Cross-Regional Oil Palm Tree Detection
2020 IEEE/CVF Conference on Computer Vision and Pattern Recognition Workshops (CVPRW)(2020)
摘要
As oil palm has become one of the most rapidly expanding tropical crops in the world, detecting and counting oil palms have received considerable attention. Although deep learning has been widely applied to remote sensing image processing including tree crown detection, the large size and the variety of the data make it extremely difficult for cross-regional and large-scale scenarios. In this paper, we propose a cross-regional oil palm tree detection (CROPTD) method. CROPTD contains a local domain discriminator and a global domain discriminator, both of which are generated by adversarial learning. Additionally, since the local alignment does not take full advantages of its transferability information, we improve the local module with the local attention mechanism, taking more attention on more transferable regions. We evaluate our CROPTD on two large- scale high-resolution satellite images located in Peninsular Malaysia. CROPTD improves the detection accuracy by 8.69% in terms of average F1-score compared with the Baseline method (Faster R-CNN) and performs 4.99-2.21% better than other two state-of-the-art domain adaptive object detection approaches. Experimental results demonstrate the great potential of our CROPTD for large-scale, cross-regional oil palm tree detection, guaranteeing a high detection accuracy as well as saving the manual annotation efforts. Our training and validation dataset are available on https://github.com/rs-dl/CROPTD.
更多查看译文
关键词
adaptive object detection approaches,Faster R-CNN,oil palms counting,cross-regional oil palm tree detection method,tree crown detection,high detection accuracy,transferable regions,local attention mechanism,local domain discriminator,CROPTD
AI 理解论文
溯源树
样例
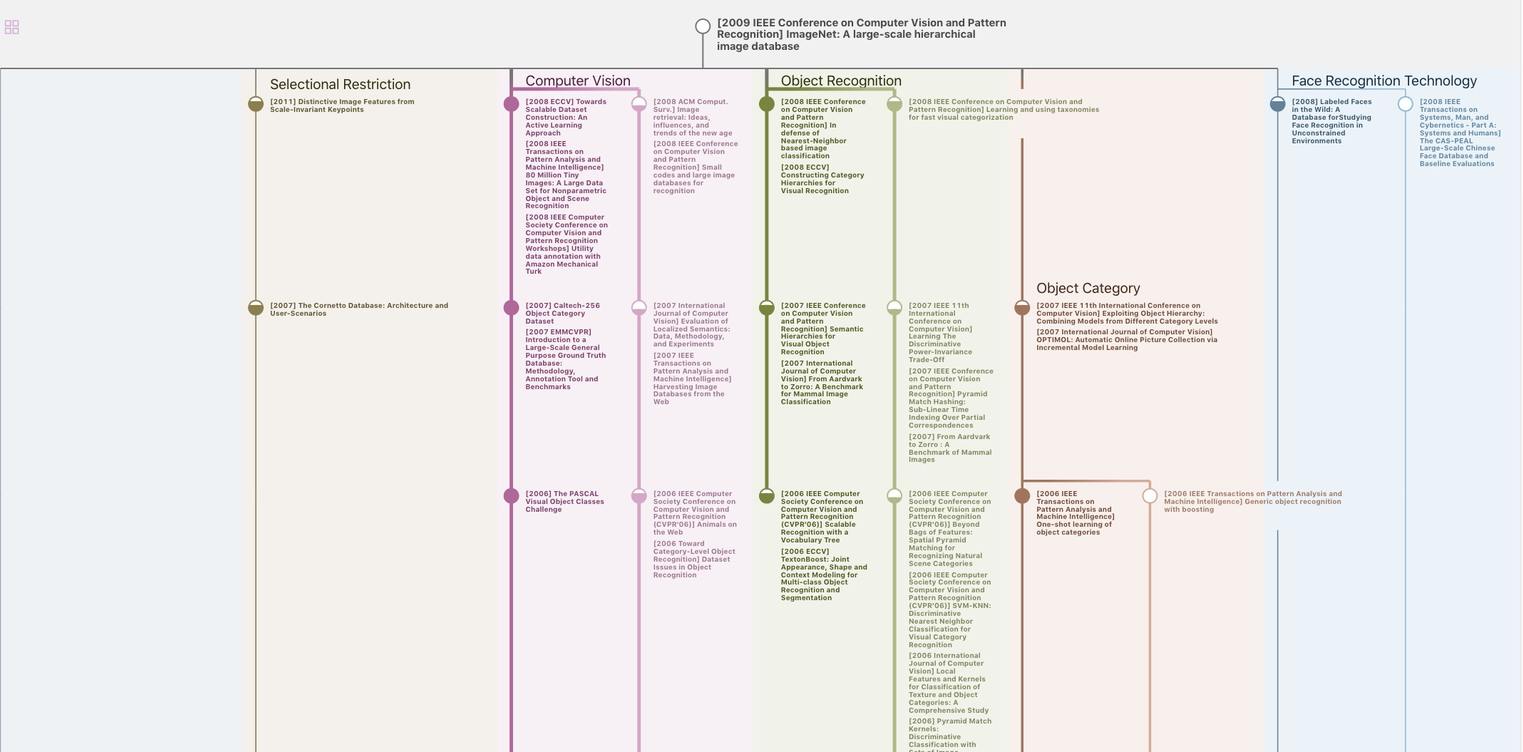
生成溯源树,研究论文发展脉络
Chat Paper
正在生成论文摘要