Robust Machine Learning Algorithms for Text Analysis∗
semanticscholar(2019)
摘要
We study the Latent Dirichlet Allocation model, a popular Bayesian algorithm for text analysis. Our starting point is the generic lack of identification of the model’s parameters, which suggests that the choice of prior matters. We then characterize by how much the posterior mean of a given functional of the model’s parameters varies in response to a change in the prior, and we suggest two algorithms to approximate this range. Both of our algorithms rely on obtaining multiple Nonnegative Matrix Factorizations of either the posterior draws of the corpus’ population term-document frequency matrix or of its sample analogue. The key idea is to maximize/minimize the functional of interest over all these nonnegative matrix factorizations. To illustrate the applicability of our results, we revisit recent work on the effects of increased transparency on discussions regarding monetary policy decisions in the United States.
更多查看译文
AI 理解论文
溯源树
样例
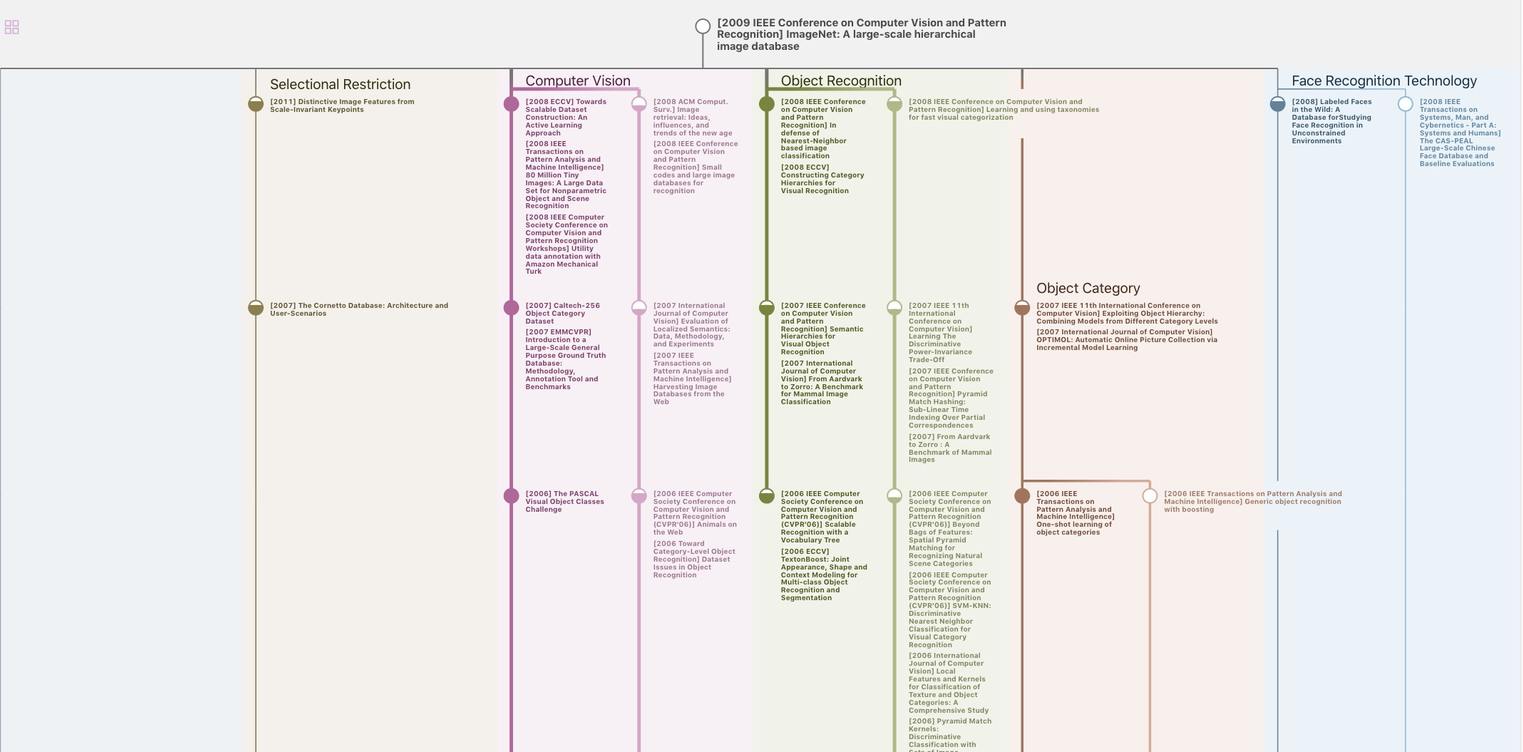
生成溯源树,研究论文发展脉络
Chat Paper
正在生成论文摘要