Mapping and Characterizing Global-Scale Human Settlements Using HPC
semanticscholar(2013)
摘要
Spatiotemporal analytics derived from large-scale analysis of satellite imagery is a key component in the models used for global population mapping, risk analysis, and critical infrastructure assessment. Making informed national-level decisions based on image derived analytics require processing petabytes of high resolution satellite image data. The computational process often involves extracting, representing, and classifying visual data patterns to detect different types of man-made structures [1]. Computing numerical feature descriptors from raw image data is an expensive but key step in the computational process. The scale of the computational process and the need to repeat the process at time stipulated intervals can easily saturate conventional CPUs. As a feasible solution, we have built a computational system based on GPGPU architecture for deriving big image data analytics [7]. In this study, we demonstrate an efficient GPU implementation for multi-scale feature extraction and exploit multi-GPU architecture for large-scale image analysis for characterizing human settlements.
更多查看译文
AI 理解论文
溯源树
样例
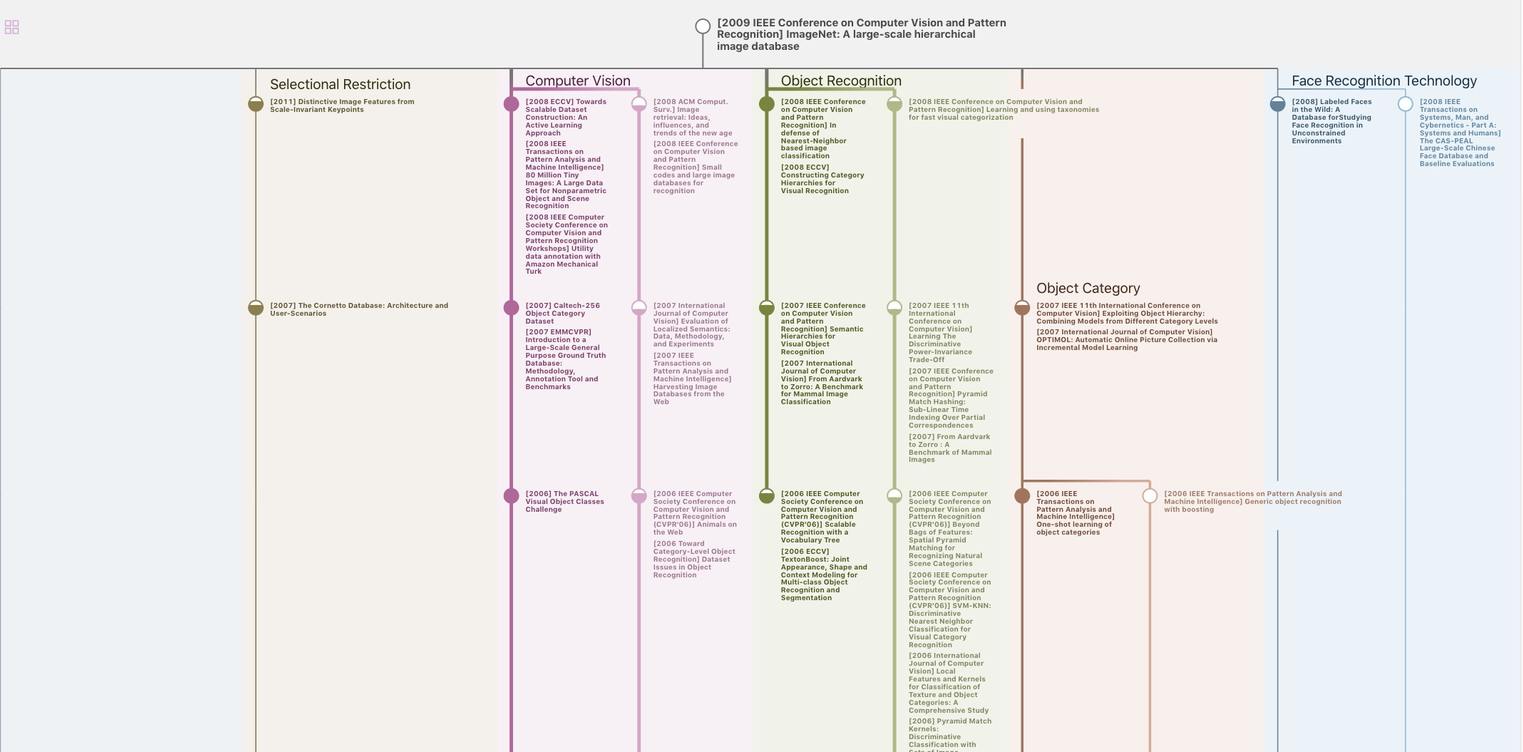
生成溯源树,研究论文发展脉络
Chat Paper
正在生成论文摘要