Office Appliance Classification From Disaggregated Plug-Load Data
semanticscholar(2014)
摘要
As buildings consume 40% of US primary energy, energy efficiency through smart plug-load management can have a significant impact on US carbon emissions. In this paper we investigate learning algorithms to classify plug-loads based on minutely energy plug-load data and hourly weather data. One month of data is preprocessed into 31 daily features which are used to classify 5660 training samples into 31 labels using a range of multi-class classification algorithms. Of all models tested, random forest trees performed the best, with a test error of 17%. This promising result can likely be further refined with improved feature selection, and a larger dataset. Future work will focus on increasing the model performance and integrating it into a useful product for plug-load management companies.
更多查看译文
AI 理解论文
溯源树
样例
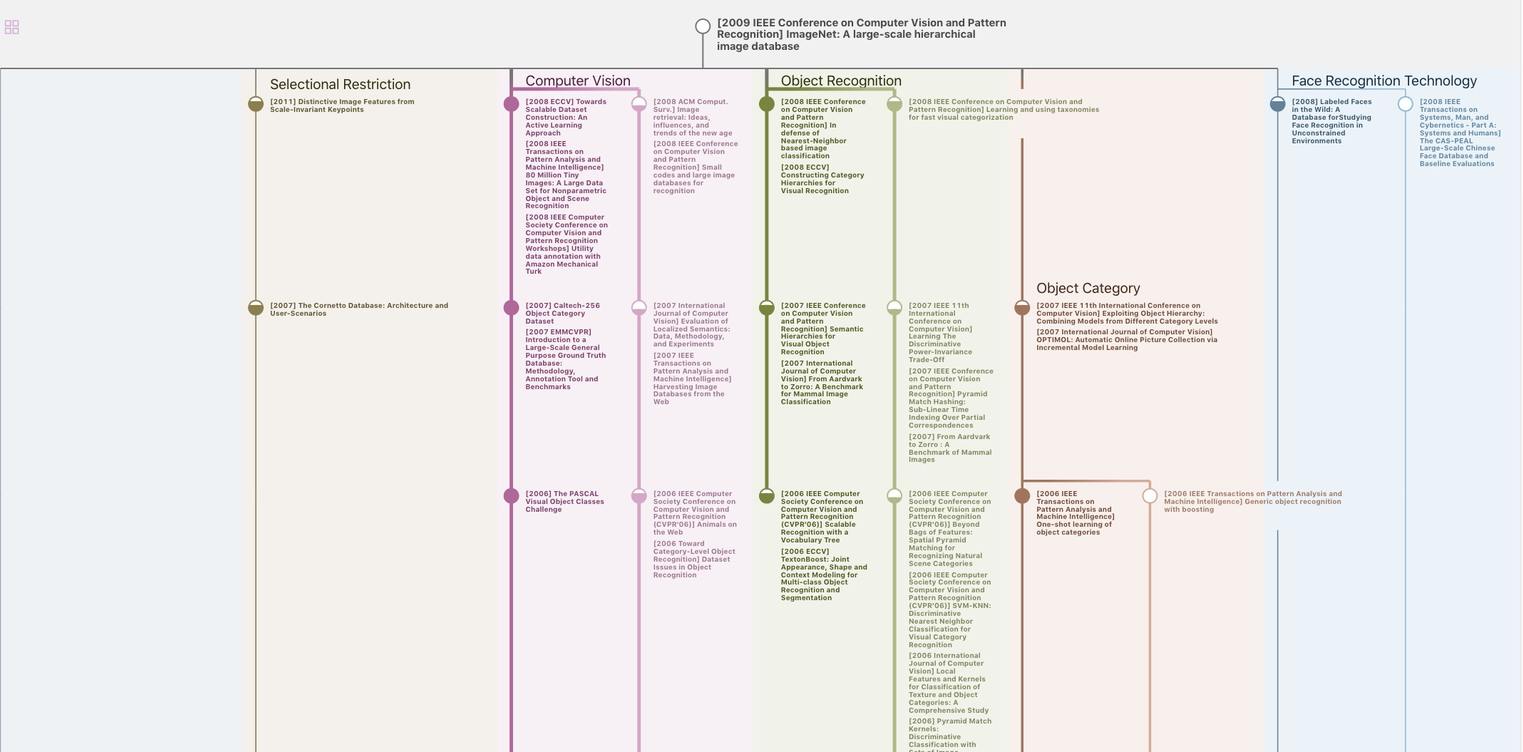
生成溯源树,研究论文发展脉络
Chat Paper
正在生成论文摘要