Identifying and Prioritizing Fire Inspections : A Case Study of Predicting Fire Risk in Atlanta
semanticscholar(2015)
摘要
The Atlanta Fire Rescue Department (AFRD) attempts to reduce fire risk by inspecting buildings for potential hazards and fire code violations. This paper provides a case study exemplifying how data science can be used to help cities identify and prioritize potential property inspections, using machine learning, geocoding, and information visualization. As a result of this work, we generated a risk score for 5,000 buildings in the city, with an average of 73% accuracy for predicting future fires. We also identified 19,397 new potential properties to inspect, based on AFRD criteria, and developed an interactive map to allow AFRD staff to make informed decisions about fire inspections. The results of this study hold great promise for helping cities use data–driven techniques to make civic processes more efficient.
更多查看译文
AI 理解论文
溯源树
样例
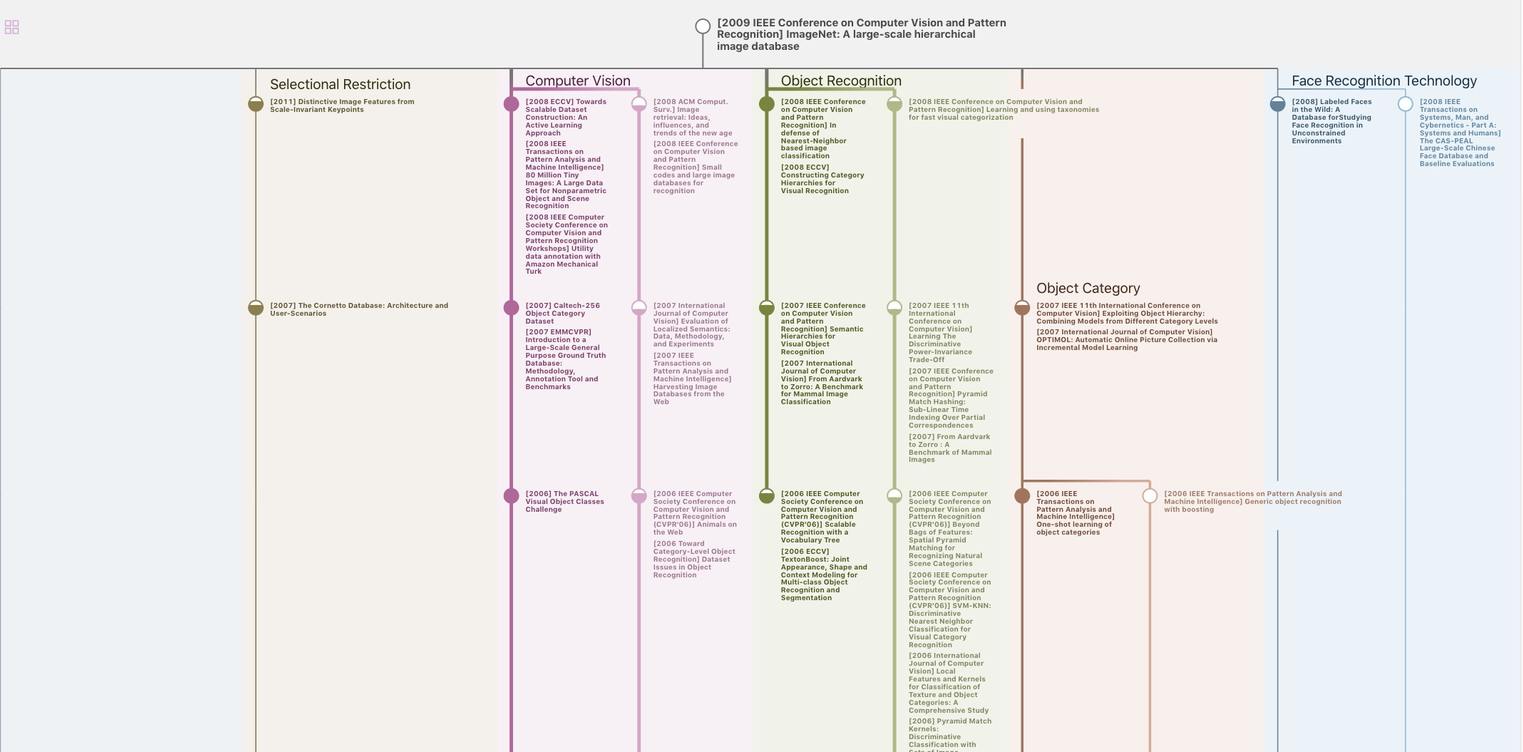
生成溯源树,研究论文发展脉络
Chat Paper
正在生成论文摘要