DynGAN: Generative Adversarial Networks for Dynamic Network Embedding
semanticscholar(2019)
摘要
Embedding large graphs in a low-dimensional space has proven useful in various applications. However, there is a limited focus on real-world networks that are dynamic in nature and continuously evolving with time. In this paper, we propose a novel adversarial algorithm to learn representation of dynamic networks. We leverage generative adversarial networks and recurrent networks to capture temporal and structural information. We conduct extensive experiments on the task of graph reconstruction, link prediction and graph prediction. Experimental results demonstrate consistent, stable, and better results against state-of-the-art methods in many cases.
更多查看译文
AI 理解论文
溯源树
样例
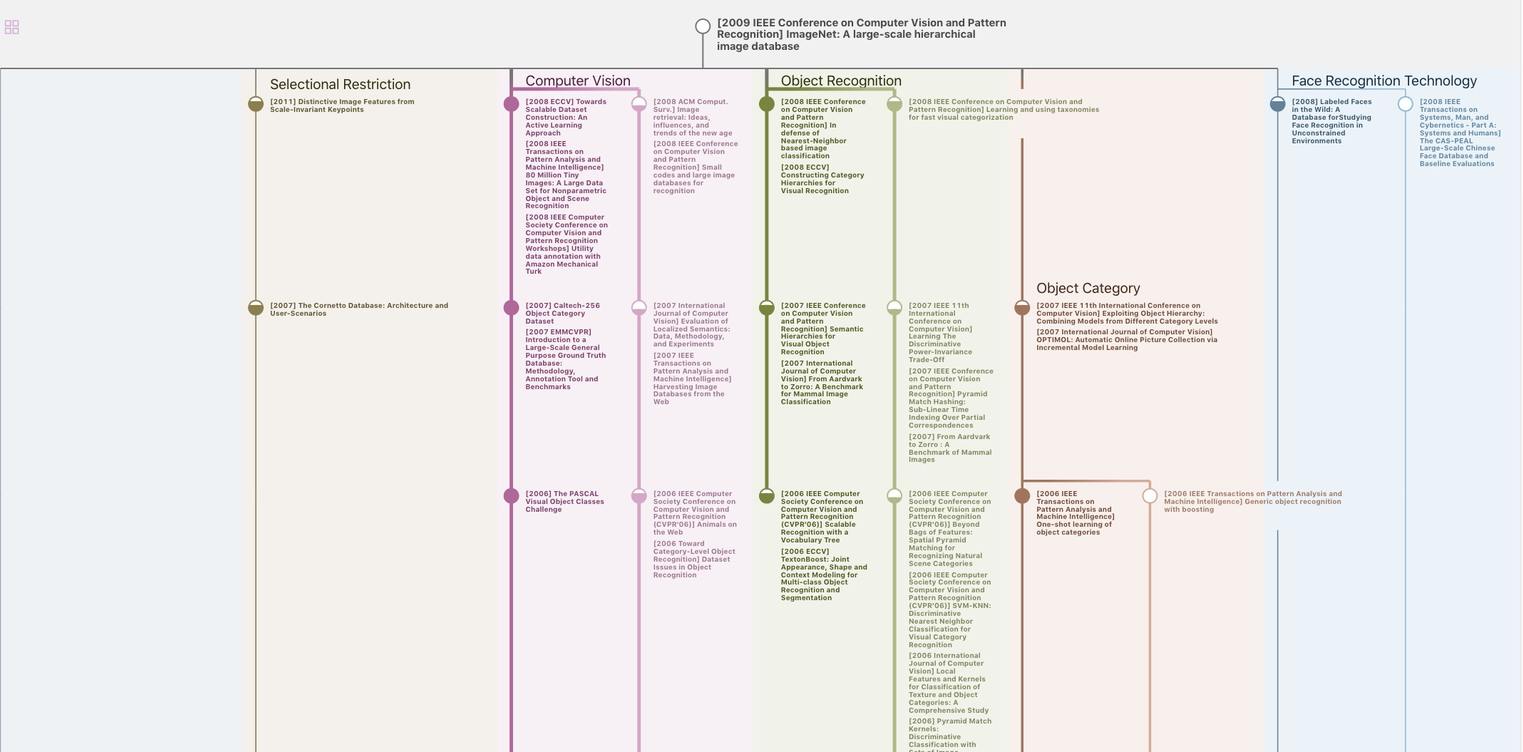
生成溯源树,研究论文发展脉络
Chat Paper
正在生成论文摘要