Multi-view Deep Gaussian Process with a Pre-training Acceleration Technique.
PAKDD (2)(2020)
摘要
Deep Gaussian process (DGP) is one of the popular probabilistic modeling methods, which is powerful and widely used for function approximation and uncertainty estimation. However, the traditional DGP lacks consideration for multi-view cases in which data may come from different sources or be constructed by different types of features. In this paper, we propose a generalized multi-view DGP (MvDGP) to capture the characteristics of different views and model data in different views discriminately. In order to make the proposed model more efficient in training, we introduce a pre-training network in MvDGP and incorporate stochastic variational inference for fine-tuning. Experimental results on real-world data sets demonstrate that pre-trained MvDGP outperforms the state-of-the-art DGP models and deep neural networks, achieving higher computational efficiency than other DGP models.
更多查看译文
关键词
Deep Gaussian process, Multi-view learning, Variational inference, Stochastic optimization, Pre-training technique
AI 理解论文
溯源树
样例
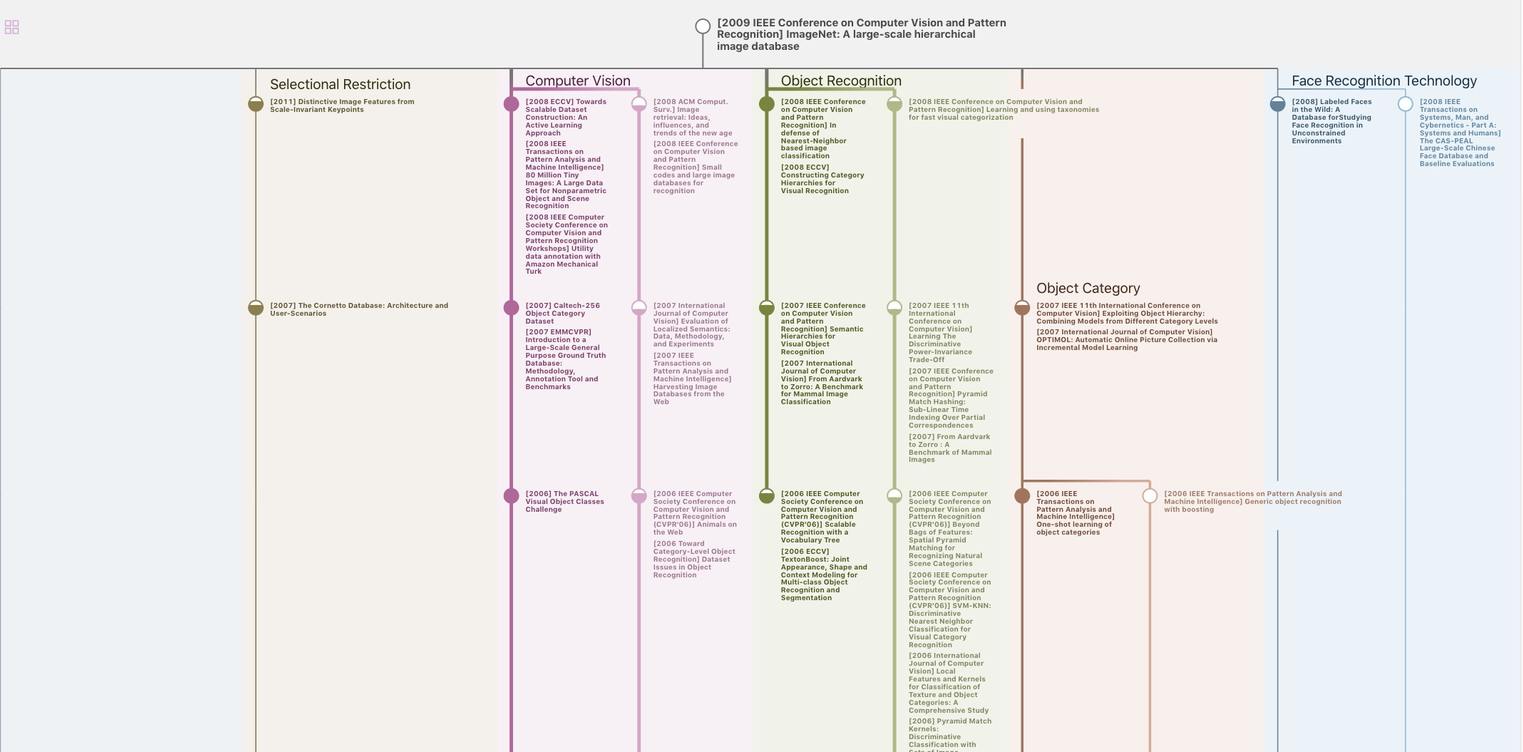
生成溯源树,研究论文发展脉络
Chat Paper
正在生成论文摘要