Experimental Demonstration And Tolerancing Of A Large-Scale Neural Network (165 000 Synapses) Using Phase-Change Memory As The Synaptic Weight Element
IEEE TRANSACTIONS ON ELECTRON DEVICES(2015)
摘要
Using two phase-change memory devices per synapse, a three-layer perceptron network with 164 885 synapses is trained on a subset (5000 examples) of the MNIST database of handwritten digits using a backpropagation variant suitable for nonvolatile memory (NVM) + selector crossbar arrays, obtaining a training (generalization) accuracy of 82.2% (82.9%). Using a neural network simulator matched to the experimental demonstrator, extensive tolerancing is performed with respect to NVM variability, yield, and the stochasticity, linearity, and asymmetry of the NVM-conductance response. We show that a bidirectional NVM with a symmetric, linear conductance response of high dynamic range is capable of delivering the same high classification accuracies on this problem as a conventional, software-based implementation of this same network.
更多查看译文
关键词
Artificial neural networks,Machine learning,Multilayer perceptrons,Nonvolatile memory,Phase change memory
AI 理解论文
溯源树
样例
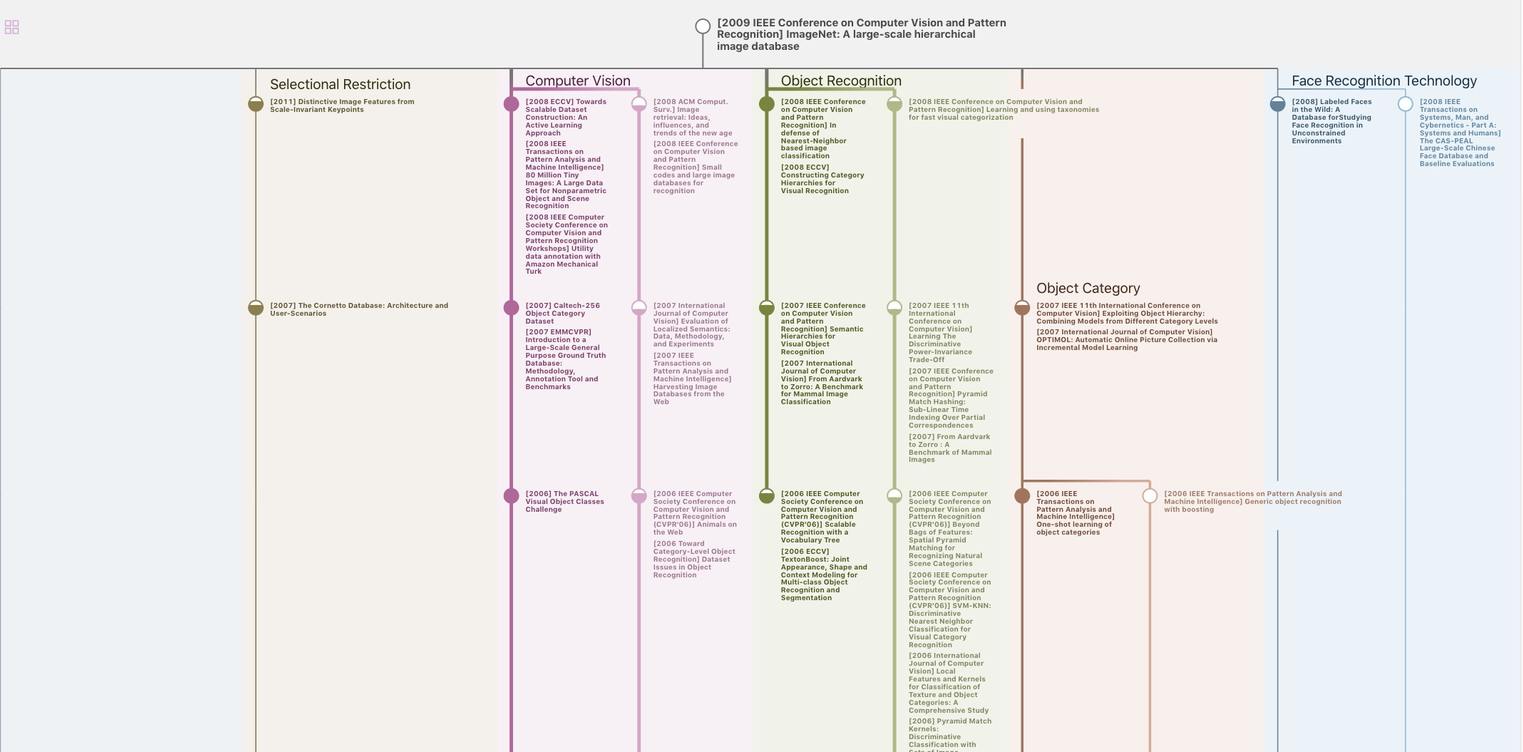
生成溯源树,研究论文发展脉络
Chat Paper
正在生成论文摘要