SNP-based soybean variety selection
semanticscholar(2017)
摘要
One of the main tools in soybean variety selection is analyzing data from yield testing. While most of the tested varieties never come to commercialization, some commercialized varieties are tested in the small number of testing fields. Current Marker-assisted selection (MAS) strategies are focused on major quantitative trait locus (QTL), whereas yield has the complex inheritance that is controlled by multiple QTL with minor effect on phenotype [14]. With the machine learning, yield potential can be efficiently predicted using high dimensional single nucleotide polymorphisms (SNP) markers of varieties. Therefore predicted yield potential could enable better allocation of varieties in testing fields, reducing the number of commercialization of unsuccessful varieties and reducing costs of production. This paper will examine data from Syngenta AI Challenge [4, 9] and the effectiveness of Gradient Boosted Regression Trees for predicting yield potential from SNP markers.
更多查看译文
AI 理解论文
溯源树
样例
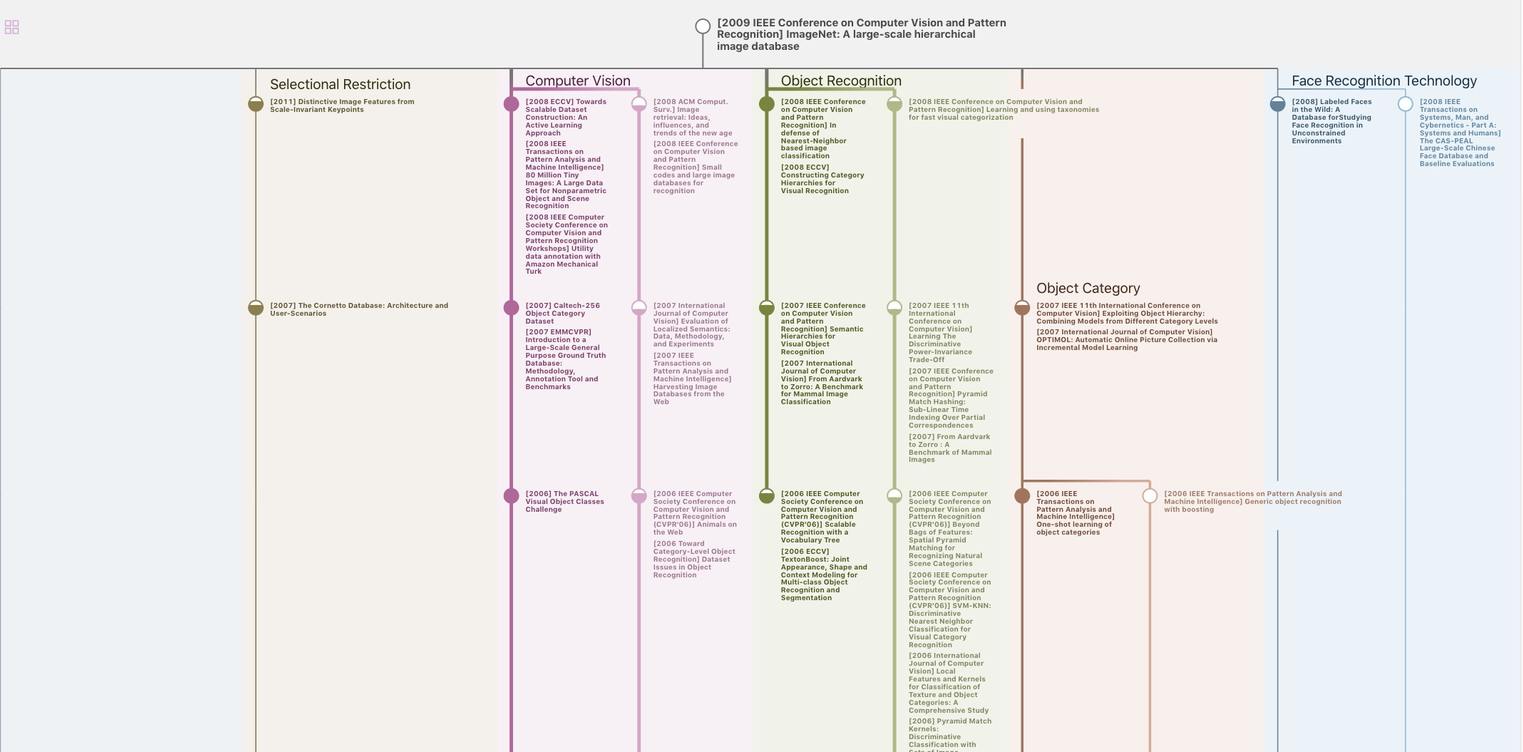
生成溯源树,研究论文发展脉络
Chat Paper
正在生成论文摘要