Hierarchical Graph Matching Networks for Deep Graph Similarity Learning
arxiv(2021)
摘要
While the celebrated graph neural networks yield effective representations for individual nodes of a graph, there has been relatively less success in extending to deep graph similarity learning. Recent work has considered either global-level graph-graph interactions or low-level node-node interactions, ignoring the rich cross-level interactions (e.g., between nodes and a whole graph). In this paper, we propose a Hierarchical Graph Matching Network (HGMN) for computing the graph similarity between any pair of graph-structured objects. Our model jointly learns graph representations and a graph matching metric function for computing graph similarities in an end-to-end fashion. The proposed HGMN model consists of a node-graph matching network for effectively learning cross-level interactions between nodes of a graph and a whole graph, and a siamese graph neural network for learning global-level interactions between two graphs. Our comprehensive experiments demonstrate that HGMN consistently outperforms state-of-the-art graph matching network baselines for both classification and regression tasks.
更多查看译文
关键词
Code similarity,deep learning,graph neural network (GNN),graph similarity
AI 理解论文
溯源树
样例
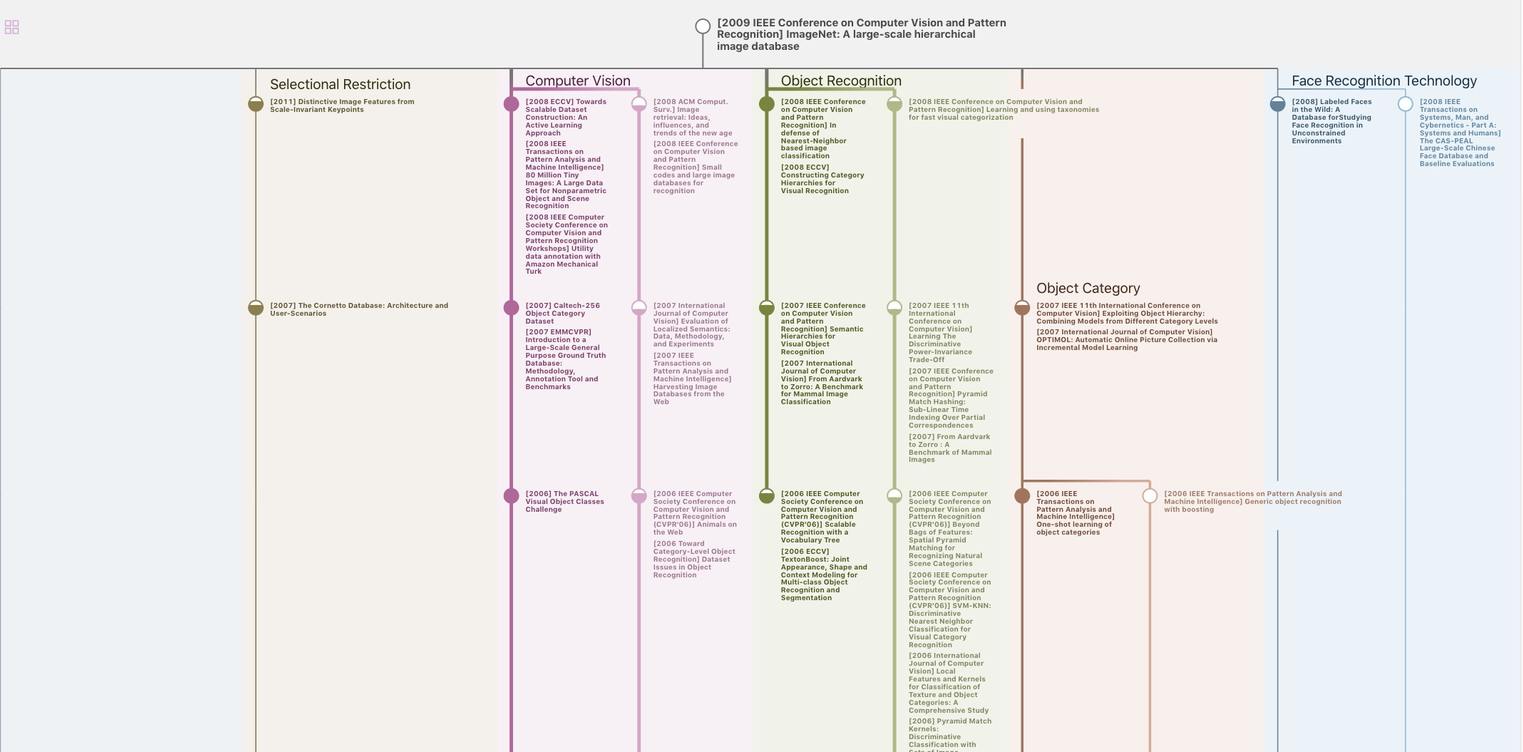
生成溯源树,研究论文发展脉络
Chat Paper
正在生成论文摘要