Word Vector Model Descriptions In Depth :
semanticscholar
摘要
: Many state of the art NLP algorithms currently use deep learning techniques. A 2014 paper by Goodfellow et al demonstrates that, at least in image classification, error rates from deep learning can be improved by adversarial training. Generating adversarial examples relies on having highdimensional input spaces and many output labels. We present two methods of generating adversarial examples, and also introduce a new loss function for training word vectors in a CBOW model. Using QVEC as a metric for our word vectors, these techniques both improve on the vanilla CBOW model, and these methods can be used in conjunction with other generalization techniques such as dropout and early stopping.
更多查看译文
AI 理解论文
溯源树
样例
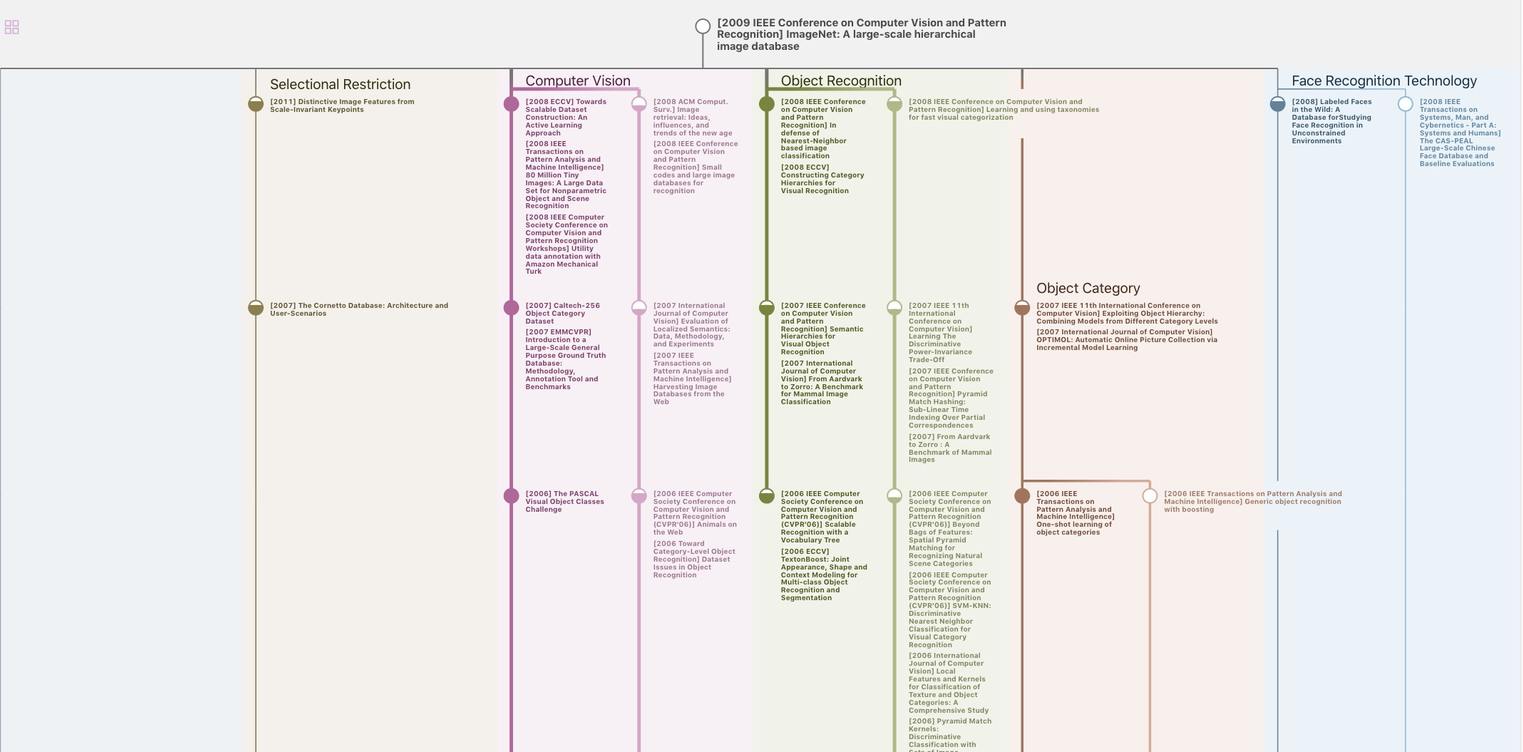
生成溯源树,研究论文发展脉络
Chat Paper
正在生成论文摘要