On bonus-based exploration methods
semanticscholar(2020)
摘要
Research on exploration in reinforcement learning, as applied to Atari 2600 gameplaying, has emphasized tackling difficult exploration problems such as MONTEZUMA’S REVENGE (Bellemare et al., 2016). Recently, bonus-based exploration methods, which explore by augmenting the environment reward, have reached above-human average performance on such domains. In this paper we reassess popular bonus-based exploration methods within a common evaluation framework. We combine Rainbow (Hessel et al., 2018) with different exploration bonuses and evaluate its performance on MONTEZUMA’S REVENGE, Bellemare et al.’s set of hard of exploration games with sparse rewards, and the whole Atari 2600 suite. We find that while exploration bonuses lead to higher score on MONTEZUMA’S REVENGE they do not provide meaningful gains over the simpler -greedy scheme. In fact, we find that methods that perform best on that game often underperform -greedy on easy exploration Atari 2600 games. We find that our conclusions remain valid even when hyperparameters are tuned for these easy-exploration games. Finally, we find that none of the methods surveyed benefit from additional training samples (1 billion frames, versus Rainbow’s 200 million) on Bellemare et al.’s hard exploration games. Our results suggest that recent gains in MONTEZUMA’S REVENGE may be better attributed to architecture change, rather than better exploration schemes; and that the real pace of progress in exploration research for Atari 2600 games may have been obfuscated by good results on a single domain.
更多查看译文
AI 理解论文
溯源树
样例
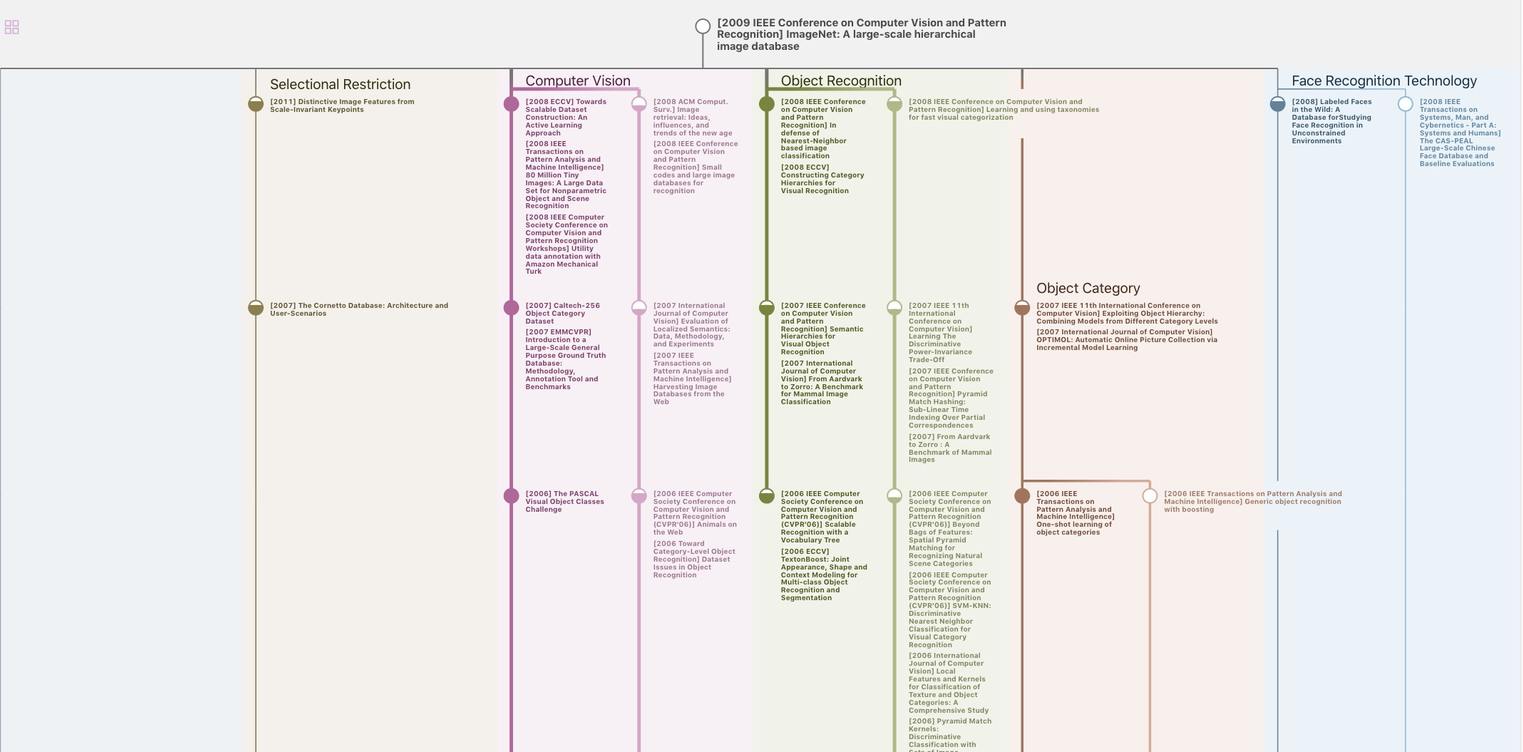
生成溯源树,研究论文发展脉络
Chat Paper
正在生成论文摘要