Robotic Grasping Using Deep Reinforcement Learning
2020 IEEE 16TH INTERNATIONAL CONFERENCE ON AUTOMATION SCIENCE AND ENGINEERING (CASE)(2020)
摘要
In this work, we present a deep reinforcement learning based method to solve the problem of robotic grasping using visio-motor feedback. The use of a deep learning based approach reduces the complexity caused by the use of handdesigned features. Our method uses an off-policy reinforcement learning framework to learn the grasping policy. We use the double deep Q-learning framework along with a novel Grasp-Q-Network to output grasp probabilities used to learn grasps that maximize the pick success. We propose a visual servoing mechanism that uses a multi-view camera setup that observes the scene which contains the objects of interest. We performed experiments using a Baxter Gazebo simulated environment as well as on the actual robot. The results show that our proposed method outperforms the baseline Q-learning framework and increases grasping accuracy by adapting a multi-view model in comparison to a single-view model.
更多查看译文
关键词
deep reinforcement learning
AI 理解论文
溯源树
样例
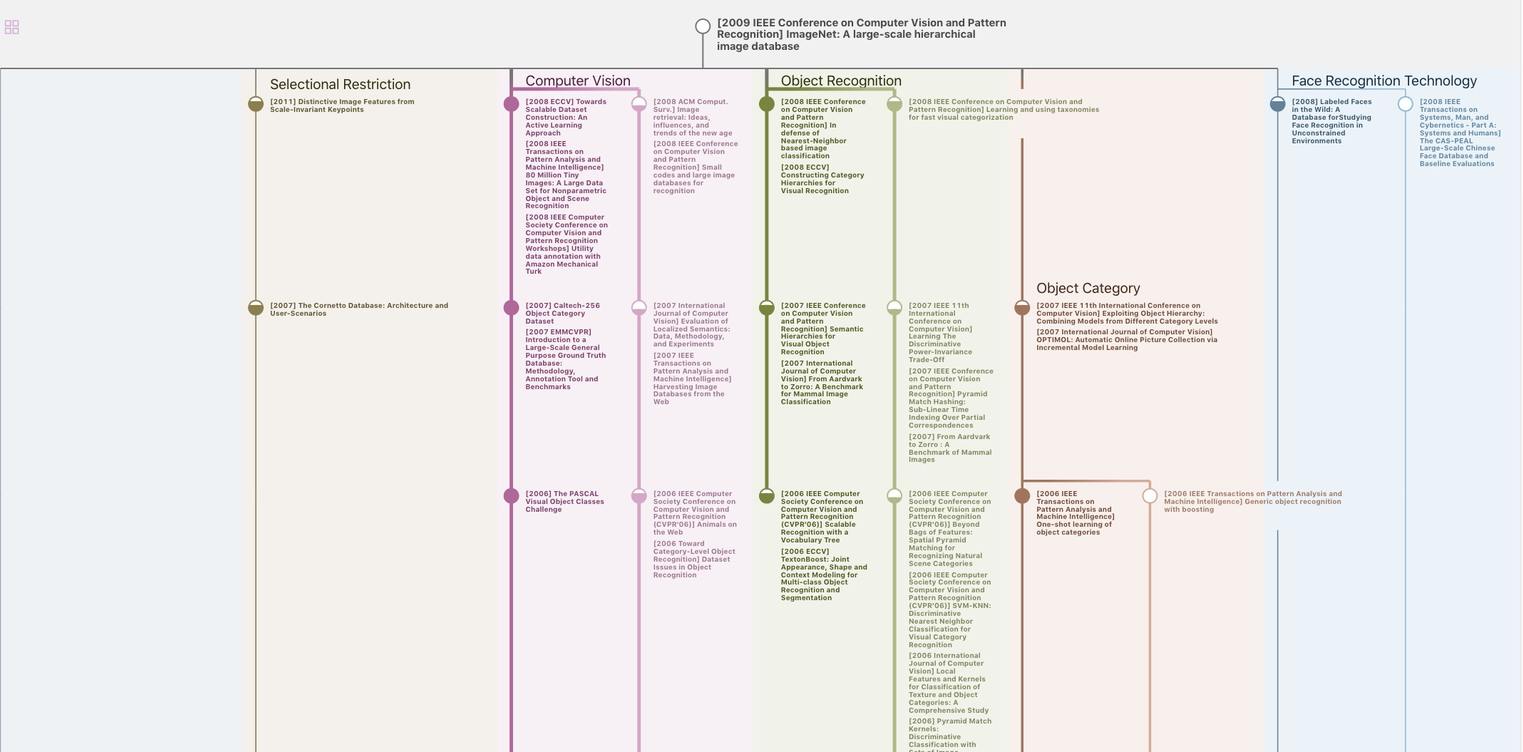
生成溯源树,研究论文发展脉络
Chat Paper
正在生成论文摘要