Symbolic Planning and Model-Free Reinforcement Learning: Training Taskable Agents
semanticscholar(2019)
摘要
We investigate the use of explicit symbolic action models, as typically used for Automated Planning, in the context of Reinforcement Learning (RL). Our objective is to make RL agents more sample efficient and human taskable. We say an agent is taskable when it is capable of achieving a variety of different goals and there is a simple method for goal specification. Moreover, we expect taskable agents to easily transfer skills learned for one task to other related tasks. To these ends, we consider high-level models that inexactly represent the low-level environment in which an agent acts. Given a model, defining goal-directed tasks is a simple problem, and we show how to communicate these goals to an agent by leveraging state-of-the-art symbolic planning techniques. We automatically generate families of highlevel solutions and subsequently represent them as a reward machine, a recently introduced formalism for describing structured reward functions. In doing this, we not only specify what the task at hand is, but also give a high-level description of how to achieve it. The structure present in this description can be successfully exploited by a Hierarchical RL system. The reward machine represents a collection of sequential solutions and can be used to prune the options available when training. We can ensure that, at every step, the meta-controller can only select options that represent advancement in some high-level plan. We empirically demonstrate the merits of our approach, comparing to a naive baseline where a single sequential plan is strictly followed, and to standard Hierarchical RL techniques. Our results show that the approach is an effective method for specifying tasks to an RL agent. Given adequately pretrained options, our approach reaches high-quality policies in previously unseen tasks in extremely few training steps and consistently outperforms the standard techniques.
更多查看译文
AI 理解论文
溯源树
样例
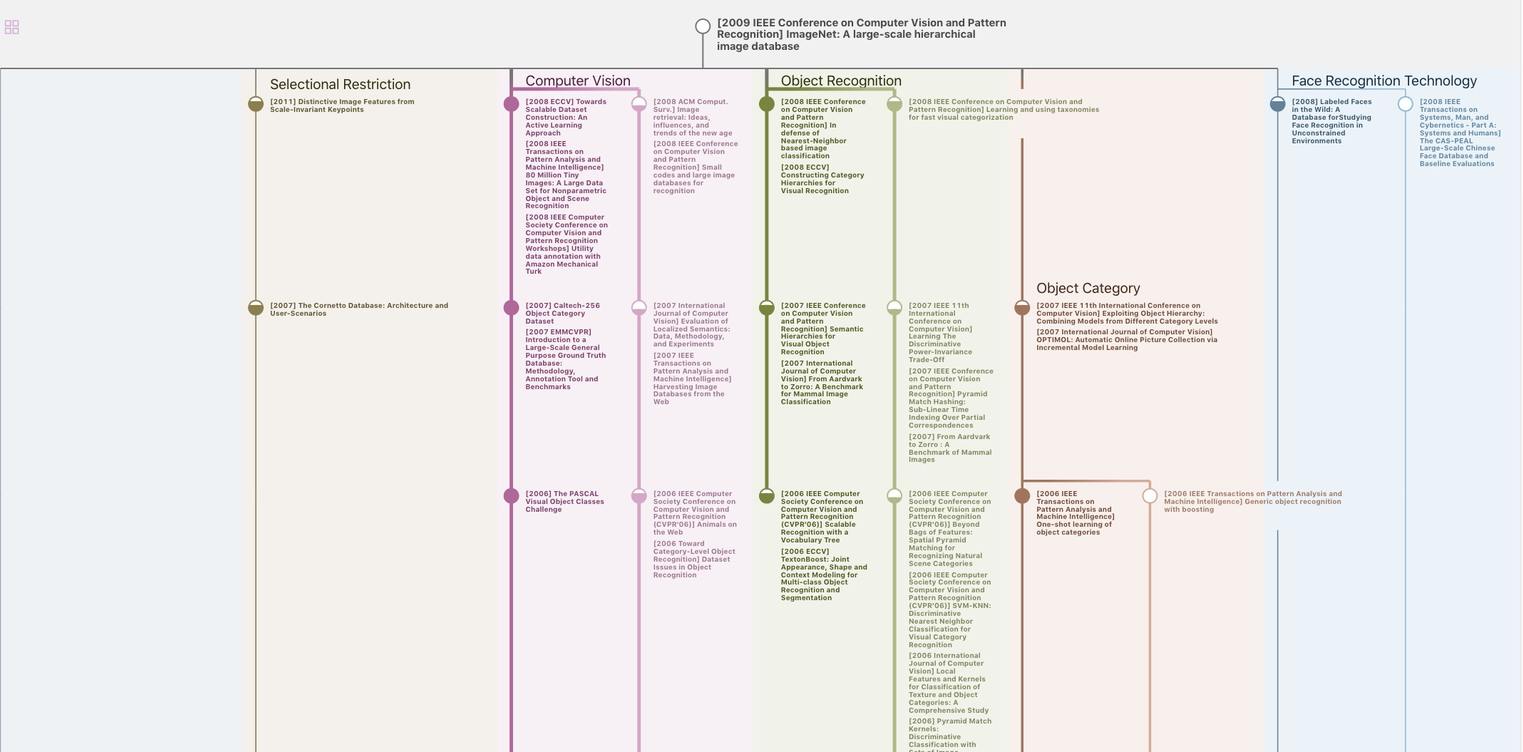
生成溯源树,研究论文发展脉络
Chat Paper
正在生成论文摘要