Deep Contextual Clinical Prediction with Reverse Distillation
THIRTY-FIFTH AAAI CONFERENCE ON ARTIFICIAL INTELLIGENCE, THIRTY-THIRD CONFERENCE ON INNOVATIVE APPLICATIONS OF ARTIFICIAL INTELLIGENCE AND THE ELEVENTH SYMPOSIUM ON EDUCATIONAL ADVANCES IN ARTIFICIAL INTELLIGENCE(2021)
摘要
Healthcare providers are increasingly using machine learning to predict patient outcomes to make meaningful interventions. However, despite innovations in this area, deep learning models often struggle to match performance of shallow linear models in predicting these outcomes, making it difficult to leverage such techniques in practice. In this work, motivated by the task of clinical prediction from insurance claims, we present a new technique called reverse distillation which pretrains deep models by using high-performing linear models for initialization. We make use of the longitudinal structure of insurance claims datasets to develop Self Attention with Reverse Distillation, or SARD, an architecture that utilizes a combination of contextual embedding, temporal embedding and self-attention mechanisms and most critically is trained via reverse distillation. SARD outperforms state-of-the-art methods on multiple clinical prediction outcomes, with ablation studies revealing that reverse distillation is a primary driver of these improvements. Code is available at https://github.com/clinicalml/omop-learn.
更多查看译文
关键词
deep contextual clinical prediction
AI 理解论文
溯源树
样例
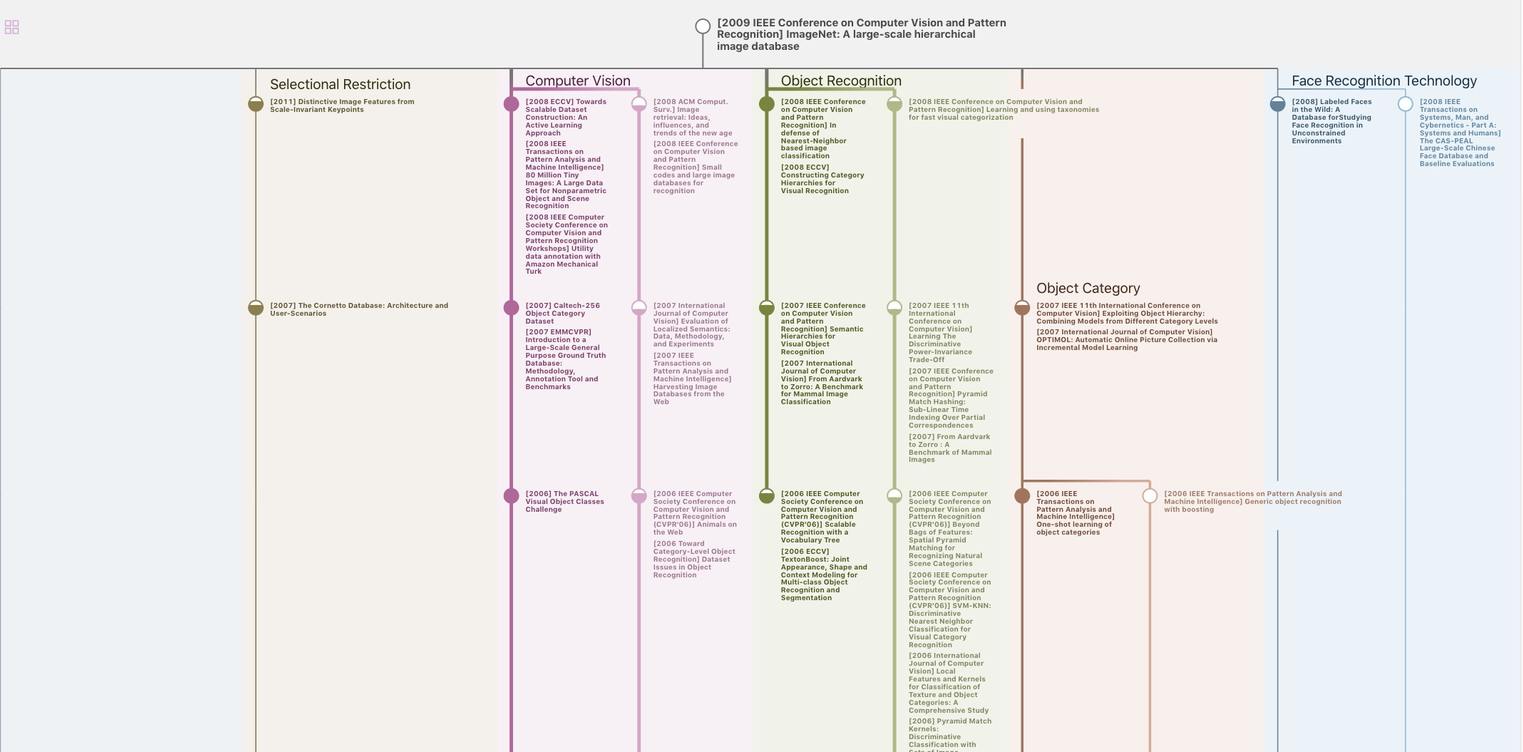
生成溯源树,研究论文发展脉络
Chat Paper
正在生成论文摘要