Bayesian Learning of Non-Negative Matrix / Tensor Factorizations by Simulating Pólya Urns
semanticscholar(2018)
摘要
We introduce a dynamic generative model, Bayesian Allocation Model (BAM) that makes the connection among nonnegative tensor decompositions, topic models, and Bayesian networks explicit. Our construction is based on marking the events of a Poisson process using a Bayesian network and integrating out the parameters analytically where the resulting marginal process is a Pólya urn. The marginal likelihood can then be characterized as the probability that an urn process hits a certain small set. This alternative random process perspective allows us to develop sequential Monte Carlo (SMC) algorithms for computing nonnegative tensor decompositions and the marginal likelihood whose computational complexity is independent from the tensor dimensions. Our analysis and simulation results suggest that the SMC algorithms have favorable properties in the sparse data regime, but our construction also provides additional justification for the popular variational algorithms in the dense/large data limits.
更多查看译文
AI 理解论文
溯源树
样例
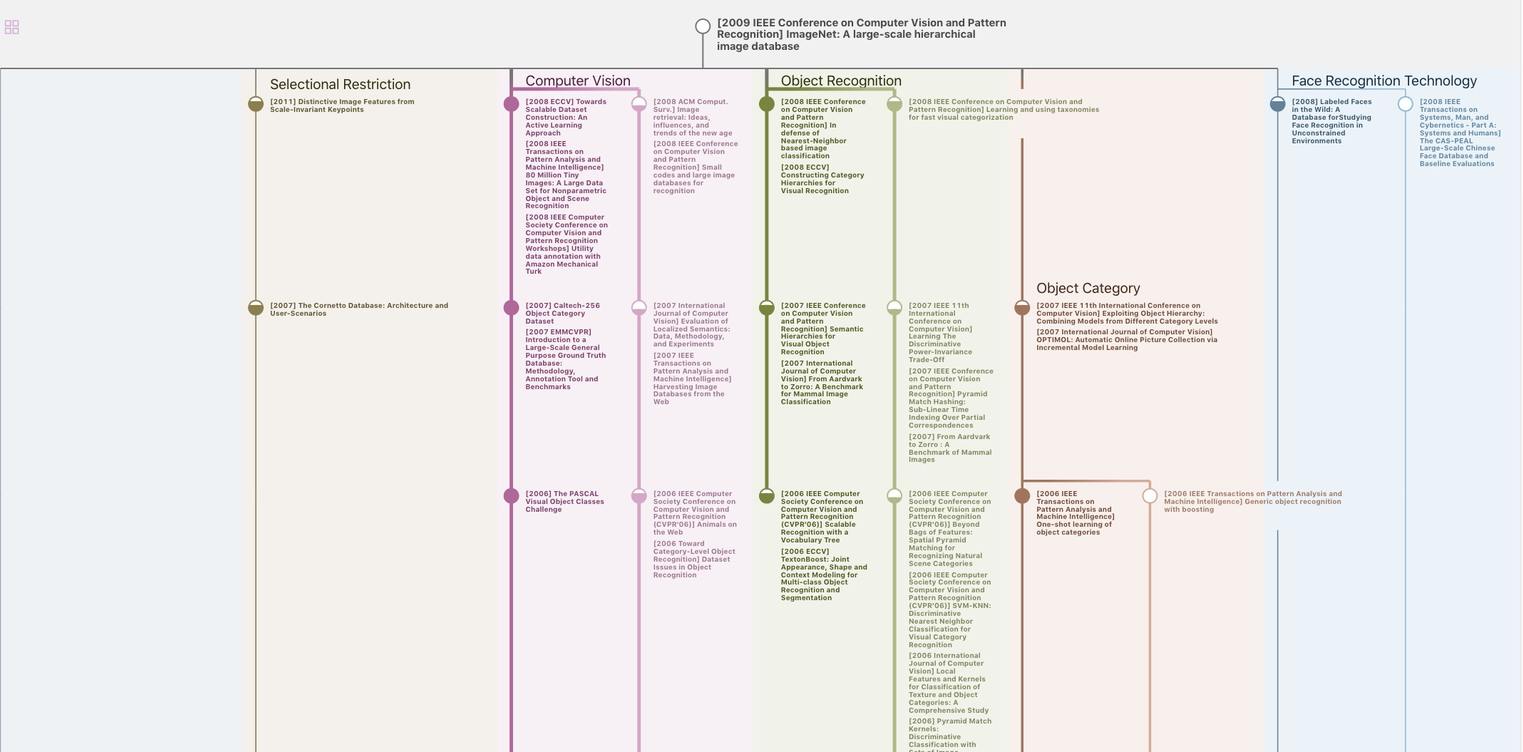
生成溯源树,研究论文发展脉络
Chat Paper
正在生成论文摘要