Leveraging Symbolic Planning Models in Hierarchical Reinforcement Learning
semanticscholar(2019)
摘要
We investigate the use of explicit action models—as typically used for Automated Planning—in the context of Reinforcement Learning (RL). These action models allow agents to reason about macro-actions and high-level symbolic state spaces. As a consequence, agents with access to an action model and a planner can automatically synthesize high-level plans that can, in turn, be used as high-level instructions to significantly improve sample efficiency. Our approach is based on classical and partial-order planning, in combination with hierarchical RL and recent advances in reward specification and problem decomposition for RL. Empirical results show that our approach finds high-quality policies for previously unseen tasks in extremely few training steps, consistently outperforming standard Hierarchical RL techniques.
更多查看译文
AI 理解论文
溯源树
样例
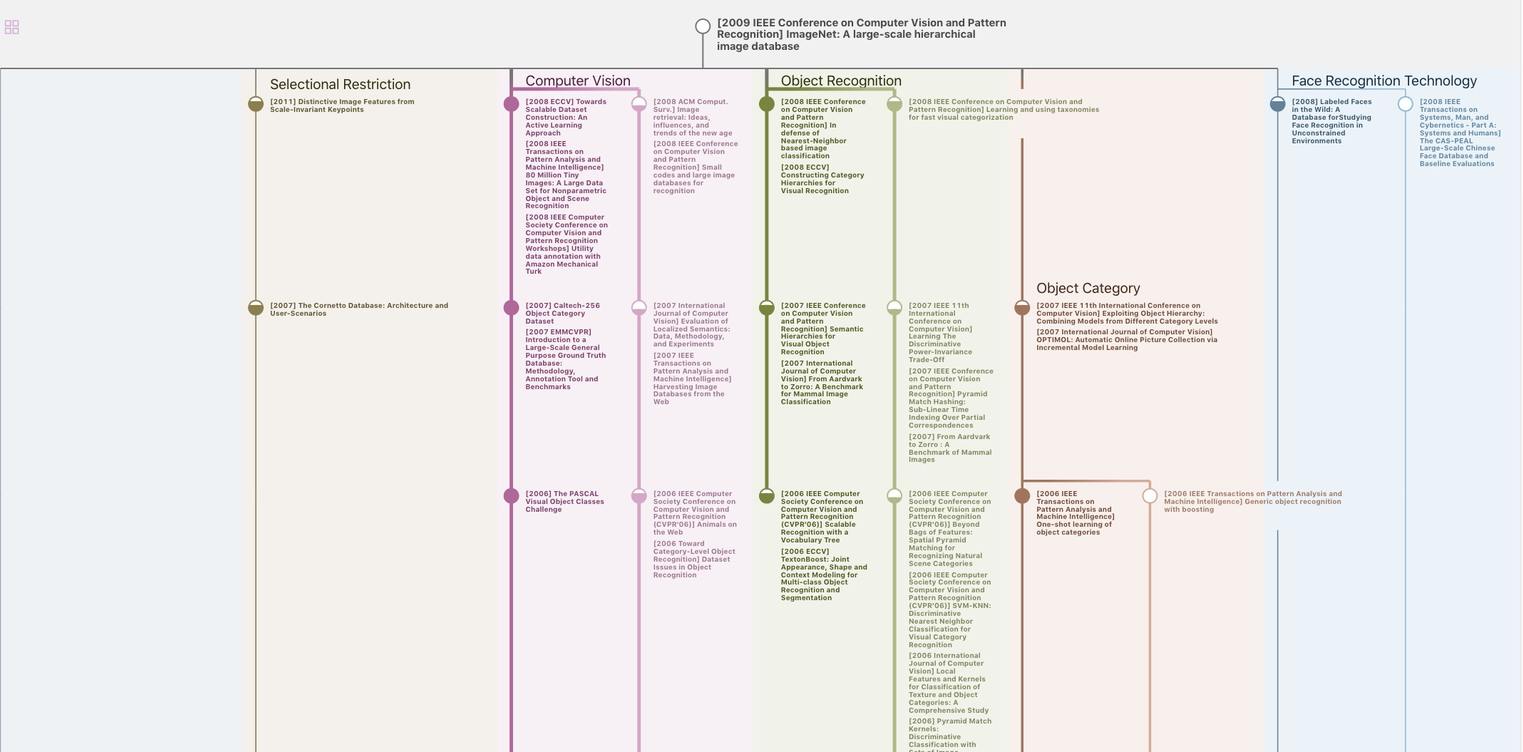
生成溯源树,研究论文发展脉络
Chat Paper
正在生成论文摘要