Learning Doubly Intractable Latent Variable Models via Score Matching
semanticscholar(2016)
摘要
Most current approaches for learning latent variable models, such as variational methods, require access to a normalized joint distribution. However, for models that do not belong to the standard, widely-studied parametric classes or that are derived from an undirected graphical model, the normalizer is often not readily available. Here we generalize the score matching approach [1] to learn a wide class of latent variable models based on joint exponential family (or maximum-entropy) distributions with arbitrary sufficient statistic vectors. We derive a stochastic gradient based optimization scheme that does not depend on the computation of normalizing constants for either of the joint or the posterior densities.
更多查看译文
AI 理解论文
溯源树
样例
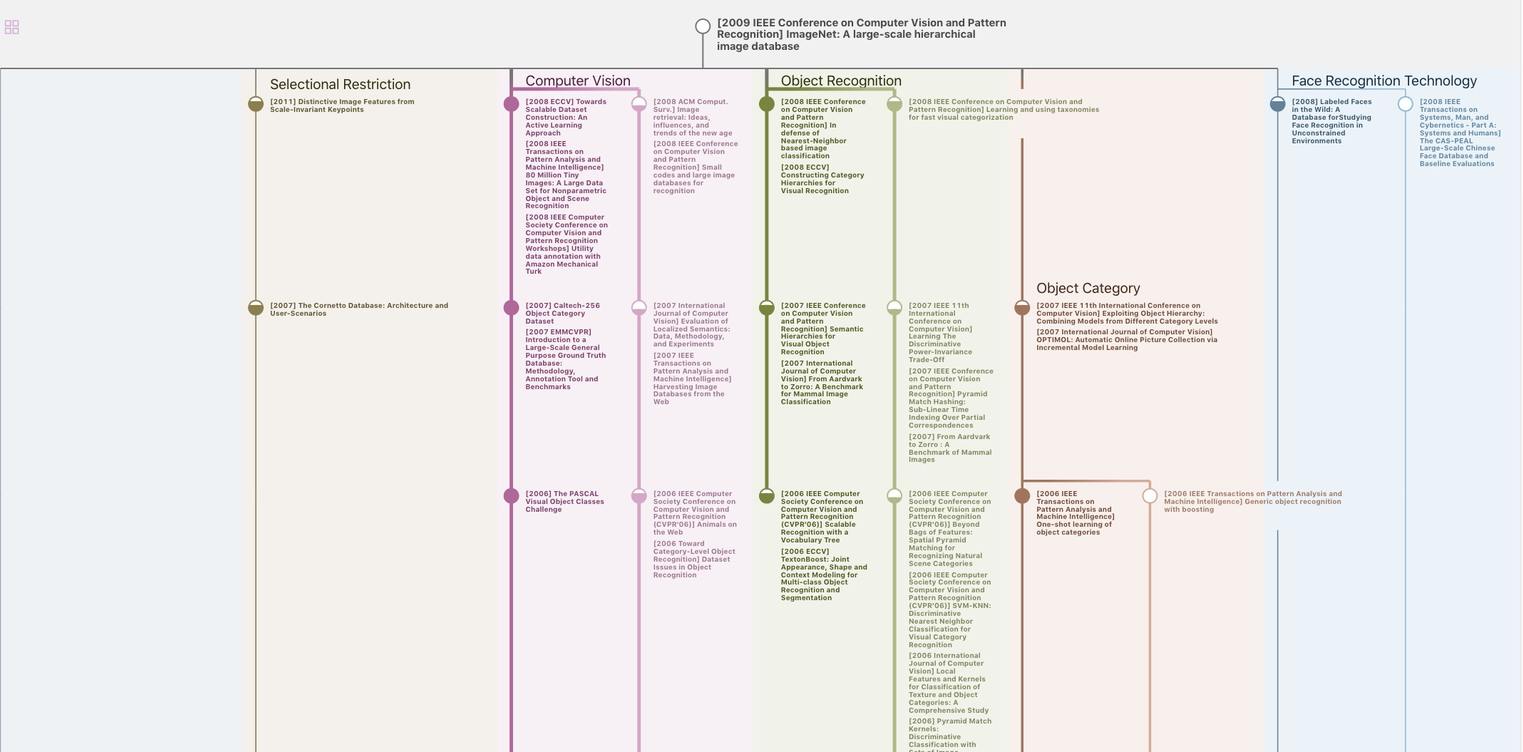
生成溯源树,研究论文发展脉络
Chat Paper
正在生成论文摘要