Solving ODE with Universal Flows: Approximation Theory for Flow-Based Models
international conference on learning representations(2020)
摘要
Normalizing flows are powerful invertible probabilistic models that can be used to translate two probability distributions, in a way that allows us to efficiently track the change of probability density. However, to trade for computational efficiency in sampling and in evaluating the log-density, special parameterization designs have been proposed at the cost of representational expressiveness. In this work, we propose to use ODEs as a framework to establish universal approximation theory for certain families of flow-based models.
更多查看译文
AI 理解论文
溯源树
样例
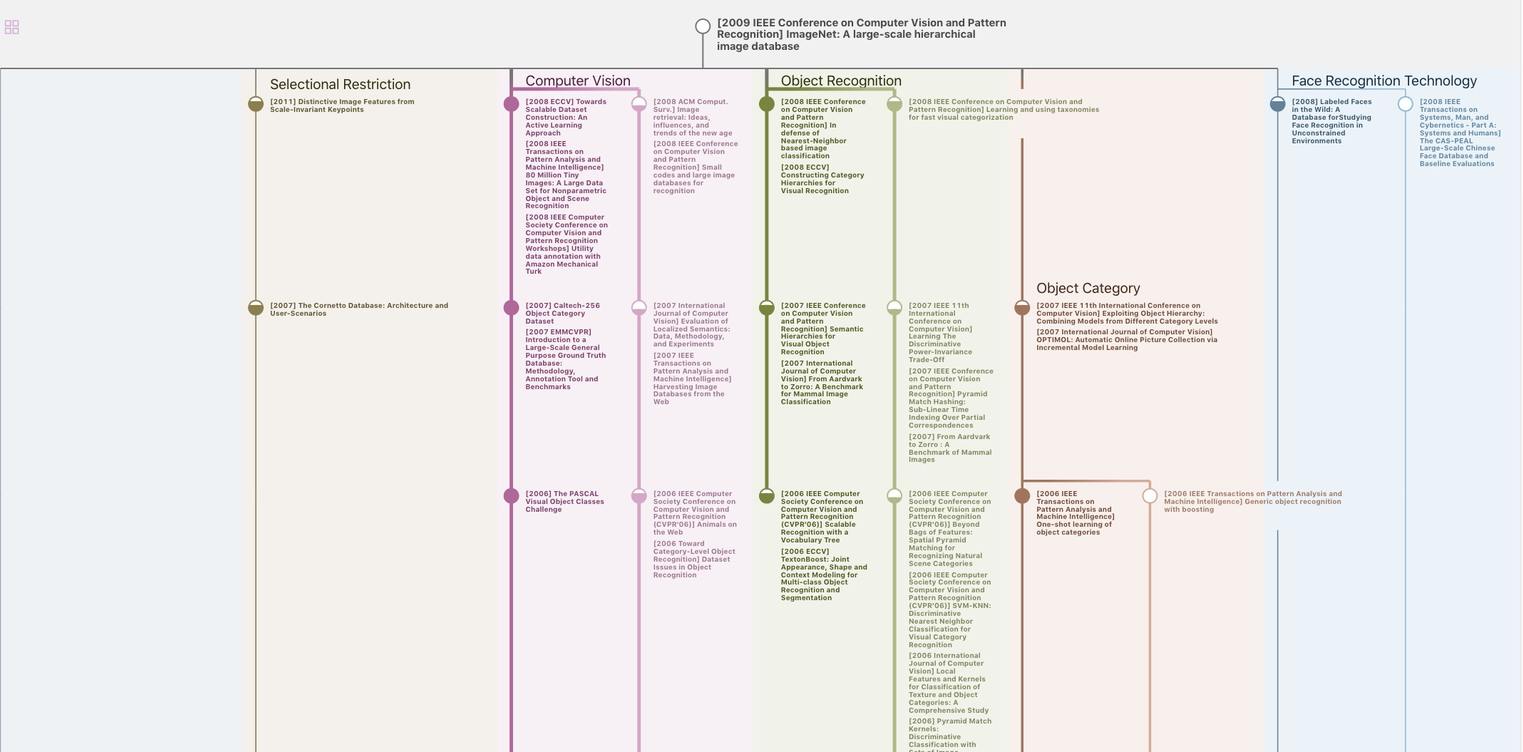
生成溯源树,研究论文发展脉络
Chat Paper
正在生成论文摘要