Prediction of the Number of New Cases of 2019 Novel Coronavirus (COVID-19) Using a Social Media Search Index
SSRN Electronic Journal(2020)
摘要
Purpose: Predicting the number of novel Coronavirus Disease 2019 (COVID-19) new-suspected or confirmed cases is crucial in the prevention and control of the COVID-19 outbreak.Methods: Social media search indexes (SMSI) for dry cough, fever, chest distress, coronavirus, and pneumonia were collected from December 31, 2019, to February 9, 2020. The new-suspected cases of COVID-19 data were collected from January 20, 2020, to February 9, 2020. We used the lagged series of SMSI to predict new-suspected COVID-19 case numbers during this period. To avoid overfitting, five methods, namely subset selection, forward selection, lasso regression, ridge regression, and elastic net, were used to estimate coefficients. We selected the optimal method to predict new-suspected COVID-19 case numbers over the subsequent 20 days. We further validated the optimal method for new-confirmed cases of COVID-19 from December 31, 2019, to February 17, 2020.Results: The new-suspected COVID-19 case numbers were significantly correlated with the lagged series of SMSI. SIMI could be earlier detected 6–9 days than new-suspected cases of COVID-19. The optimal method was subset select, which had the lowest estimation error and a moderate number of predictors. The subset selection method was also significantly correlated with the new-confirmed COVID-19 cases after validation. SMSI findings on lag day 10 were significantly correlated with new-confirmed COVID-19 cases.Conclusions: SMSI could be a significant predictor of the number of COVID-19 infections.Funding Statement: Lo-Hsu Medical Foundation, Lotung Poh-Ai Hospital, supports SzuYuan Wu’s work (Funding Number: 10908 and 10909). Lei Qin's work is supported by University of International Business and Economics Huiyuan outstanding young scholars research funding (17YQ15), "the Fundamental Research Funds for the central Universities" in UIBE (CXTD10-10). Ben-Chang Shia’s work was also supported by an institutional grant from Taipei Medical University (Taipei, Taiwan) for New Faculty Research (TMU103-AE1-B22).Declaration of Interests: The authors have no potential conflicts of interest to declare.
更多查看译文
AI 理解论文
溯源树
样例
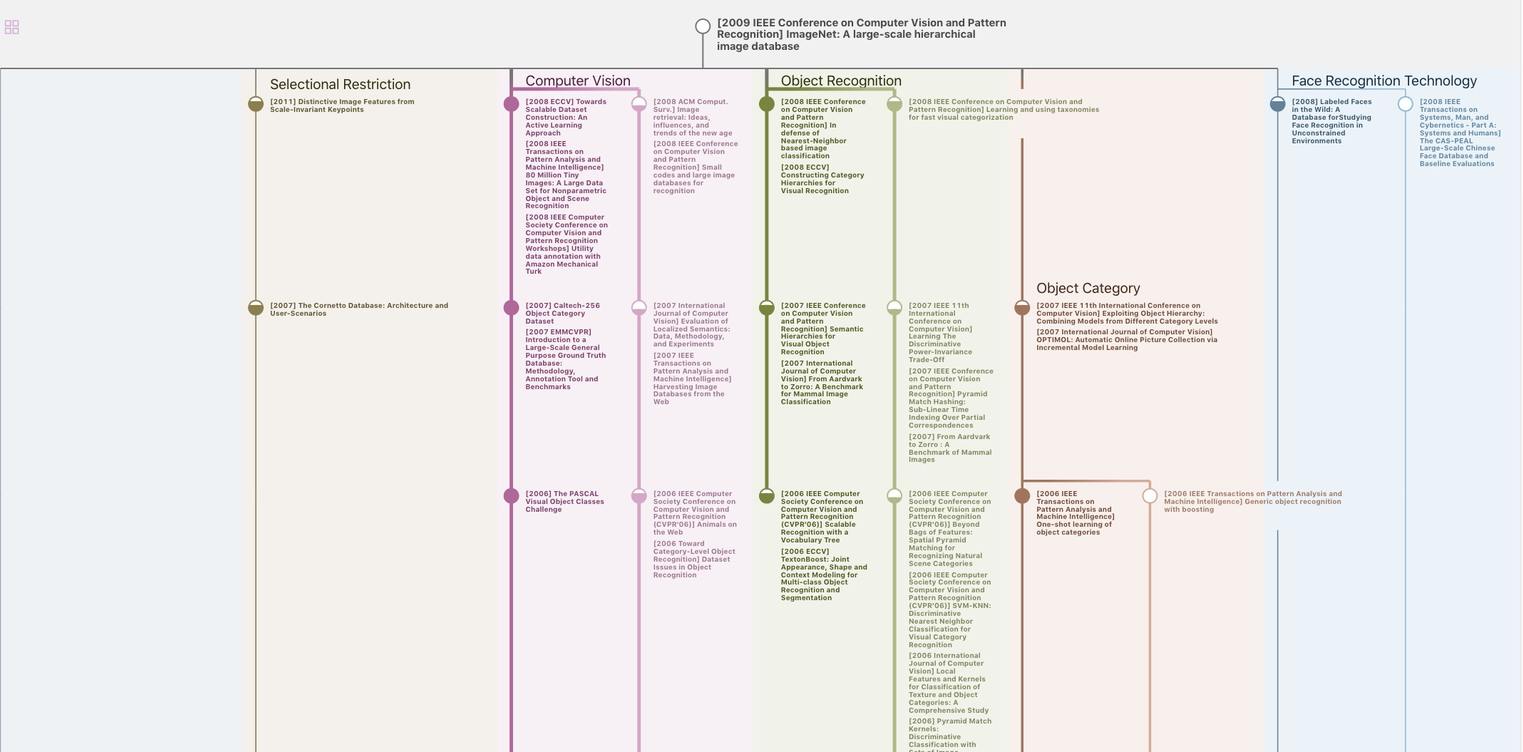
生成溯源树,研究论文发展脉络
Chat Paper
正在生成论文摘要