ParticleMDI: particle Monte Carlo methods for the cluster analysis of multiple datasets with applications to cancer subtype identification
Advances in Data Analysis and Classification(2020)
摘要
We present a novel nonparametric Bayesian approach for performing cluster analysis in a context where observational units have data arising from multiple sources. Our approach uses a particle Gibbs sampler for inference in which cluster allocations are jointly updated using a conditional particle filter within a Gibbs sampler, improving the mixing of the MCMC chain. We develop several approaches to improving the computational performance of our algorithm. These methods can achieve greater than an order-of-magnitude improvement in performance at no cost to accuracy and can be applied more broadly to Bayesian inference for mixture models with a single dataset. We apply our algorithm to the discovery of risk cohorts amongst 243 patients presenting with kidney renal clear cell carcinoma, using samples from the Cancer Genome Atlas, for which there are gene expression, copy number variation, DNA methylation, protein expression and microRNA data. We identify 4 distinct consensus subtypes and show they are prognostic for survival rate ( p < 0.0001 ).
更多查看译文
关键词
Cluster analysis, Mixture models, Bayesian inference, Particle Monte Carlo, 62H30, 62P10, 65C05
AI 理解论文
溯源树
样例
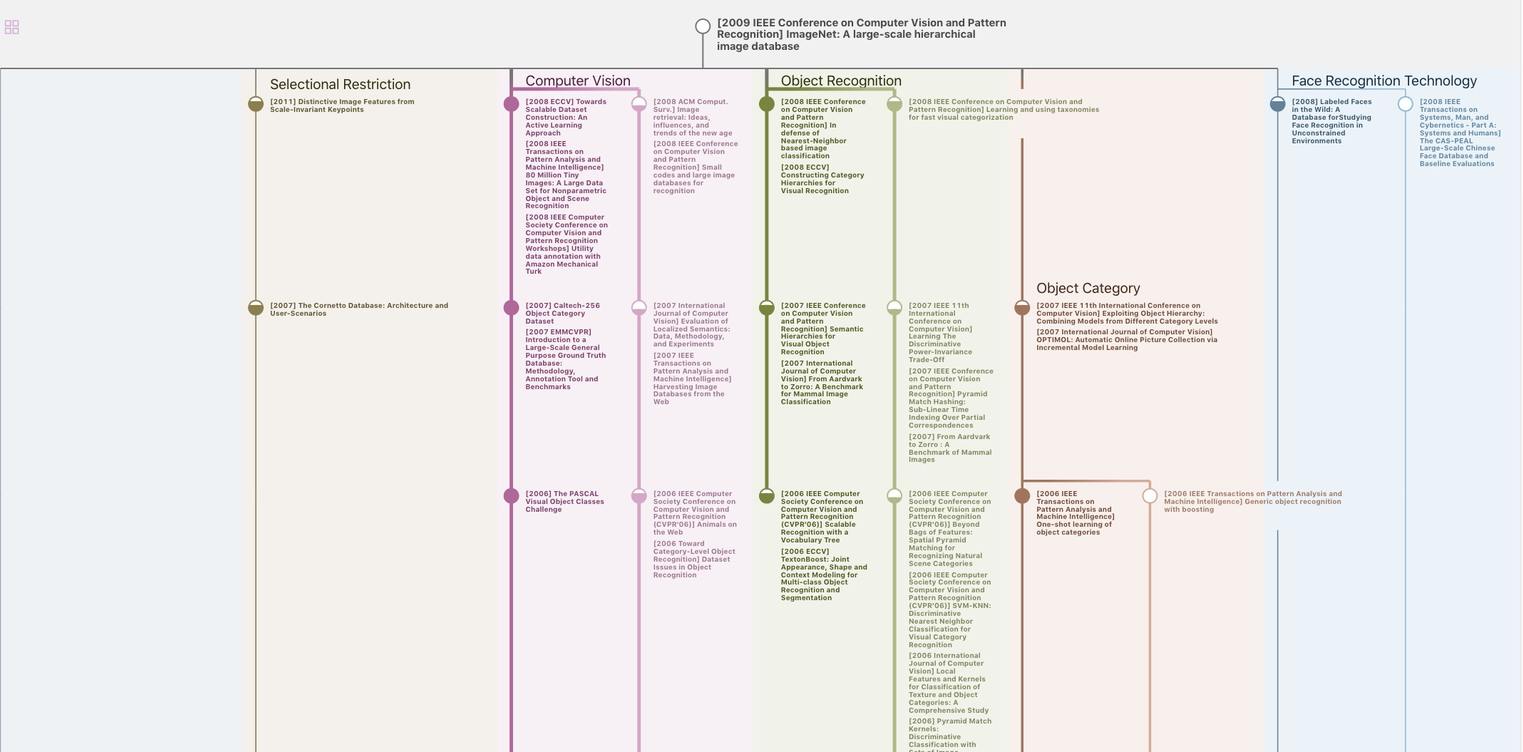
生成溯源树,研究论文发展脉络
Chat Paper
正在生成论文摘要