Online Learning for Multi-Agent Local Navigation
semanticscholar(2013)
摘要
In this paper, we present an adaptive framework for improving the local navigation in multi-agent simulations. Our framework uses techniques from Reinforcement Learning allowing the agents to adapt their behavior online while the simulation is still running. It is general and can be easily combined with existing schemes for local navigation. As an example, we demonstrate its application using the Optimal Reciprocal Collision Avoidance method introduced in robotics. Preliminary results show that, using our adaptive approach, the agents exhibit a more polite and respectful behavior toward each other, as compared to approaches that do not use learning. This alleviates congestion phenomena observed in non-adaptive local navigation methods and helps the agents reach their goal destinations faster.
更多查看译文
AI 理解论文
溯源树
样例
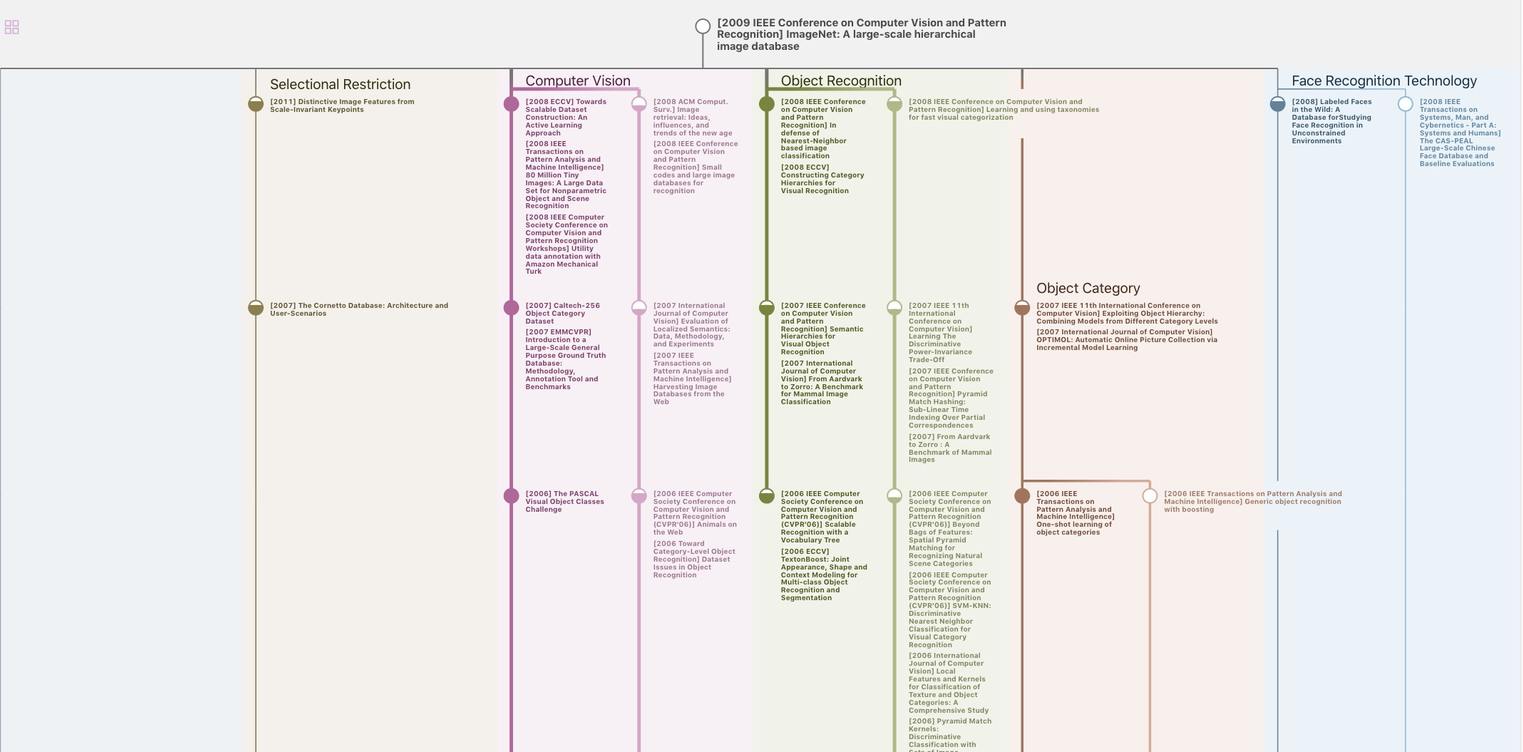
生成溯源树,研究论文发展脉络
Chat Paper
正在生成论文摘要