Survival neural networks for time-to-event prediction in longitudinal study
KNOWLEDGE AND INFORMATION SYSTEMS(2020)
摘要
Time-to-event prediction has been an important practical task for longitudinal studies in many fields such as manufacturing, medicine, and healthcare. While most of the conventional survival analysis approaches suffer from the presence of censored failures and statistically circumscribed assumptions, few attempts have been made to develop survival learning machines that explore the underlying relationship between repeated measures of covariates and failure-free survival probability. This requires a purely dynamic-data-driven prediction approach, free of survival models or statistical assumptions. To this end, we propose two real-time survival networks: a time-dependent survival neural network (TSNN) with a feed-forward architecture and a recurrent survival neural network (RSNN) incorporating long short-term memory units. The TSNN additively estimates a latent failure risk arising from the repeated measures and performs multiple binary classifications to generate prognostics of survival probability, while the RSNN with time-dependent input covariates implicitly estimates the relation between these covariates and the survival probability. We propose a novel survival learning criterion to train the neural networks by minimizing the censoring Kullback–Leibler divergence, which guarantees monotonicity of the resulting probability. Besides the failure-event AUC, C-index, and censoring Brier score, we redefine a survival time estimate to evaluate the performance of the competing models. Experiments on four datasets demonstrate the great promise of our approach in real applications.
更多查看译文
关键词
Time-to-event prediction,Longitudinal study,Survival neural network,Classification probability,Time-dependent covariate
AI 理解论文
溯源树
样例
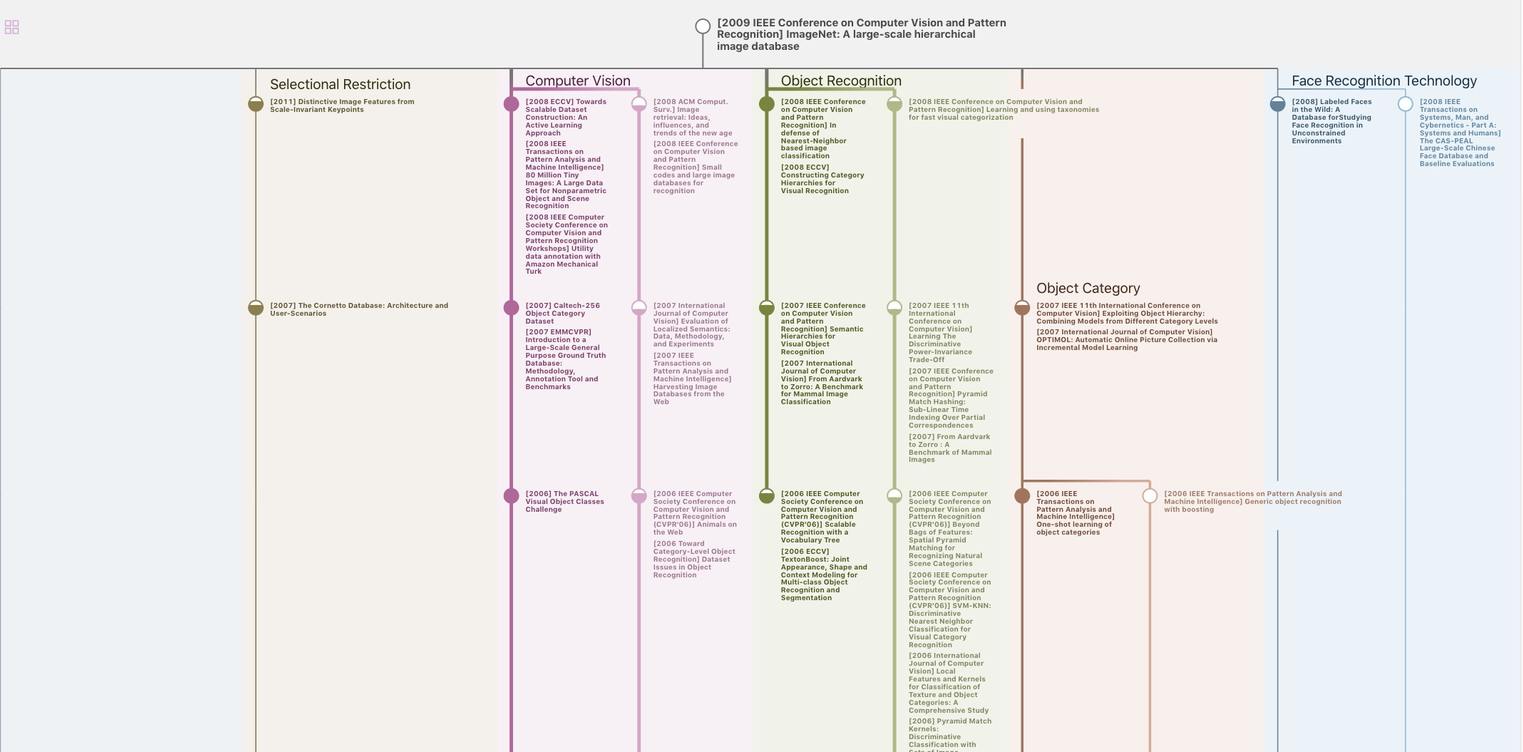
生成溯源树,研究论文发展脉络
Chat Paper
正在生成论文摘要