Periodic Stepsize Adaptation
semanticscholar(2008)
摘要
Previously, Bottou and LeCun [1] established that the secon d-order stochastic gradient descent (SGD) method can potentially achieve generalization perfo rmance as well as empirical optimum in a single pass through the training examples. However, sec ond-order SGD requires computing the inverse of the Hessian matrix of the loss function, which is usually prohibitively expensive. Recently, we invented a new second-order SGD method, called Periodic Stepsize Adaptation (PSA). PSA explores a simple linear relation between the Hessian ma trix and the Jacobian matrix of the mapping function. Instead of approximating Hessian, PSA ap proximates the Jacobian matrix which is proved to be simpler and more effective than approximatin g Hessian in an on-line setting. Experimental results for conditional random fields (CRF) and neura l networks (NN) show that single-pass performance of PSA is very close to empirical optimum.
更多查看译文
AI 理解论文
溯源树
样例
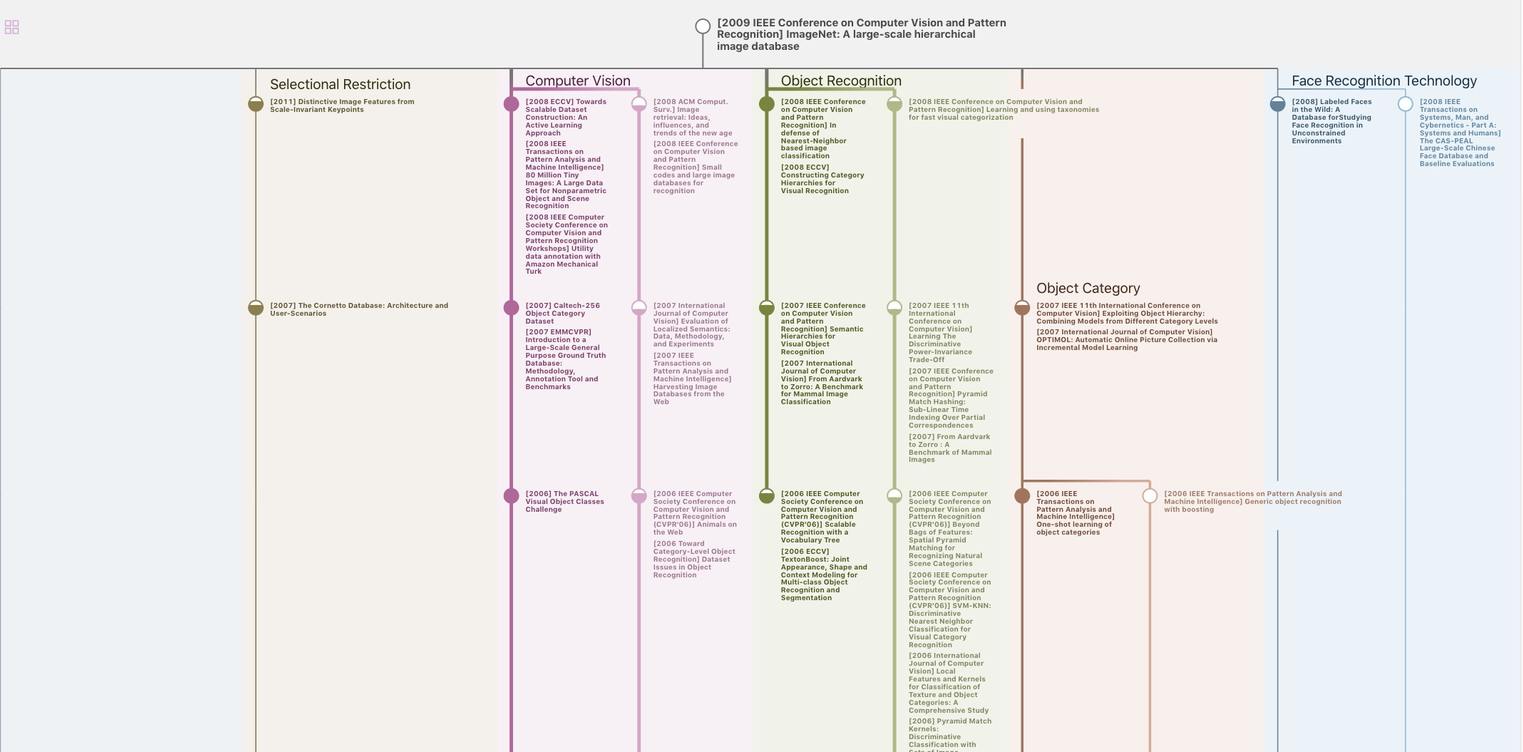
生成溯源树,研究论文发展脉络
Chat Paper
正在生成论文摘要