Efficacy of the Extended Principal Orthogonal Decomposition Method on DNA Microarray Data in Cancer Detection
semanticscholar
摘要
Recent advances in microarray technology offer the ability to study the expression of thousands of genes simultaneously. The DNA data stored on these microarray chips can provide crucial information for early clinical cancer diagnosis. The Principal Orthogonal Decomposition (POD) method has been widely used as an effective feature detection method. In this paper, we present an enhancement to the standard approach of using the POD technique as a disease detection tool. In the standard method, cancer diagnosis of an arbitrary sample is based on its correlation value with the cancerous or normal signature extracted using the POD method on DNA microarray data. In this paper, we extend the POD method by feeding the extracted principal features into Machine Learning algorithms to detect cancer. Particularly, Linear Support Vector Machine, Feed Forward Back Propagation Networks, and Self-Organizing Maps have been used on liver cancer, colon cancer, and leukemia data. Sensitivity, specificity, and accuracy have been used as a mean to evaluate predictive abilities of the proposed extended POD methods. Our results indicate overall the proposed methods provide slight improvements over the standard POD method.
更多查看译文
AI 理解论文
溯源树
样例
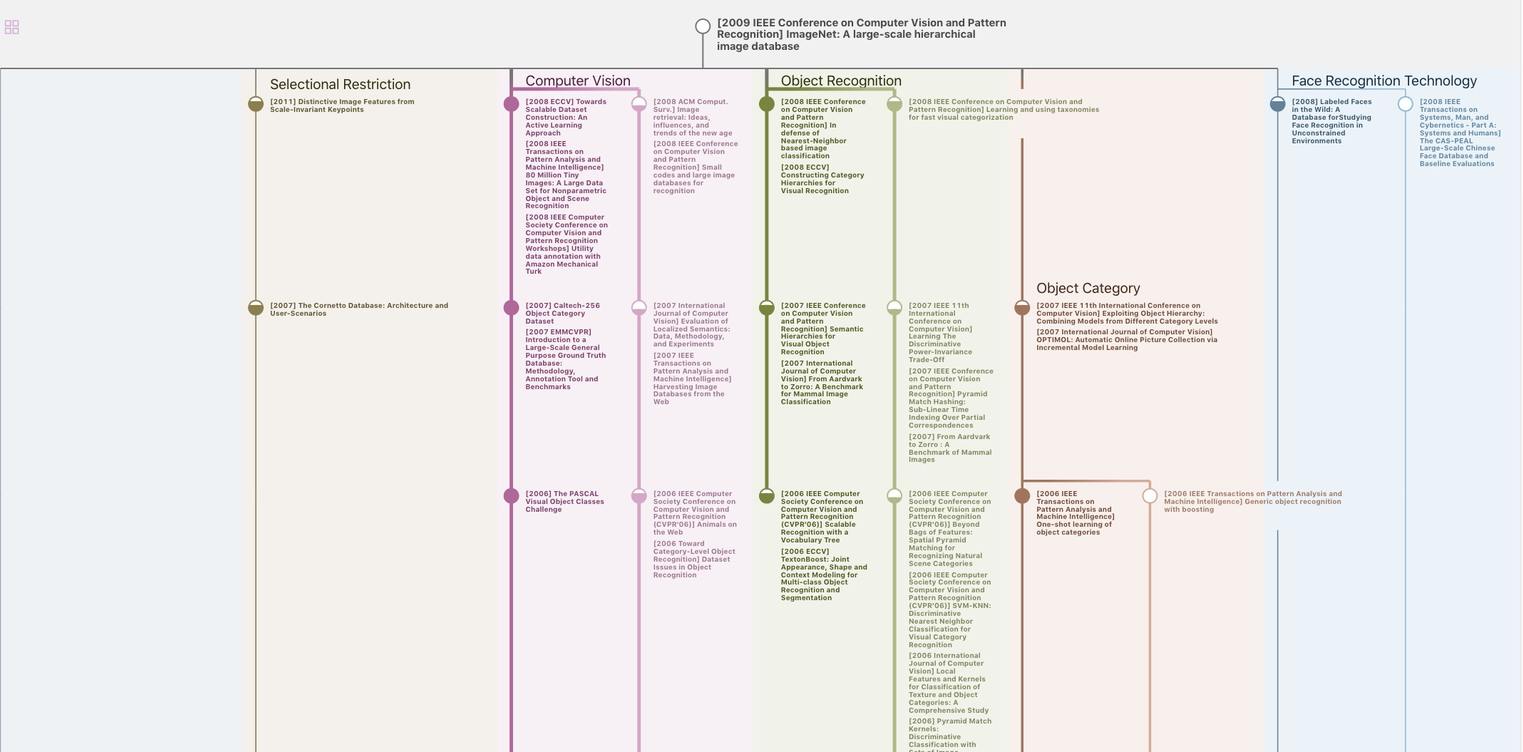
生成溯源树,研究论文发展脉络
Chat Paper
正在生成论文摘要