Evaluating RL Agents in Hanabi with Unseen Partners
semanticscholar(2020)
摘要
Hanabi is a cooperative game that challenges existing AI techniques due to its focus on modeling the mental states of other players to interpret and predict their behavior. While there are agents that can achieve near-perfect scores in the game by agreeing on some shared strategy, comparatively little progress has been made in ad-hoc cooperation settings, where partners and strategies are not known in advance. In this paper, we show that agents trained through self-play using the popular Rainbow DQN architecture fail to cooperate well with simple rule-based agents that were not seen during training and, conversely, when these agents are trained to play with any individual rule-based agent, or even a mix of these agents, they fail to achieve good self-play scores. Cooperative multi-agent problems with hidden information are challenging for humans and AI systems due to the need to model other actors’ mental states. This model can be used both to predict their future behavior and to infer unseen features of the world through the lens of their observed behavior. The ability to impute distinct mental states to oneself and others has been referred to as having a theory of mind (Premack and Woodruff 1978). Hanabi (Antoine Bauza, 2010) is a cooperative card game that has received attention of AI researchers because strategies for playing it rely heavily on theory of mind and communication. While agents that achieve near-perfect scores in a selfplay setting using a shared strategy have been developed for the game (Bouzy 2017; Foerster et al. 2018; Wu 2016), comparatively little progress has been made on ad-hoc cooperation settings, where the identity (and behavior) of other agents is not known in advance. In particular, there are to our knowledge no Reinforcement Learning (RL) agents designed to play either with humans or with simple rule-based agents inspired by human play such as the ones described by (Walton-Rivers et al. 2017). In this paper, we examine the behavior of RL agents trained using the Rainbow DQN architecture (Hessel et al. 2018) when paired with the aforementioned rule-based agents. The main question we address is: can these RL agents cooperate well with partners that were not seen during training? We answer this question negatively in two ways: first, we show that Rainbow agents trained purely through self-play perform very poorly when paired with the rule-based agents we selected. Second, we show that Rainbow agents that were trained with one or more rule-based agents as partners fail to play well with a particular “unseen” partner: itself. In other words, it fails to perform well in self-play, despite being able to achieve reasonable scores with its training partners.
更多查看译文
AI 理解论文
溯源树
样例
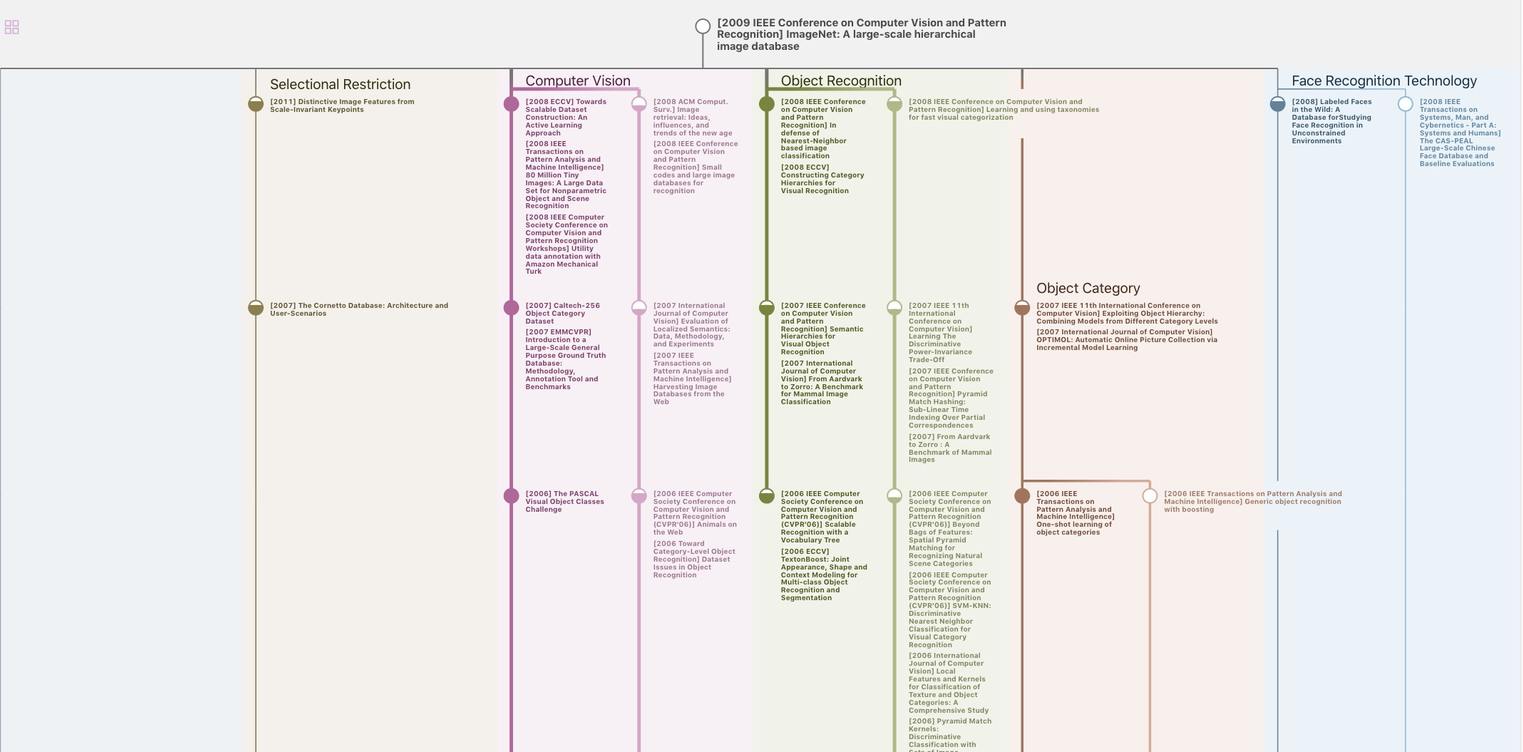
生成溯源树,研究论文发展脉络
Chat Paper
正在生成论文摘要