Sparse Estimation: An MMSE Approach
CONSTRUCTIVE APPROXIMATION(2023)
摘要
The objective of this paper is to estimate parameters with a sparse prior via the minimum mean square error (MMSE) approach. We model the sparsity by the Bernoulli-uniform prior. The MMSE estimator gives the posterior mean of the parameter to be estimated. However, its computation involves multiple integrations of many variables that is hard to implement numerically. In order to overcome this difficulty, we develop a coordinate minimization algorithm to approximate the MMSE estimator for any arbitrary given prior. We connect this algorithm to a variational model and establish a comprehensive convergence analysis. The algorithm converges to a special stationary point of the variational model, which attains the minimum of the mean square error at each coordinate when others are fixed. Then, this general algorithm is applied to the Bernoulli-uniform sparse prior and leads to a stable estimator that provides a good balance between sparsity and unbiasedness. The advantages of our sparsity model and algorithm over other approaches (e.g., the maximum a posteriori approaches) are analysed in detail and further demonstrated by numerical simulations. The applications of the general theory and algorithm developed here go beyond sparse estimation.
更多查看译文
关键词
Minimum mean square error,Bayesian method,Coordinate minimization,Variational model,Nonconvex optimization
AI 理解论文
溯源树
样例
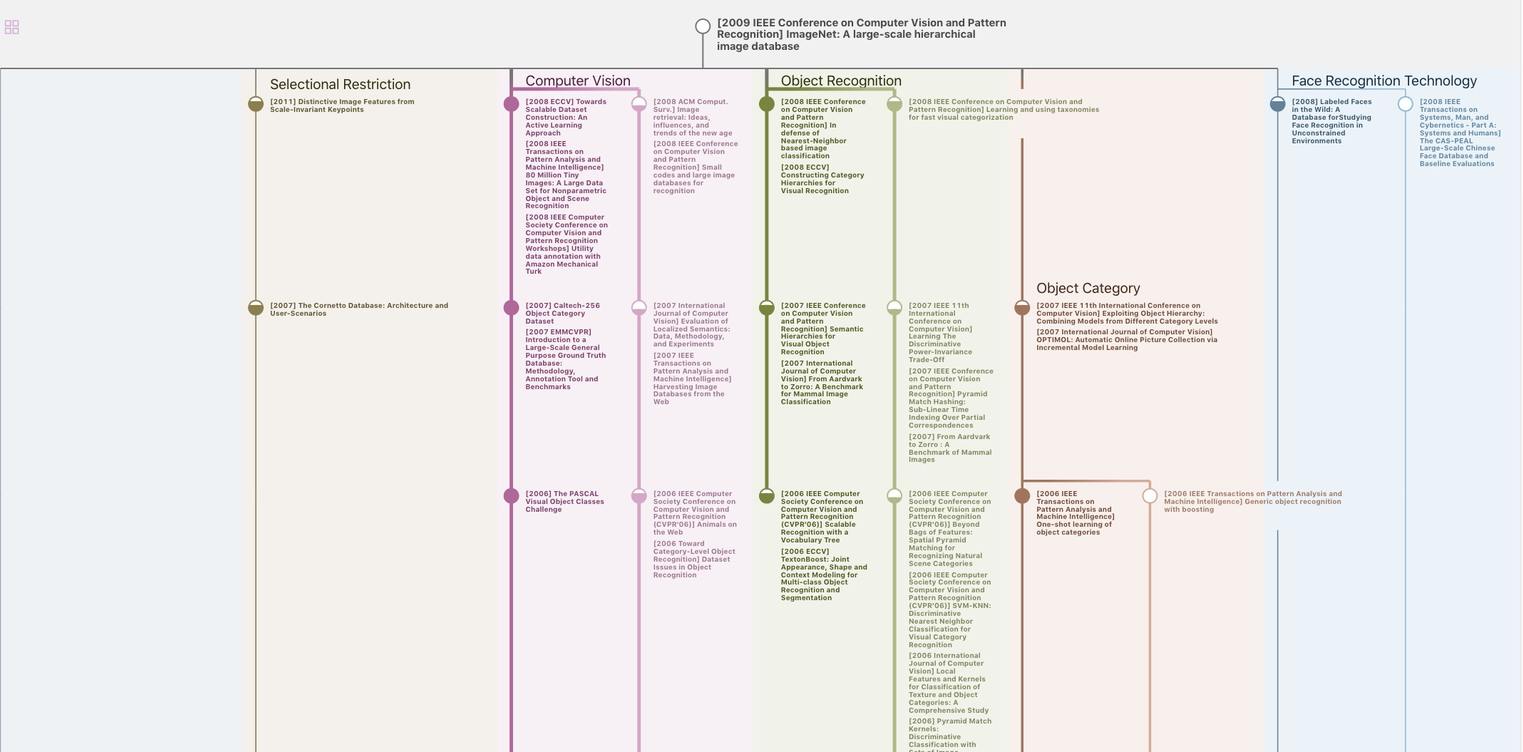
生成溯源树,研究论文发展脉络
Chat Paper
正在生成论文摘要