Using POMDPs to Learn Language in a Spatial Reference Game
semanticscholar(2018)
摘要
Much of early human language learning takes place in an unsupervised setting. In this work, we investigate how autonomous agents can use goal-oriented tasks in a spatial reference game to learn language. This problem is made difficult by the high dimensionality of the state and action spaces as well as the fact that it relates achieving one objective (i.e. reaching a goal) to achieving a secondary one (i.e. learning directional language). We formalize this problem as a Markov decision process (MDP) and partially observable Markov decision processes (POMDPs). We analyze the performance of the agent under different conditions using dynamic programming and online POMDP solution techniques. We perform and visualize simulations of the policies and real-time update of belief states. We observe that knowing the language can influence the time it takes to arrive at a goal state, and completely learning the language can be incentivized by explicitly optimizing for that task.
更多查看译文
AI 理解论文
溯源树
样例
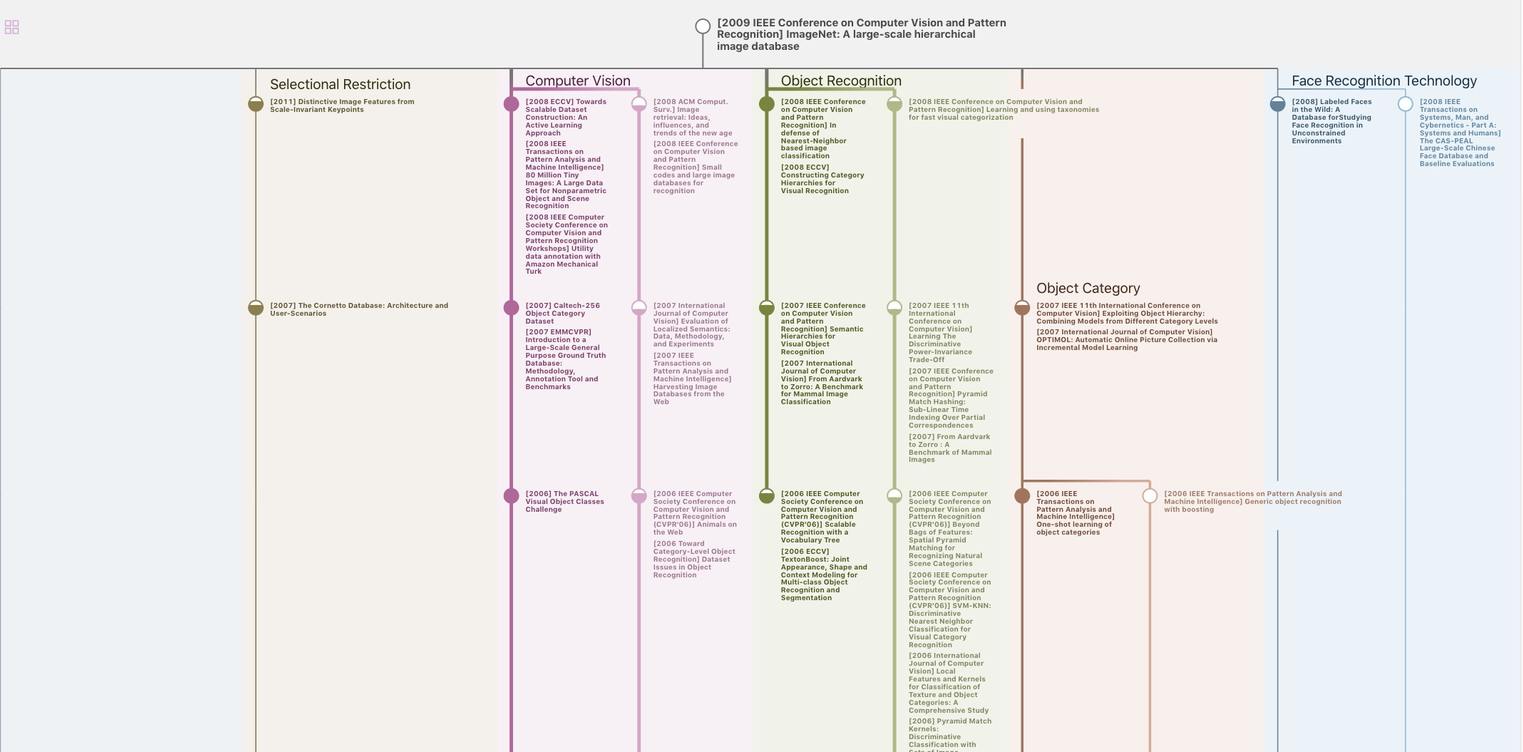
生成溯源树,研究论文发展脉络
Chat Paper
正在生成论文摘要