Unsurpervised Learning in Hybrid Cognitive Architectures
NeSy@AAAI(2012)
摘要
We present a model of unsupervised learning in the hybrid SAL (Synthesis of ACT-R and Leabra) architecture. This model follows the hypothesis that higher evaluative cognitive mechanisms can serve to provide training signals for perceptual learning. This addresses the problem that supervised learning seems necessary for strong perceptual performance, but explicit feedback is rare in the real world and difficult to provide for artificial learning systems. The hybrid model couples the perceptual strengths of Leabra with ACT-R’s cognitive mechanisms, specifically its declarative memory, to evolve its own symbolic representations of objects encountered in the world. This is accomplished by presenting the objects to the Leabra visual system and committing the resulting representation to ACT-R’s declarative memory. Subsequent presentations are either recalled as instances of a previous object category, in which case the positive association with the representation is rehearsed by Leabra, or they cause ACTR to generate new category labels, which are also subject to the same rehearsal. The rehearsals drive the network’s representations to convergence for a given category; at the same time, rehearsals on the ACT-R side reinforce the chunks that encode the associations between representation and label. In this way, the hybrid model bootstraps itself into learning new categories and their associated features; this framework provides a potential approach to solving the symbol grounding problem. We outline the operations of the hybrid model, evaluate its performance on the CU3D-100 (cu3d.colorado.edu) image set, and discuss further potential improvements to the model, including the integration of motor functions as a way of providing an internal feedback signal to augment and guide a purely bottom-up unsupervised
更多查看译文
AI 理解论文
溯源树
样例
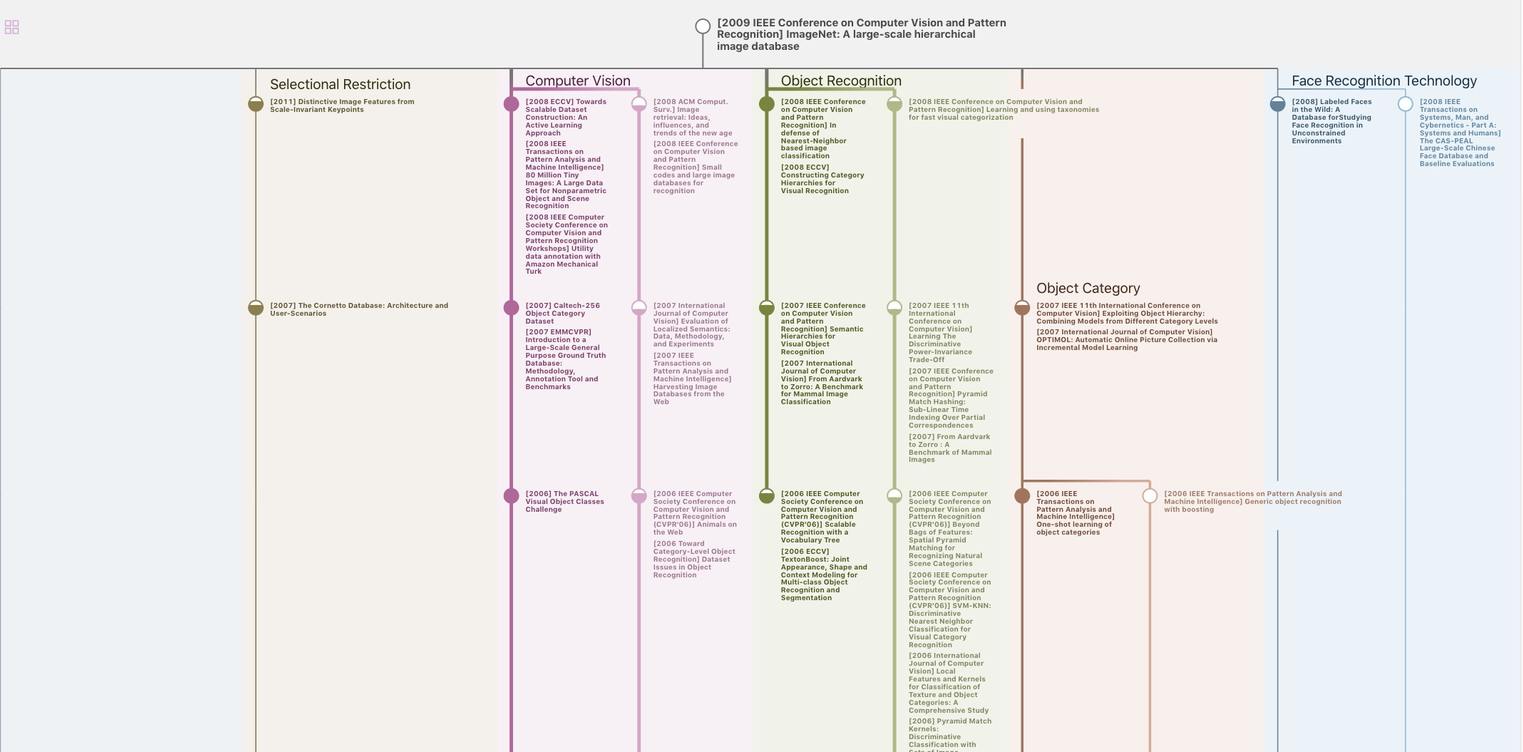
生成溯源树,研究论文发展脉络
Chat Paper
正在生成论文摘要