information contained in these large and complex datasets. While penalized regression estimators can be used to identify potential biomarkers among a large set of molecular features, it is well-known that the performance and statistical properties of the selected model depend on the loss and penalty functions used to construct the regularized estimator. For example, the presence of outlying observations in the data can seriously affect classical estimators that penalize the square error loss function. Similarly, the choice of the penalty function in these estimators is important to be able to preserve groups of correlated proteins in the selected model. Thus, in this paper we propose a new class of penalized robust estimators based on the elastic net penalty, which can be tuned to keep groups of correlated variables together as they enter or leave the model, while protecting the resulting estimator against possibly aberrant observations in the dataset. Our robust penalized estimators have very good robustness properties and are also consistent under relatively weak assumptions. In this paper we also propose an efficient algorithm to compute our robust penalized estimators and we derive a data-driven method to select the penalty term, which is a critical part of any application with real data. Our numerical experiments show that our proposals compare favorably to other robust penalized estimators. Noteworthy, our robust estimators identify new potentially relevant biomarkers of cardiac allograft vasculopathy that are not found with non-robust alternatives. Importantly, the selected model is validated in a new set of 52 test samples, achieving an area under the receiver operating characteristic curve (AUC) of 0.85. *Supported by NSERC Discovery Grant MSC 2010 subject classifications: Primary 62J; secondary 62J05, 62J07, 62J07; Primary 62P; secondary 62P10; Primary 62F; secondary 62F35
更多查看译文
AI 理解论文
溯源树
样例
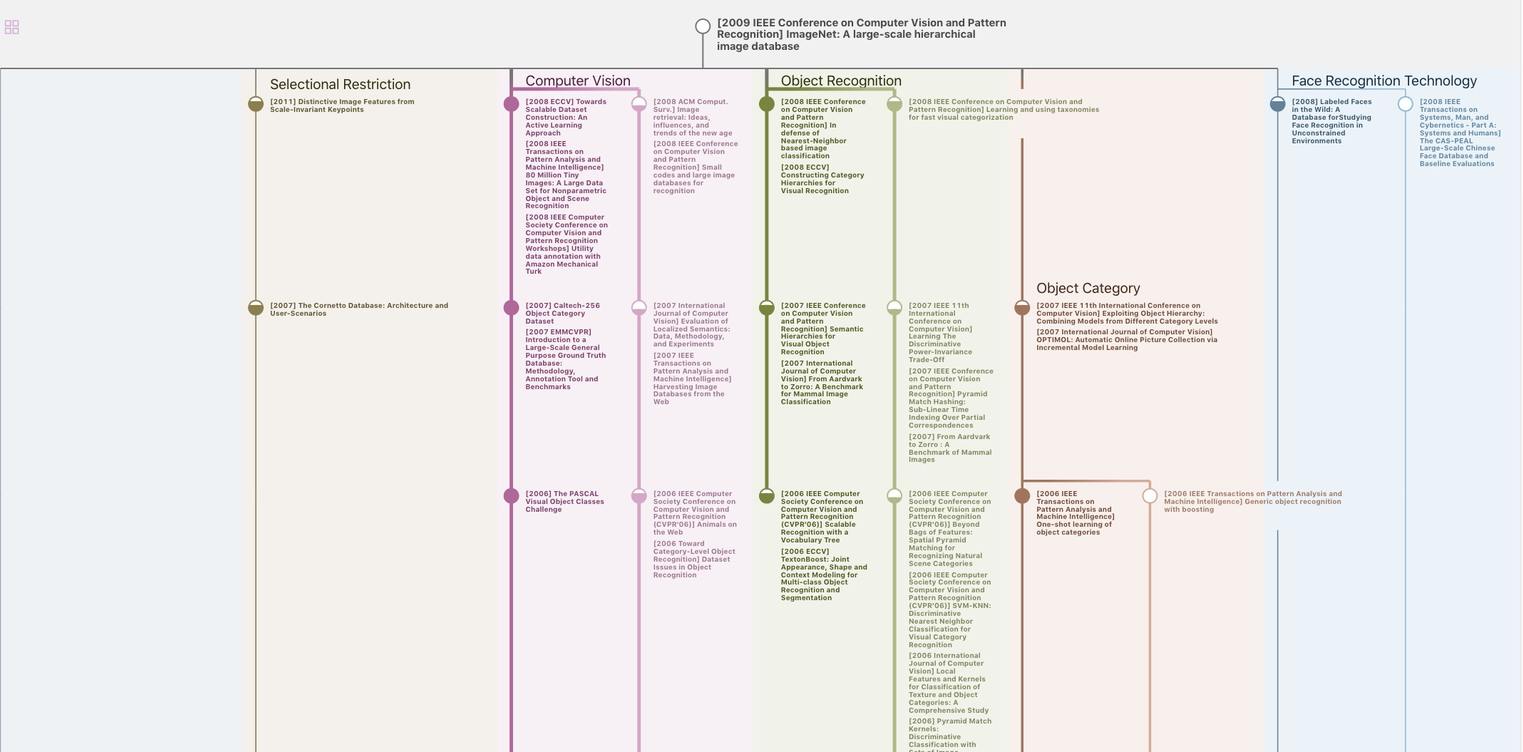
生成溯源树,研究论文发展脉络
Chat Paper
正在生成论文摘要